An adversarial non-volume preserving flow model with Boltzmann priors
International Journal of Machine Learning and Cybernetics(2019)
摘要
Flow-based generative models (flow models) are conceptually attractive due to tractability of the exact log-likelihood and the exact latent-variable inference. In order to generate sharper images and extend the Gaussian prior of Flow models to other discrete forms, we propose an adversarial non-volume preserving flow model with Boltzmann priors (ANVP) for modeling complex high-dimensional densities. In order to generate sharper images, an ANVP model introduces an adversarial regularizer into the loss function to penalize the condition that it places a high probability in regions where the training data distribution has a low density. Moreover, we show that the Gaussian prior can be extended to other forms such as the Boltzmann prior in the proposed ANVP model, and we use multi-scale transformations and Boltzmann priors to model the data distribution. The experiments show that proposed model is effective in image generation task.
更多查看译文
关键词
Flow models, Boltzmann machines, Generative adversarial networks, Deep generative model
AI 理解论文
溯源树
样例
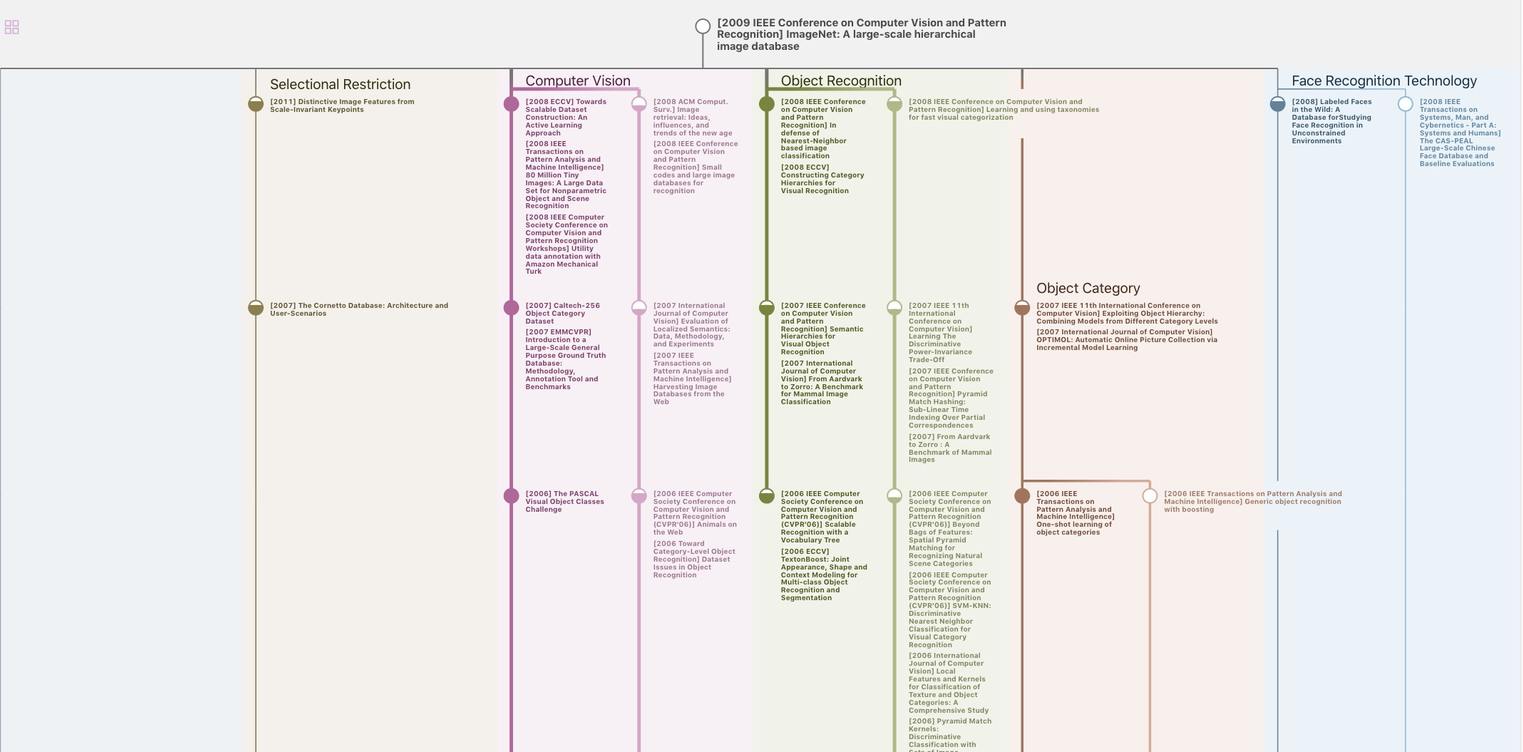
生成溯源树,研究论文发展脉络
Chat Paper
正在生成论文摘要