spECK - accelerating GPU sparse matrix-matrix multiplication through lightweight analysis.
PPoPP '20: 25th ACM SIGPLAN Symposium on Principles and Practice of Parallel Programming San Diego California February, 2020(2020)
摘要
Sparse general matrix-matrix multiplication on GPUs is challenging due to the varying sparsity patterns of sparse matrices. Existing solutions achieve good performance for certain types of matrices, but fail to accelerate all kinds of matrices in the same manner. Our approach combines multiple strategies with dynamic parameter selection to dynamically choose and tune the best fitting algorithm for each row of the matrix. This choice is supported by a lightweight, multi-level matrix analysis, which carefully balances analysis cost and expected performance gains. Our evaluation on thousands of matrices with various characteristics shows that we outperform all currently available solutions in 79% over all matrices with >15k products and that we achieve the second best performance in 15%. For these matrices, our solution is on average 83% faster than the second best approach and up to 25X faster than other state-of-the-art GPU implementations. Using our approach, applications can expect great performance independent of the matrices they work on.
更多查看译文
关键词
SpGEMM, Sparse Matrix, GPU, Analysis
AI 理解论文
溯源树
样例
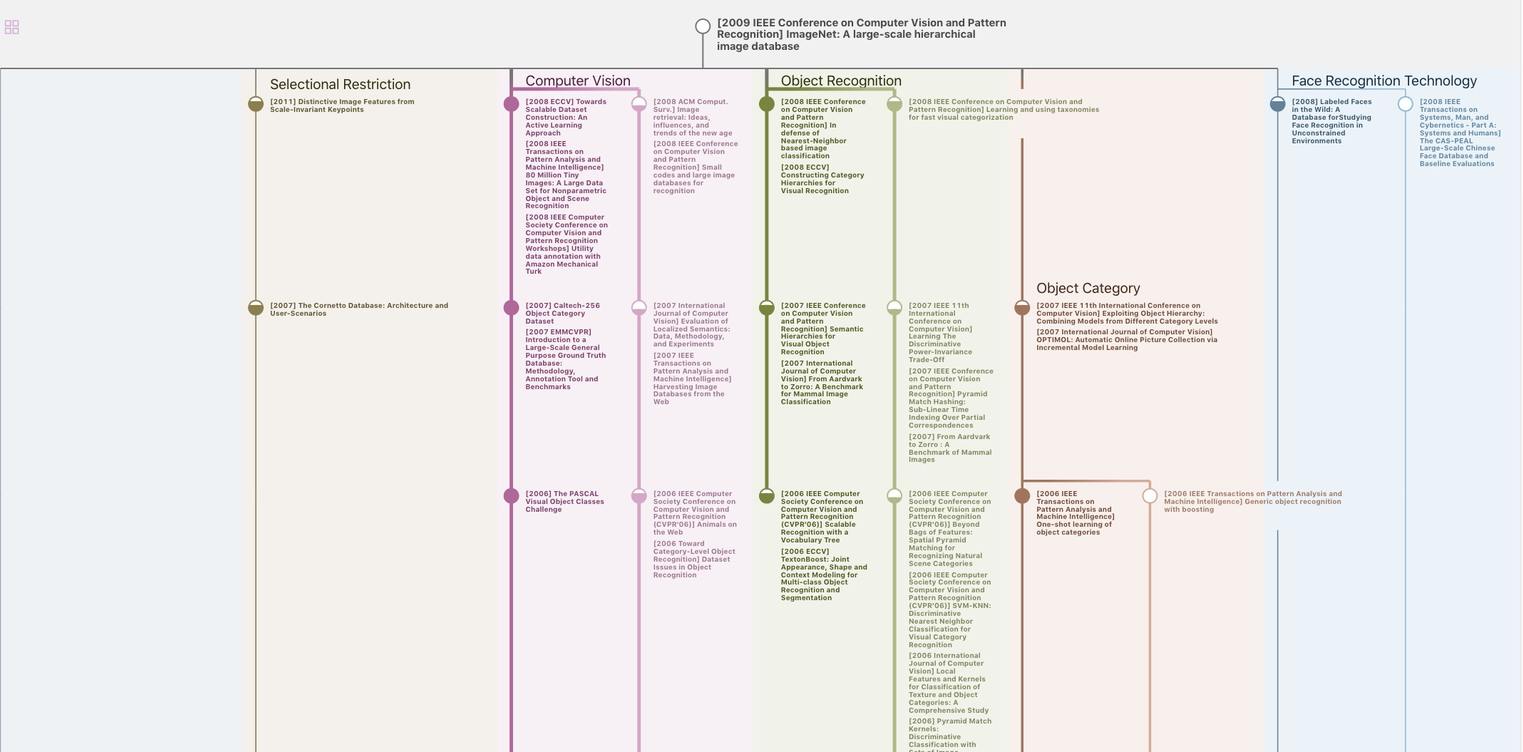
生成溯源树,研究论文发展脉络
Chat Paper
正在生成论文摘要