Learning models of quantum systems from experiments
NATURE PHYSICS(2021)
摘要
As Hamiltonian models underpin the study and analysis of physical and chemical processes, it is crucial that they are faithful to the system they represent. However, formulating and testing candidate Hamiltonians for quantum systems from experimental data is difficult, because one cannot directly observe which interactions are present. Here we propose and demonstrate an automated protocol to overcome this challenge by designing an agent that exploits unsupervised machine learning. We first show the capabilities of our approach to infer the correct Hamiltonian when studying a nitrogen-vacancy centre set-up. In preliminary simulations, the exact model is known and is correctly inferred with success rates up to 59%. When using experimental data, 74% of protocol instances retrieve models that are deemed plausible. Simulated multi-spin systems, characterized by a space of 10 10 possible models, are also investigated by incorporating a genetic algorithm in our protocol, which identifies the target model in 85% of instances. The development of automated agents, capable of formulating and testing modelling hypotheses from limited prior assumptions, represents a fundamental step towards the characterization of large quantum systems.
更多查看译文
关键词
Computational science,Quantum information,Quantum physics,Quantum simulation,Physics,general,Theoretical,Mathematical and Computational Physics,Classical and Continuum Physics,Atomic,Molecular,Optical and Plasma Physics,Condensed Matter Physics,Complex Systems
AI 理解论文
溯源树
样例
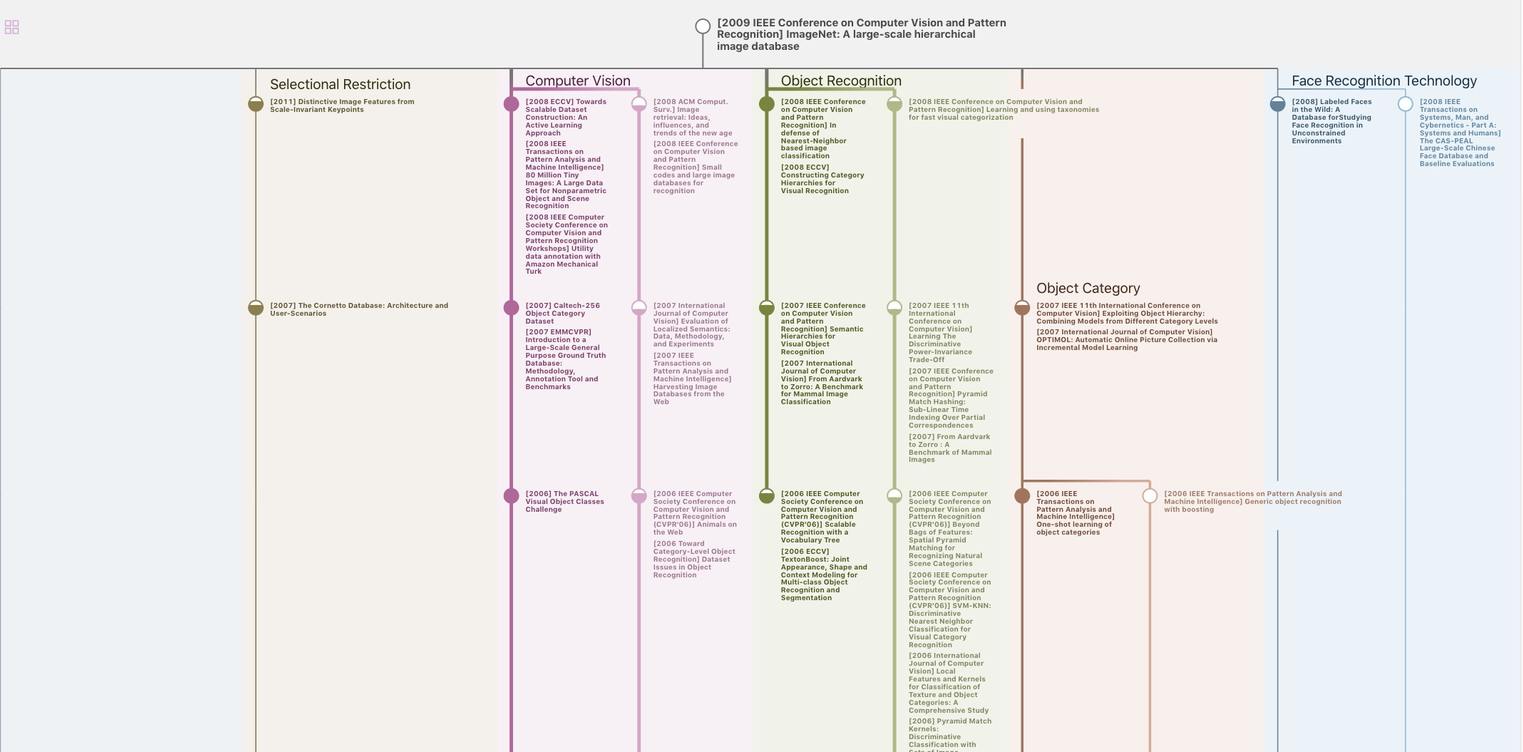
生成溯源树,研究论文发展脉络
Chat Paper
正在生成论文摘要