Likelihood Based Learning Rule for Temporal Coding In Recurrent Spiking Neural Networks
arxiv(2020)
摘要
Recurrent spiking neural networks (RSNN) in the brain learn to perform a wide range of perceptual, cognitive and motor tasks very efficiently in terms of time and energy consumption. This is due to the optimality of coding and learning schemes, which have yet to be unveiled. The formulation of biologically inspired networks capable to perform complex computations can mediate a synergetic interaction between Machine Learning and Neuroscience bringing to mutual benefits and helping to improve our understanding of biological and artificial intelligence. Even though several models have been proposed, it remains a challenging task to design RSNNs which use biologically plausible mechanisms. We propose a general probabilistic framework which relies on the principle of maximizing the likelihood for the network to solve the task. This principle permits to analytically work out an explicit and completely local plasticity rule supporting the efficient solution of several tasks. We show that the learning algorithm can be achieved in a very few iterations, and that the online approximation of the likelihood maximization is extremely beneficial to fast learning. Our model is very general and it can be applied to a wide variety of network architectures and types of biological neurons. The derived plasticity learning rule is specific to each neuron model producing a theoretical prediction which can be verified experimentally.
更多查看译文
关键词
temporal coding,likelihood based learning rule,neural networks,recurrent
AI 理解论文
溯源树
样例
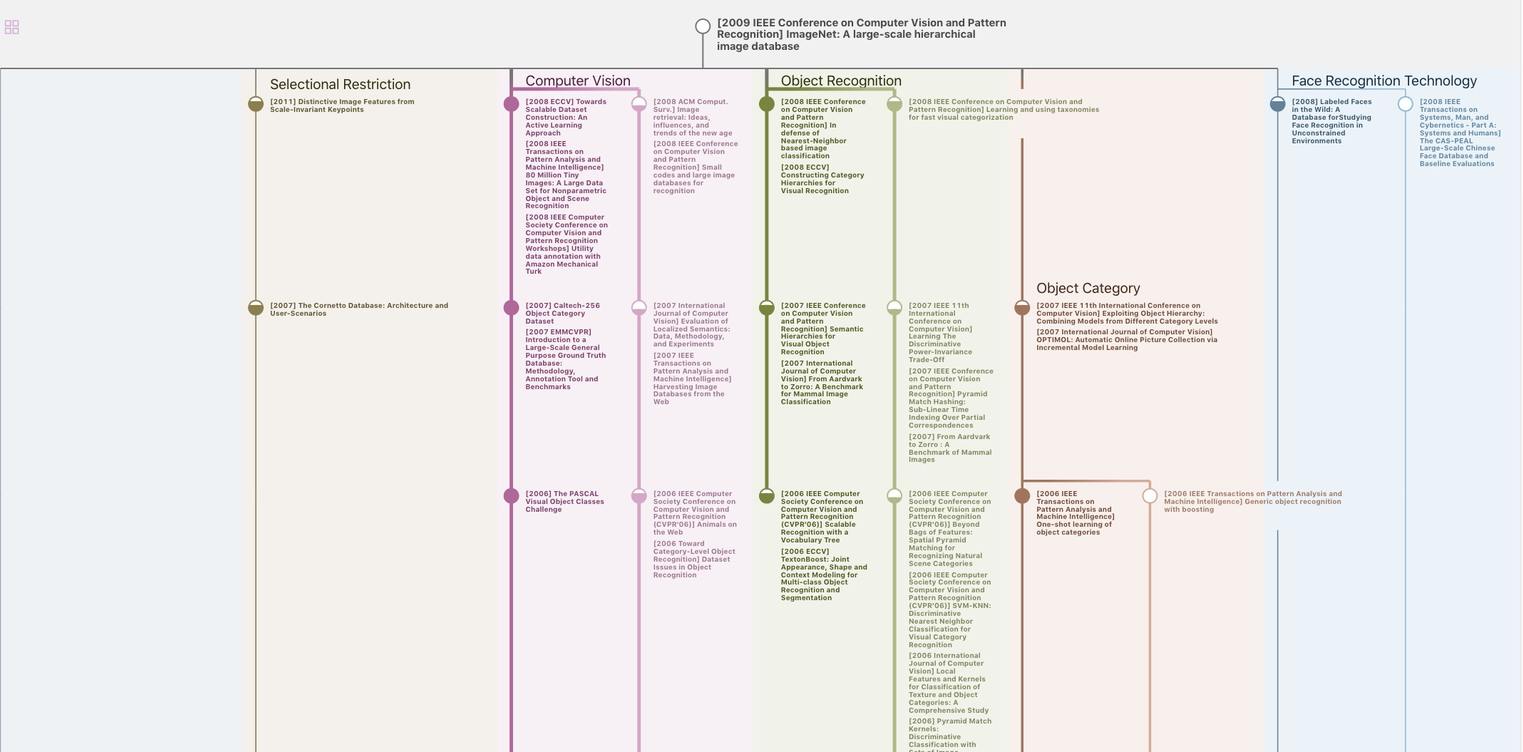
生成溯源树,研究论文发展脉络
Chat Paper
正在生成论文摘要