Towards a combinatorial characterization of bounded memory learning
arxiv(2020)
摘要
Combinatorial dimensions play an important role in the theory of machine learning. For example, VC dimension characterizes PAC learning, SQ dimension characterizes weak learning with statistical queries, and Littlestone dimension characterizes online learning. In this paper we aim to develop combinatorial dimensions that characterize bounded memory learning. We propose a candidate solution for the case of realizable strong learning under a known distribution, based on the SQ dimension of neighboring distributions. We prove both upper and lower bounds for our candidate solution, that match in some regime of parameters. In this parameter regime there is an equivalence between bounded memory and SQ learning. We conjecture that our characterization holds in a much wider regime of parameters.
更多查看译文
关键词
combinatorial characterization,memory,learning
AI 理解论文
溯源树
样例
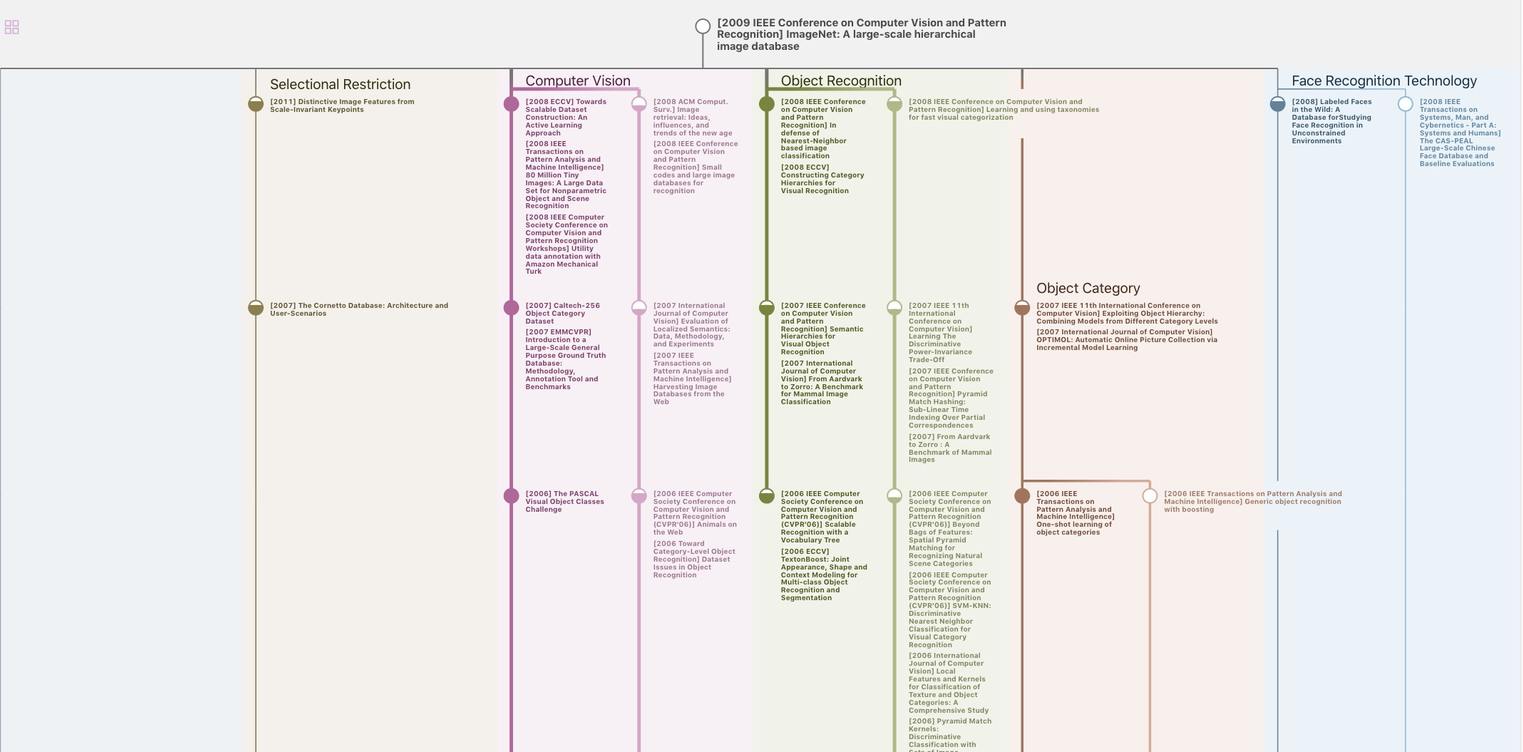
生成溯源树,研究论文发展脉络
Chat Paper
正在生成论文摘要