Enhanced Semantic Features via Attention for Real-Time Visual Tracking
2019 IEEE Visual Communications and Image Processing (VCIP)(2019)
摘要
The key to balance the tracking accuracy and speed for object tracking algorithms is to learn powerful features via offline training in a lightweight tracking framework. With the development of attention mechanisms, it's facile to apply attention to enhance the features without modifying the basic structure of the network. In this paper, a novel combination of different attention modules is implemented into a siamese-based tracker and boosts the tracking performance with little computational burden. In particular, by applying non-local self-attention and dual pooling channel attention, the extracted features tend to be more discriminative and adaptive due to the offline learning with tracking targets of different classes. Meanwhile, an Index-Difference-weight boosts the performance and reduces overfitting when full occlusion occurs. Our experimental results on OTB2013 and OTB2015 show that the tracker using the proposal to implement the attention modules can achieve state-of-the-art performance with a speed of 49 frames per second.
更多查看译文
关键词
Object tracking,Attention modules,Siamese Network,Semantic feature,Real-time Tracker
AI 理解论文
溯源树
样例
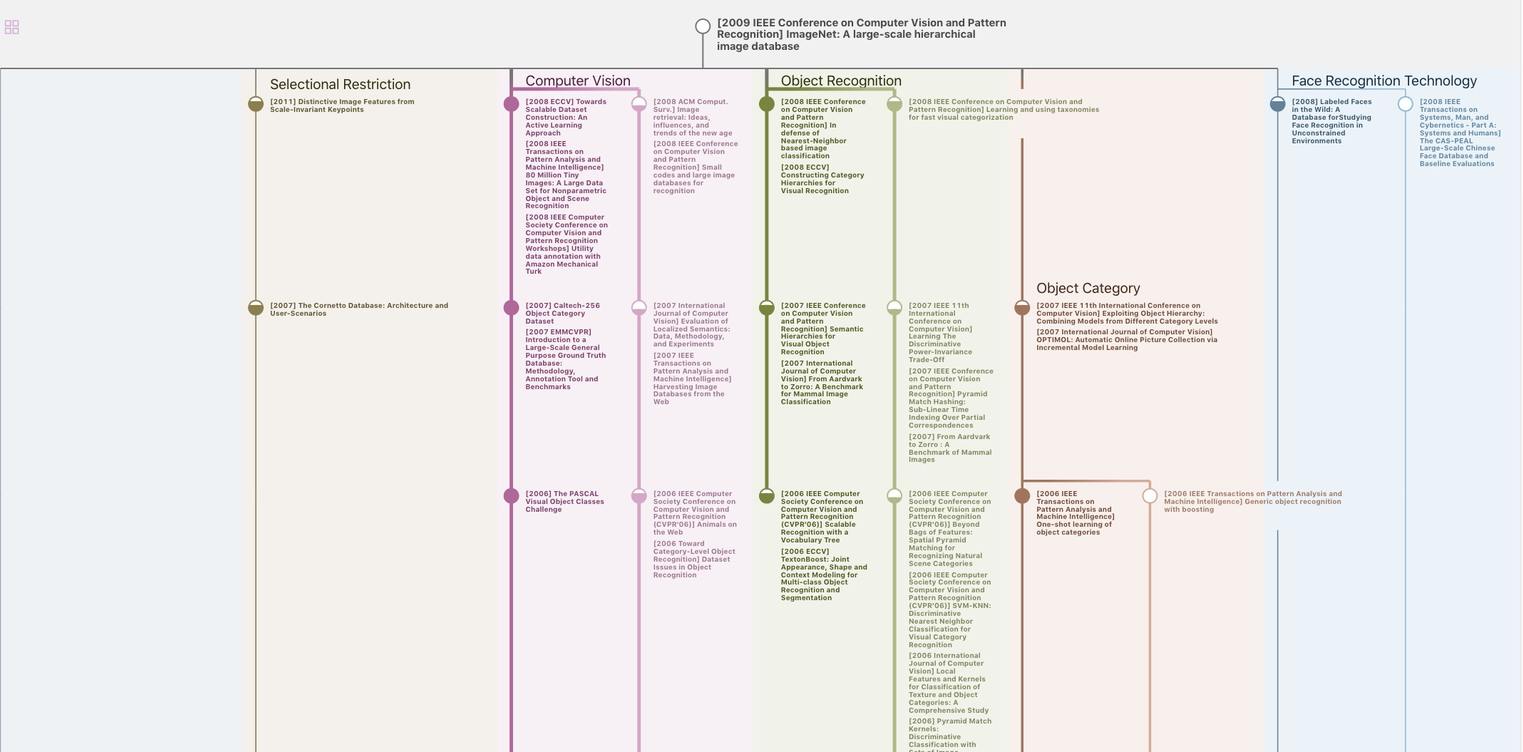
生成溯源树,研究论文发展脉络
Chat Paper
正在生成论文摘要