Modeling Polarized Reflectance of Natural Land Surfaces Using Generalized Regression Neural Networks.
REMOTE SENSING(2020)
摘要
Retrieval of complete aerosol properties over land through remote sensing requires accurate information about the polarization characteristics of natural land surfaces. In this paper, a new bidirectional polarization distribution function (BPDF) is proposed, using the generalized regression neural network (GRNN). This GRNN-based BPDF model builds a quite accurate nonlinear relationship between polarized reflectance and four input parameters, i.e., Fresnel factor, scattering angle, red, and near-infrared reflectances. It learns fast because only a smoothing parameter needs to be adjusted. The GRNN-based model is compared to six widely used BPDF models (i.e., Nadal-Breon, Maignan, Waquet, Litivinov, Diner, and Xie-Cheng models), using the Polarization and Directionality of the Earth's Reflectance (POLDER) measurements. Experiments suggest that the GRNN-based BPDF model is more accurate than these models. Compared with the best current models, the averaged root-mean-square error (RMSE) from the GRNN-based BPDF model can be reduced by 13.4% by using data collected during the whole year and is lower for 97.4% cases with data collected during every month. Moreover, compared to the widely used BPDF models, the GRNN-based BPDF model provides better performance when the scattering angle is small, and it is the first model that is able to reproduce negative polarized reflectance. The GRNN-based BPDF model is thus useful for the remote sensing of complete aerosol properties over land.
更多查看译文
关键词
bidirectional polarization distribution function,natural land surfaces,generalized regression neural network,POLDER
AI 理解论文
溯源树
样例
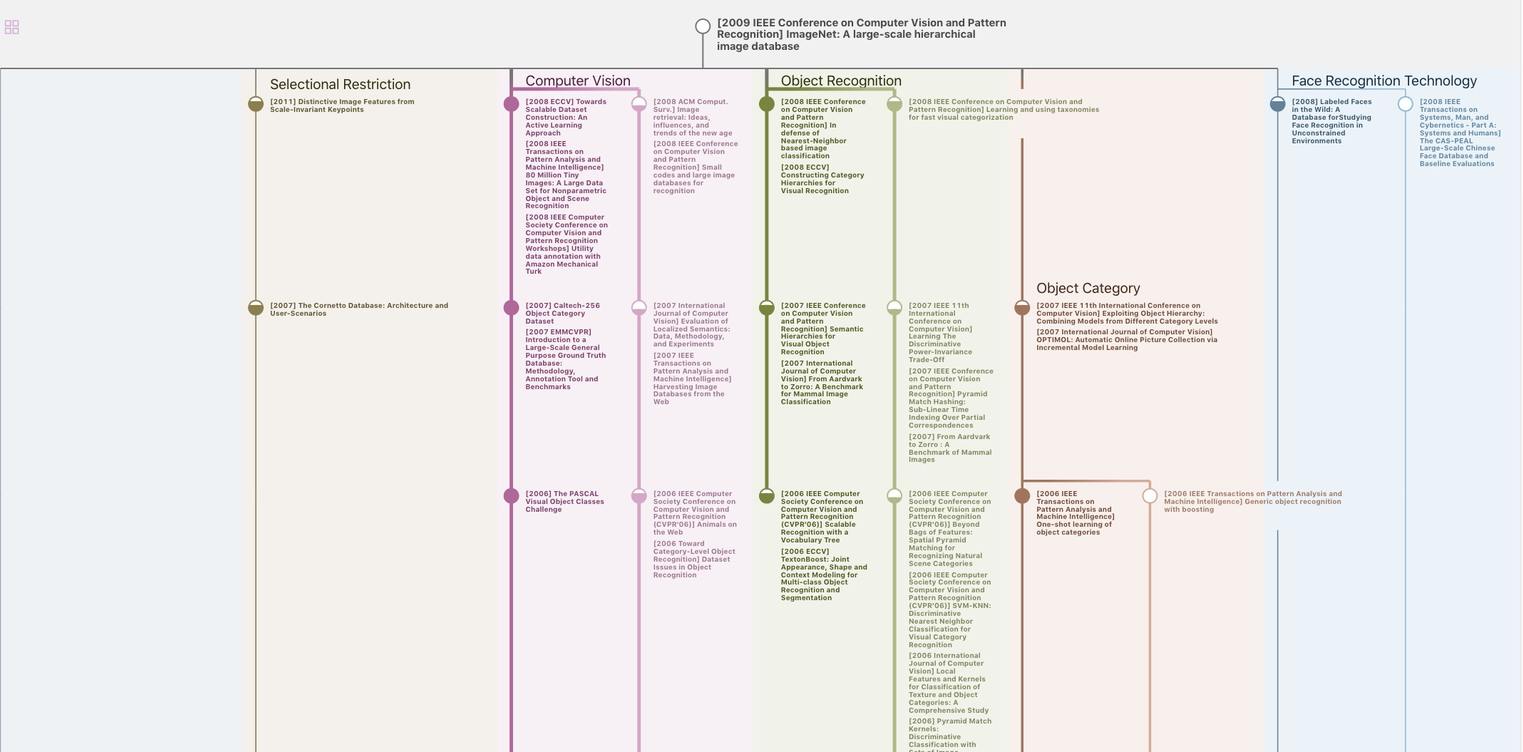
生成溯源树,研究论文发展脉络
Chat Paper
正在生成论文摘要