Prediction of Liver Respiratory Motion Based on Machine Learning.
ROBIO(2019)
摘要
Hepatic respiratory movement has always been an important factor that affects the accuracy of liver interventional therapy. To improve the prediction accuracy of image-guided therapy, we proposed a liver breath prediction model that combines machine learning, surface point set sparse registration, and internal and external breath amplitude correlation. We used surface sparse point set registration to calculate the displacement vector field of the liver surface and the displacement vector field of a specified region of the abdominal surface. Using correlation analysis of the internal and external respiratory amplitudes, we selected the liver displacement vector field that is closest to the input respiratory signal as the optimal training data. A patient-specific model that combines local vector field optimization with abdominal surface similarity optimization was constructed by combining the liver surface and the abdominal surface after segmentation, and accurate motion prediction was realized based on principal component analysis (PCA). In an experiment on 7 patients, we adopted two experimental verification methods: (1) only one data collection stage and one cross-validation stage were used, and (2) the experimental data that were collected in the first stage were used as the training data set, and the experimental data that were collected in the second stage were used as the test data set. The prediction errors of the two methods were 0.35 ± 0.08 mm and 0.96 ± 0.40 mm, respectively. In this experiment, we combined surface sparse point set registration with an internal and external breath amplitude correlation method, which substantially improved the runtime and accuracy of the experiment compared with the traditional PCA method.
更多查看译文
关键词
respiratory motion,liver,prediction,correlation analysis
AI 理解论文
溯源树
样例
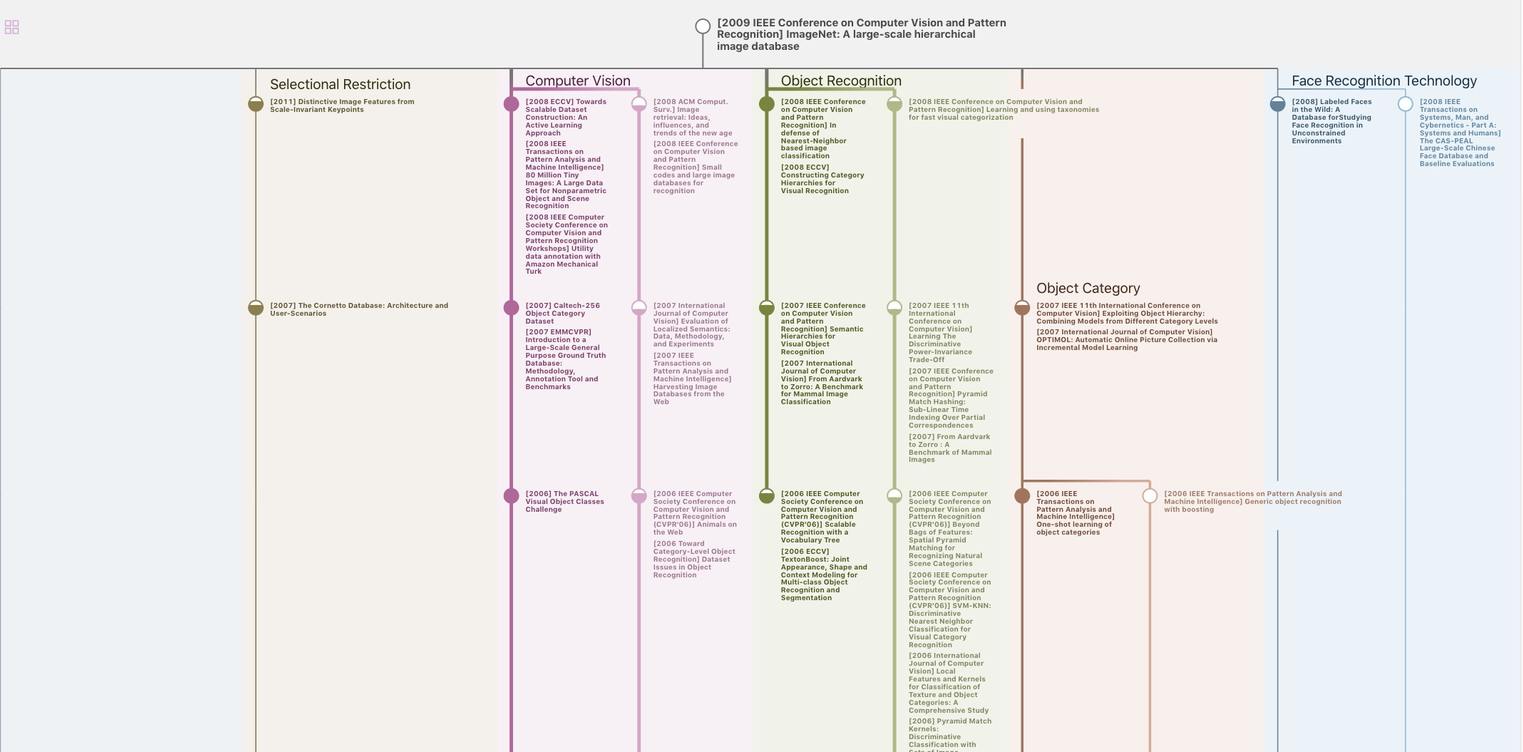
生成溯源树,研究论文发展脉络
Chat Paper
正在生成论文摘要