6D Object Pose Regression via Supervised Learning on Point Clouds
ICRA(2020)
摘要
This paper addresses the task of estimating the 6 degrees of freedom pose of a known 3D object from depth information represented by a point cloud. Deep features learned by convolutional neural networks from color information have been the dominant features to be used for inferring object poses, while depth information receives much less attention. However, depth information contains rich geometric information of the object shape, which is important for inferring the object pose. We use depth information represented by point clouds as the input to both deep networks and geometry-based pose refinement and use separate networks for rotation and translation regression. We argue that the axis-angle representation is a suitable rotation representation for deep learning, and use a geodesic loss function for rotation regression. Ablation studies show that these design choices outperform alternatives such as the quaternion representation and L2 loss, or regressing translation and rotation with the same network. Our simple yet effective approach clearly outperforms state-of-the-art methods on the YCB-video dataset.
更多查看译文
关键词
convolutional neural networks,color information,translation regression,deep learning,rotation regression,6D object pose regression,supervised learning,geometry-based pose refinement,axis-angle representation,geodesic loss function,quaternion representation,YCB-video dataset
AI 理解论文
溯源树
样例
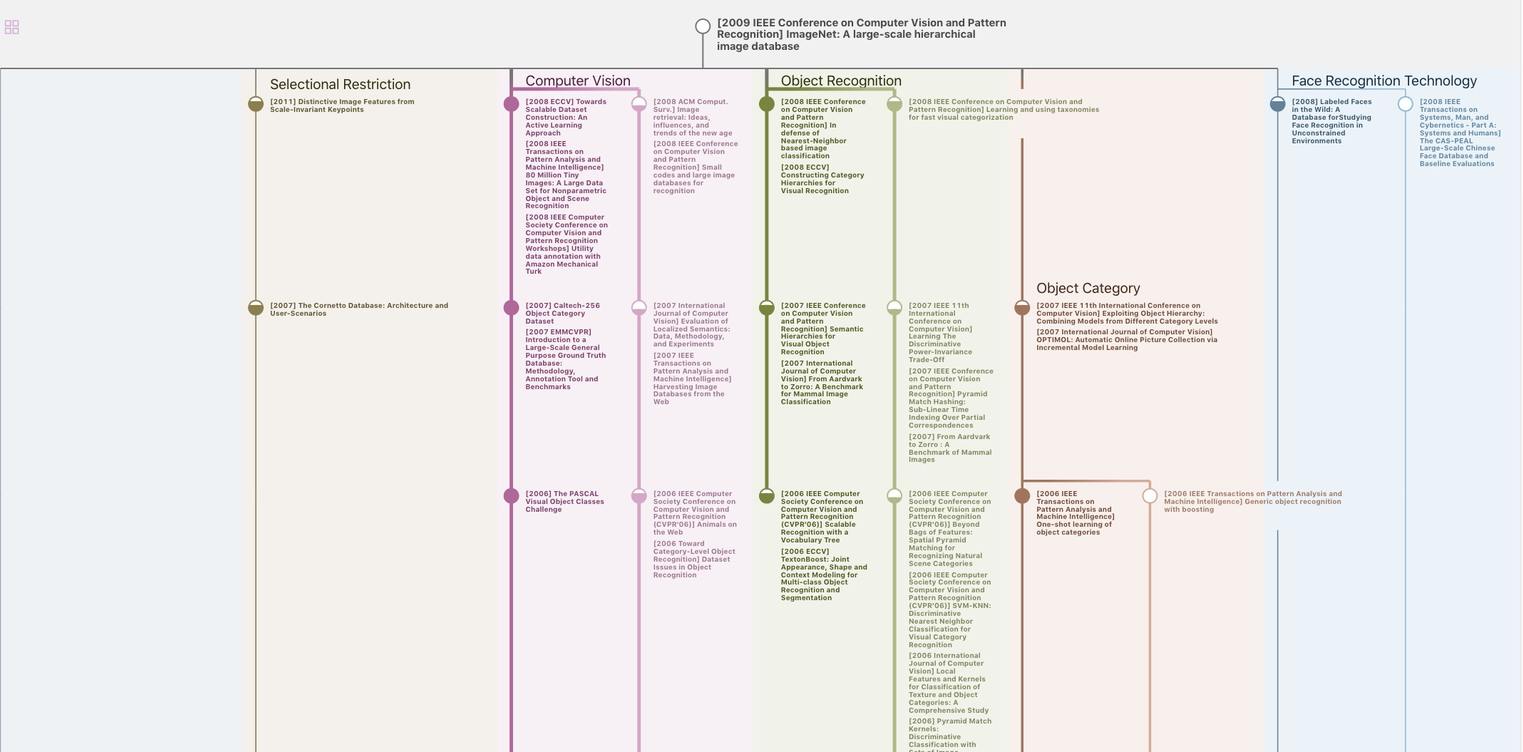
生成溯源树,研究论文发展脉络
Chat Paper
正在生成论文摘要