Improving The Assessment Of Measurement Invariance: Using Regularization To Select Anchor Items And Identify Differential Item Functioning
PSYCHOLOGICAL METHODS(2020)
摘要
A common challenge in the behavioral sciences is evaluating measurement invariance, or whether the measurement properties of a scale are consistent for individuals from different groups. Measurement invariance fails when differential item functioning (DIF) exists, that is, when item responses relate to the latent variable differently across groups. To identify DIF in a scale, many data-driven procedures iteratively test for DIF one item at a time while assuming other items have no DIF. The DIF-free items are used to anchor the scale of the latent variable across groups, identifying the model. A major drawback to these iterative testing procedures is that they can fail to select the correct anchor items and identify true DIF, particularly when DIF is present in many items. We propose an alternative method for selecting anchors and identifying DIF. Namely, we use regularization, a machine learning technique that imposes a penalty function during estimation to remove parameters that have little impact on the fit of the model. We focus specifically here on a lasso penalty for group differences in the item parameters within the two-parameter logistic item response theory model. We compare lasso regularization with the more commonly used likelihood ratio test method in a 2-group DIF analysis. Simulation and empirical results show that when large amounts of DIF are present and sample sizes are large, lasso regularization has far better control of Type I error than the likelihood ratio test method with little decrement in power. This provides strong evidence that lasso regularization is a promising alternative for testing DIF and selecting anchors.Translational AbstractMeasurement in the psychological sciences is difficult in large part because two individuals with identical values on a construct (e.g., depression) may appear unequal when measured. This can happen when an item (e.g., cries easily) is not only tapping into that construct but also into some other background characteristic of the individual-for instance, their sex. This is formally referred to as differential item functioning (DIF). If undetected and unaddressed, DIF can distort inferences about individual and group differences. There are many procedures for statistically detecting DIF, most of which are data-driven and use multiple statistical tests to determine where DIF occurs in a scale. Unfortunately, these procedures make assumptions about other untested items that are unlikely to be true. Specifically, when testing for DIF in one item, one or more other items must be assumed to have no DIF. This is paradoxical, in that the same item is assumed to have DIF in one test but assumed not to have DIF in all other tests. We propose a machine learning approach known as lasso regularization as an alternative. Lasso regularization considers DIF in all items simultaneously, rather than one item at a time, and uses a penalized estimation approach to identify items with and without DIF rather than inference tests with dubious assumptions. Computer simulations and a real data validation study show that lasso regularization performs increasingly better than a commonly used traditional method of DIF detection (the likelihood ratio test approach) as the number of items with DIF and sample size increase.
更多查看译文
关键词
differential item functioning, measurement invariance, item response theory, lasso regularization, likelihood ratio test
AI 理解论文
溯源树
样例
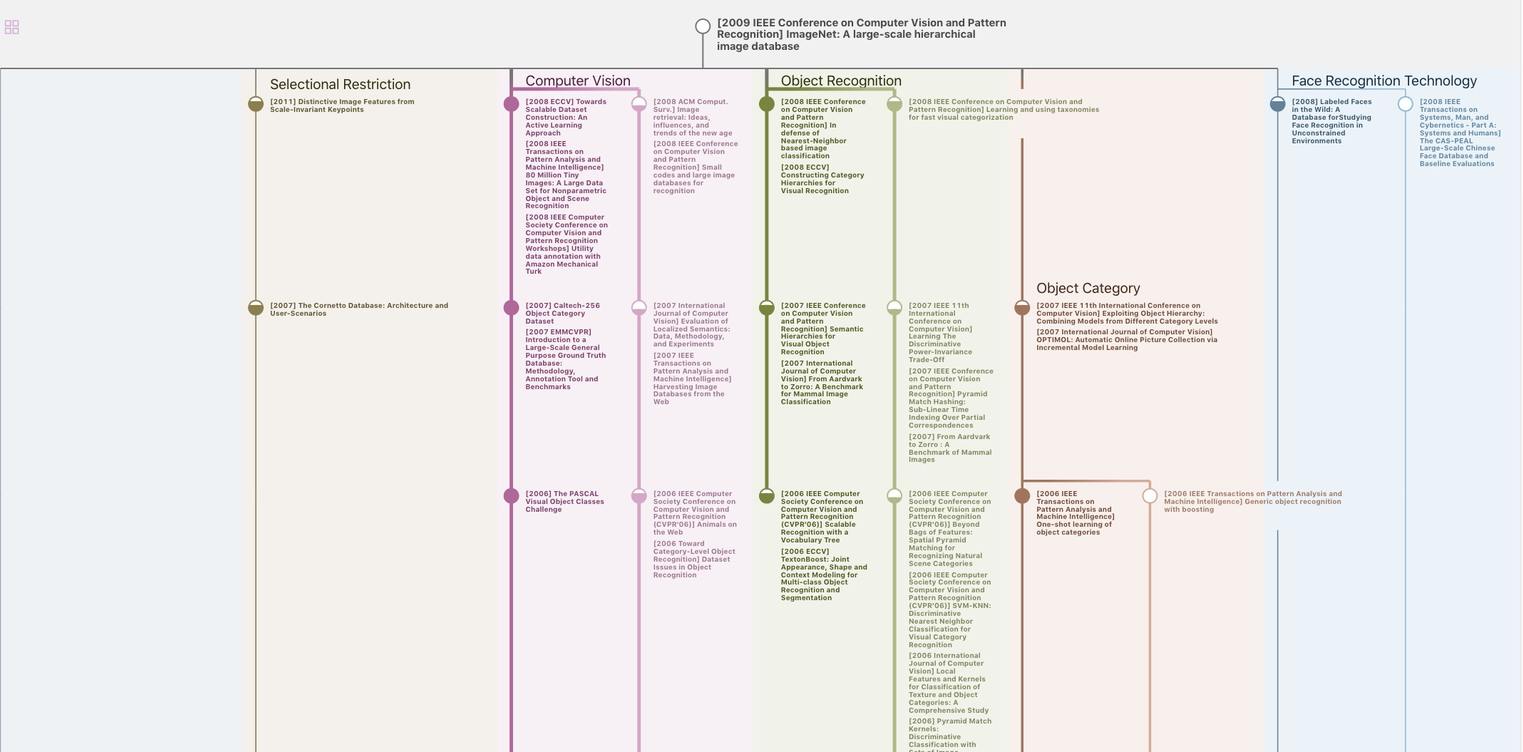
生成溯源树,研究论文发展脉络
Chat Paper
正在生成论文摘要