Fast Adaptation to Super-Resolution Networks via Meta-learning
European Conference on Computer Vision(2020)
摘要
Conventional supervised super-resolution (SR) approaches are trained with massive external SR datasets but fail to exploit desirable properties of the given test image. On the other hand, self-supervised SR approaches utilize the internal information within a test image but suffer from computational complexity in run-time. In this work, we observe the opportunity for further improvement of the performance of single-image super-resolution (SISR) without changing the architecture of conventional SR networks by practically exploiting additional information given from the input image. In the training stage, we train the network via meta-learning; thus, the network can quickly adapt to any input image at test time. Then, in the test stage, parameters of this meta-learned network are rapidly fine-tuned with only a few iterations by only using the given low-resolution image. The adaptation at the test time takes full advantage of patch-recurrence property observed in natural images. Our method effectively handles unknown SR kernels and can be applied to any existing model. We demonstrate that the proposed model-agnostic approach consistently improves the performance of conventional SR networks on various benchmark SR datasets.
更多查看译文
关键词
Deep learning,Meta-learning,Single-image super-resolution,Patch recurrence
AI 理解论文
溯源树
样例
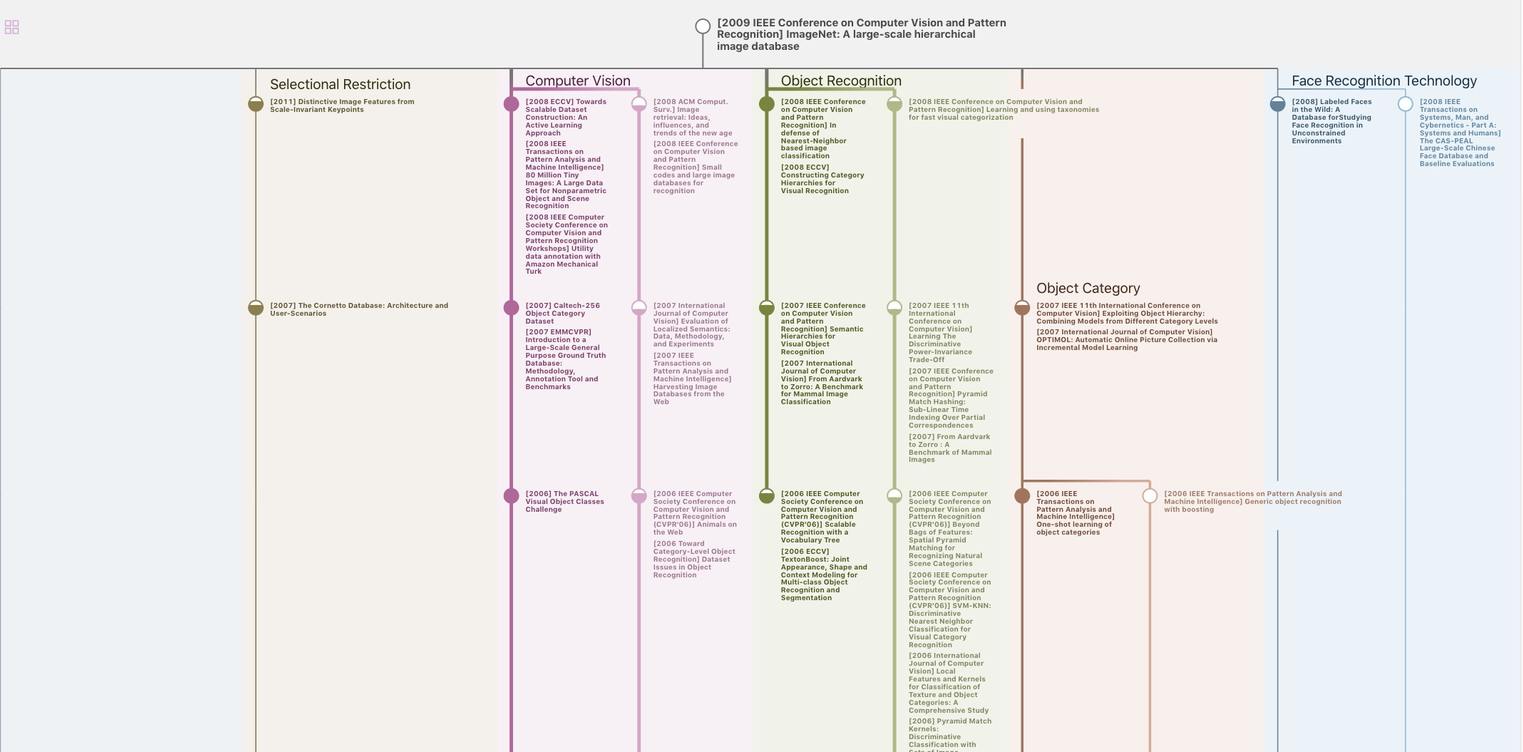
生成溯源树,研究论文发展脉络
Chat Paper
正在生成论文摘要