Multimodal Medical Image Sensor Fusion Model Using Sparse K-SVD Dictionary Learning in Nonsubsampled Shearlet Domain
IEEE Transactions on Instrumentation and Measurement(2020)
摘要
Multimodal medical image sensor fusion (MMISF) has a significant role for better visualization of the diagnostic statistics computed by integrating the vital information taken from input source images acquired using multimodal imaging sensors. The MMISF also helps the medical professionals in precise diagnosis of several critical diseases and its treatment. Often, images taken from different imaging sensors are degraded by noise interferences during acquisition or data transmission that leads to the false perception of noise as a useful feature of the image. This paper presents a novel fusion framework for multimodal neurological images, which is able to capture small-scale details of input images with original structural details. In its procedural steps, at first, source images get decomposed by the nonsubsampled shearlet transform (NSST) into a low-frequency
(lf)
and several high-frequency
(hf)
components to separate out the two basic characteristics of source image, i.e., principal information and edge details. The
lf
layers get fused with a sparse representation-based model, and the
hf
components are merged by the guided filtering-based approach. Finally, fused images are reconstructed by employing the inverse NSST. The superiority of the proposed MMISF approach is confirmed by a large extent of analytical experimentations on the different real magnetic resonance single-photon emission computed tomography, magnetic resonance-positron emission tomography and computed tomography magnetic resonance neurological image data sets. Based on all these experimental results, it is stated that the proposed MMISF approach is superior to several other approaches as it produces better visually fused images with improved computational measures.
更多查看译文
关键词
Transforms,Visualization,Medical diagnostic imaging,Image sensors,Sensors
AI 理解论文
溯源树
样例
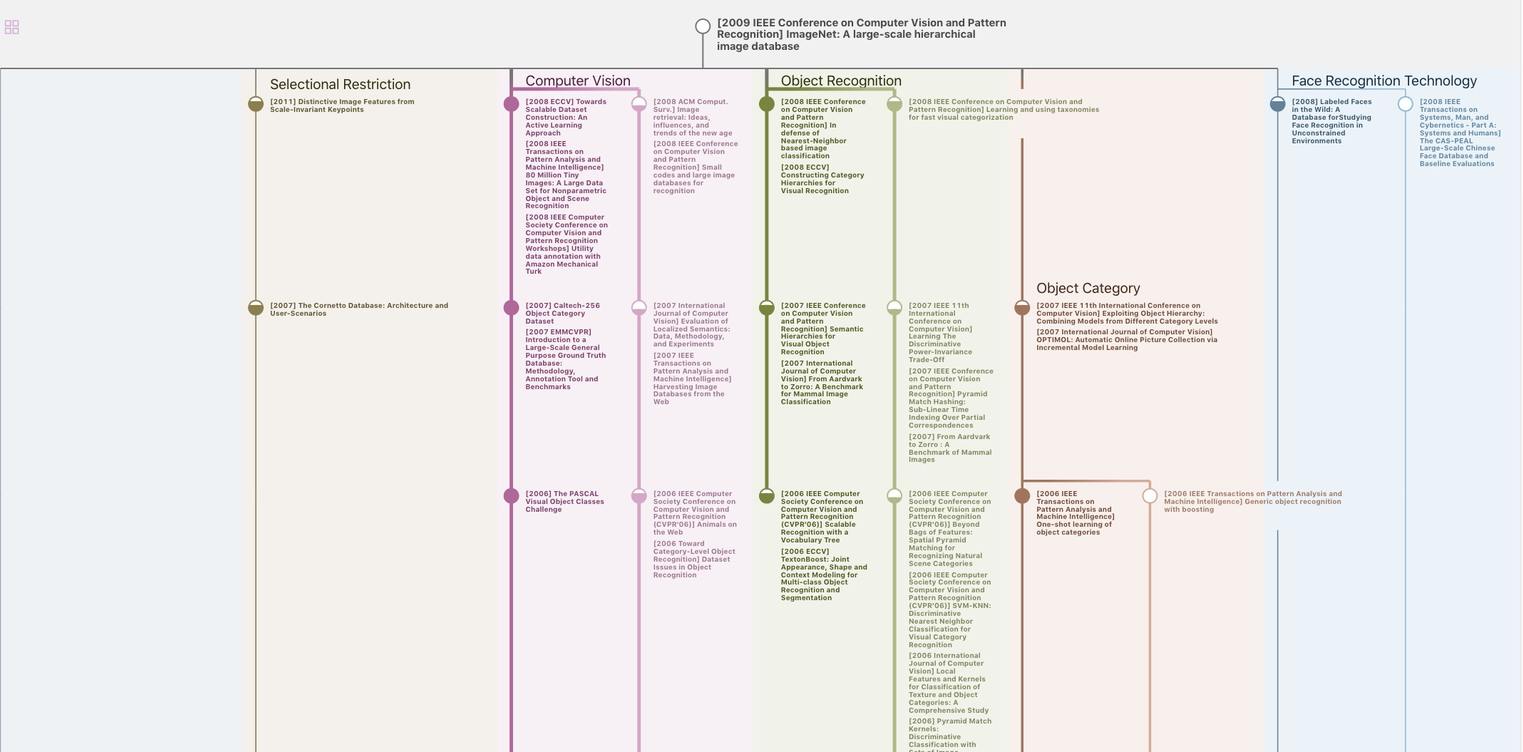
生成溯源树,研究论文发展脉络
Chat Paper
正在生成论文摘要