Video Depth Estimation by Fusing Flow-to-Depth Proposals
IROS(2020)
摘要
We present an approach with a novel differentiable flow-to-depth layer for video depth estimation. The model consists of a flow-to-depth layer, a camera pose refinement module, and a depth fusion network. Given optical flow and camera pose, our flow-to-depth layer generates depth proposals and the corresponding confidence maps by explicitly solving an epipolar geometry optimization problem. Unlike other methods, our flow-to-depth layer is differentiable, and thus we can refine camera poses by maximizing the aggregated confidence in camera pose refinement module. Our depth fusion network can utilize depth proposals and their confidence maps inferred from different adjacent frames to produce the final depth map. Furthermore, the depth fusion network can additionally take the depth proposals generated by other methods to improve the results further. The experiments on three public datasets show that our approach outperforms state-of-the-art depth estimation methods, and has strong generalization capability: our model trained on KITTI performs well on the unseen Waymo dataset while other methods degenerate a lot.
更多查看译文
关键词
flow-to-depth layer,refinement module,depth fusion network,final depth map,state-of-the-art depth estimation methods,video depth estimation,flow-to-depth proposals,given optical flow
AI 理解论文
溯源树
样例
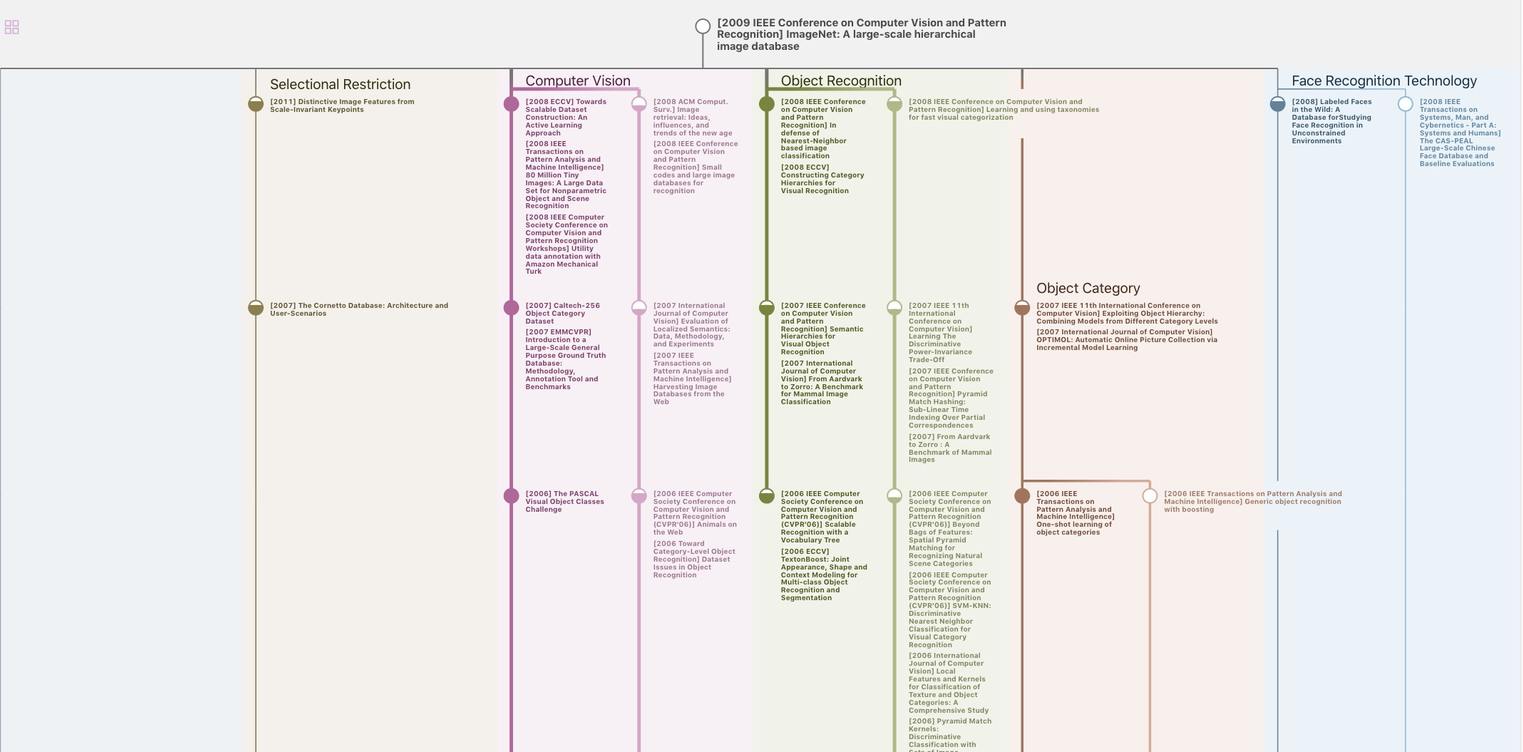
生成溯源树,研究论文发展脉络
Chat Paper
正在生成论文摘要