P145 Identifying people most at risk of a severe asthma attack using routine electronic healthcare record data
THORAX(2019)
摘要
Background Although many of the individual risk factors for asthma attacks are known, there is no published algorithm, using routinely available electronic health record data, to predict those individuals who are at a high risk of severe asthma attacks in primary care. We aimed to develop such an algorithm, so that individuals could be identified for a trial evaluating at-risk registers in primary care (the ARRISA-UK trial). Methods Multivariable logistic regression was applied to a large dataset of 61,861 people with a history of asthma from England and Scotland from Clinical Practice Research Datalink (CPRD) and external validation using the Secure Anonymised Information Linkage (SAIL) databank of 174,240 patients from Wales. We defined a severe asthma attack as one resulting in one or more hospitalisation or A&E attendance (development dataset) and asthma-related hospitalisation, A&E attendance or death (validation dataset) within a 12-month period. Results 969 (1.65%) patients (derivation data) and 2,439 (1.40%) (validation dataset) experienced one or more severe asthma attacks. Risk factors for asthma attacks were: previous hospitalisation, older age group, lower body mass index, smoking, blood eosinophilia, presence of diabetes diagnosis/therapy, ischaemic heart disease, anxiety/depression, history of anaphylaxis but not rhinitis, primary care consultations for lower respiratory tract infection, oral steroid courses and paracetamol, either no asthma treatment or high GINA step. This algorithm had good predictive ability with a Receiver Operating Characteristic (ROC) of 0.71 (95% CI 0.70 – 0.72) in the validation dataset. Those at highest risk of an attack (top 7%, 20–30 people/practice of 8,000 patients) had a positive predictive value of 5.7% (95% CI 5.3 – 6.1) and a negative predictive value of 98.9% (98.9 – 99.0), with 28.5% (26.7 – 30.3) sensitivity and specificity of 93.3% (93.2 – 93.4) Conclusions This externally validated algorithm, the ARRISA-UK At-Risk Algorithm, derived from data within routine primary care electronic health records has good predictive ability for identifying patients at high risk of severe asthma attacks and excluding individuals not at high risk. We are able to use this algorithm to determine whether prioritising care for these individuals reduces hospital admissions.
更多查看译文
AI 理解论文
溯源树
样例
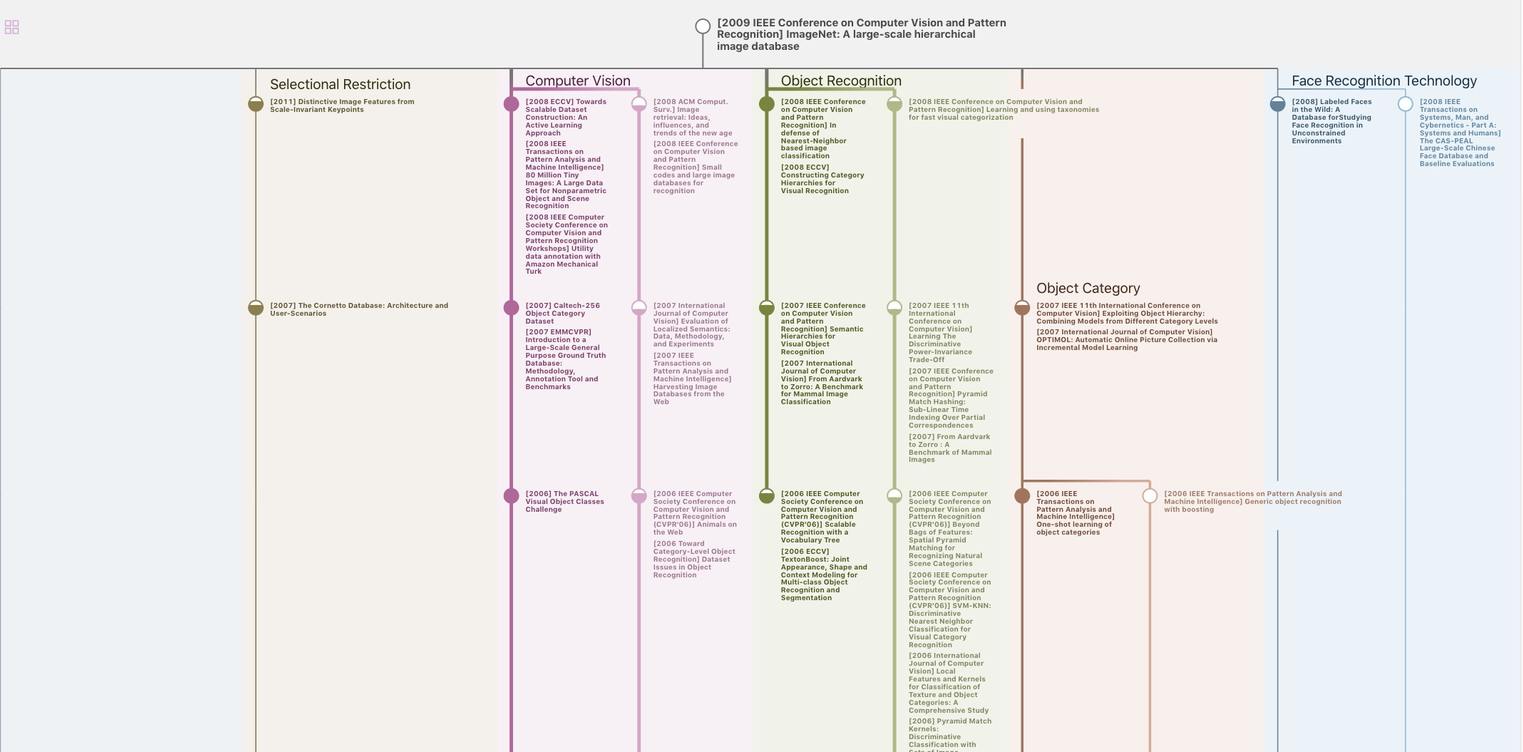
生成溯源树,研究论文发展脉络
Chat Paper
正在生成论文摘要