Do different cross‐project defect prediction methods identify the same defective modules?
Periodicals(2020)
摘要
AbstractAbstractCross‐project defect prediction (CPDP) is needed when the target projects are new projects or the projects have less training data, since these projects do not have sufficient historical data to build high‐quality prediction models. The researchers have proposed many CPDP methods, and previous studies have conducted extensive comparisons on the performance of different CPDP methods. However, to the best of our knowledge, it remains unclear whether different CPDP methods can identify the same defective modules, and this issue has not been thoroughly explored. In this article, we select 12 state‐of‐the‐art CPDP methods, including eight supervised methods and four unsupervised methods. We first compare the performance of these methods in the same experiment settings on five widely used datasets (ie, NASA, SOFTLAB, PROMISE, AEEEM, and ReLink) and rank these methods via the Scott‐Knott test. Final results confirm the competitiveness of unsupervised methods. Then we perform diversity analysis on defective modules for these methods by using the McNemar test. Empirical results verify that different CPDP methods may lead to difference in the modules predicted as defective, especially when the comparison is performed between the supervised methods and unsupervised methods. Finally, we also find there exist a certain number of defective modules, which cannot be correctly identified by any of the CPDP methods or can be correctly identified by only one CPDP method. These findings can be utilized to design more effective methods to further improve the performance of CPDP.
更多查看译文
关键词
cross-project defect prediction,diversity analysis,empirical study,software defect prediction
AI 理解论文
溯源树
样例
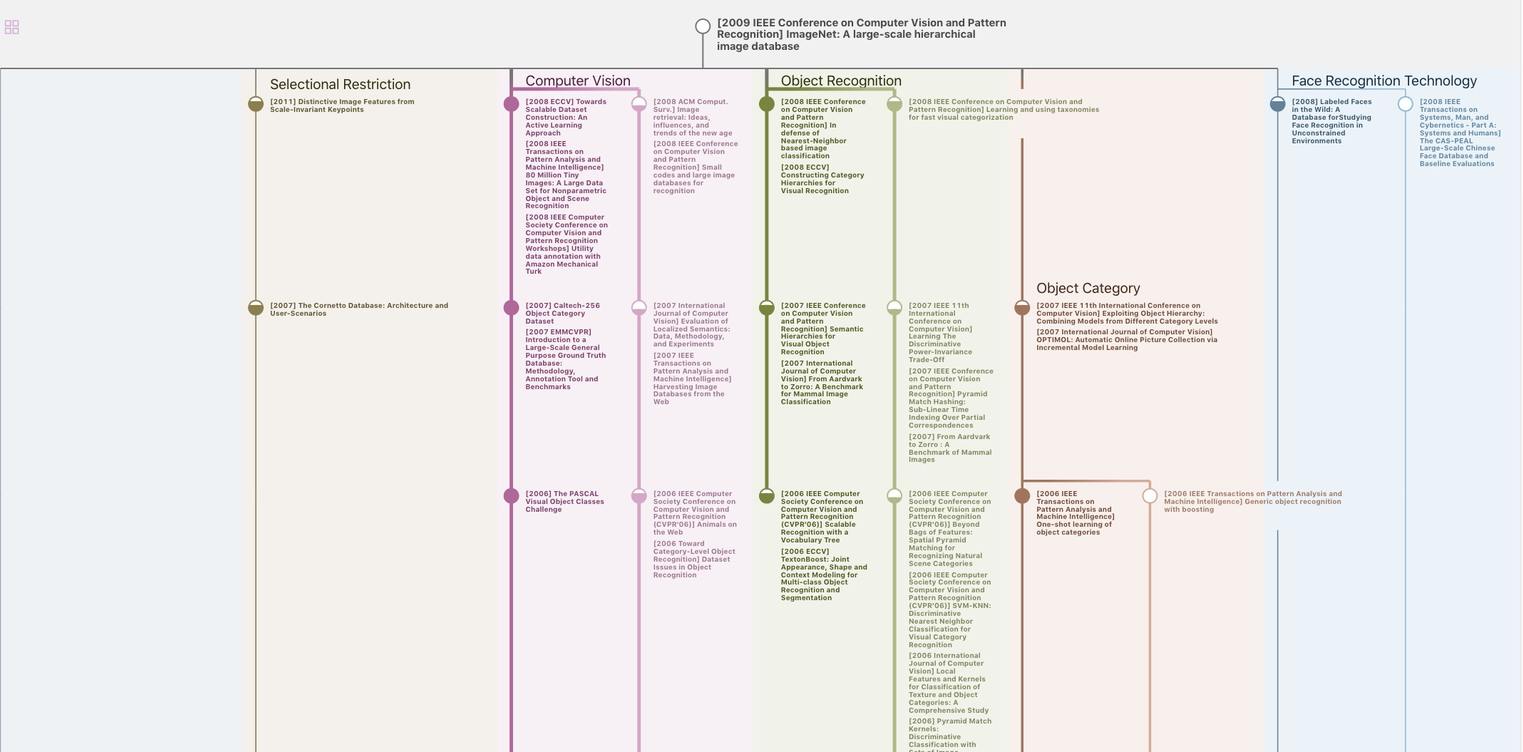
生成溯源树,研究论文发展脉络
Chat Paper
正在生成论文摘要