Quantifying the Relative Importance of Variables and Groups of Variables in Remote Sensing Classifiers Using Shapley Values and Game Theory
IEEE Geoscience and Remote Sensing Letters(2020)
摘要
Remote sensing image classification applications often involve determining which variables are the most important to obtain the best accuracy. Common metrics for assessing variable importance such as a mean decrease in accuracy (MDA) typically provide values in scaled units that are difficult to interpret, and do not easily accommodate user-defined groups of variables. In this letter, an improved method of quantifying the importance of classifier variables is developed and demonstrated in the context of land-cover classification using the random forest algorithm. The proposed method employs concepts from game theory and relies on a metric known as the Shapley value, which allows the importance of variables to be easily interpreted by providing a quantitative assessment of each variable’s contribution to classifier accuracy. Moreover, unlike MDA, the method also applies to arbitrary, user-defined groups of variables. The approach described herein thus provides a robust alternative to single-variable selection using MDA and can be used with any type of classifier.
更多查看译文
关键词
Games,Measurement,Remote sensing,Game theory,Laser radar,Correlation
AI 理解论文
溯源树
样例
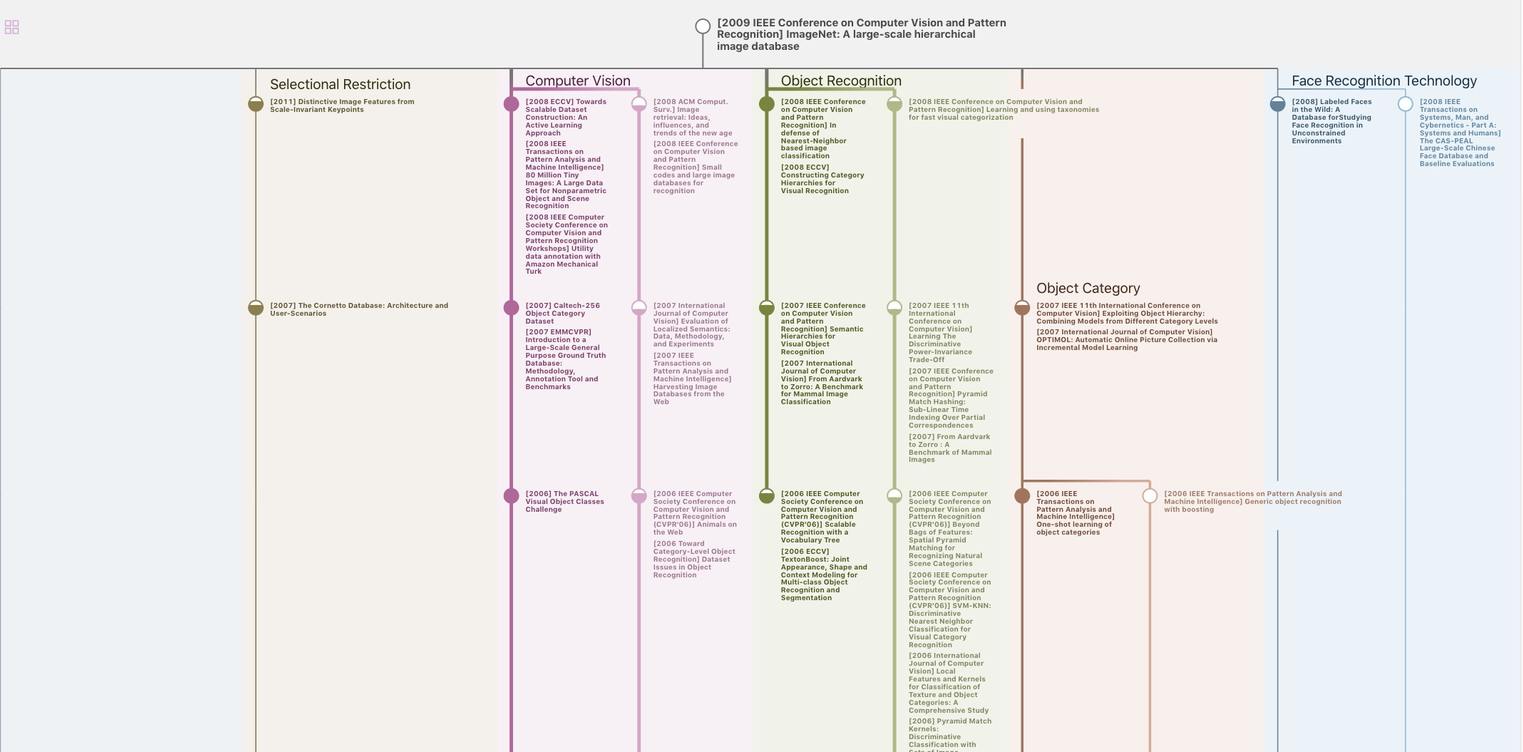
生成溯源树,研究论文发展脉络
Chat Paper
正在生成论文摘要