Cow estrus detection with low-frequency accelerometer sensor by unsupervised learning
Proceedings of the Tenth International Symposium on Information and Communication Technology(2019)
摘要
In recent years, Internet of Things (IoT) and Machine Learning (ML) has been applied successfully in agriculture. These technologies increase productivity as well as reduce labor significantly. In this paper, we focus on improving the autonomous cow estrus detection system in terms of energy consumption and precision. In previous detection pipelines, an accelerometer is mounted to the neck of cows to capture motion data with high frequency, followed by the ML algorithm to check the data and determine whether it is in estrus or not. Instead, we configured the accelerometer to sample with low frequency for minimizing its energy consumption. However, low-sampling rate as input of ML pipeline leads to an undesirable higher false alarm rate. To solve this problems, we designed a pipeline of unsupervised learning with a new heuristic post-processing algorithm. The proposed post-processing algorithm is a backtracking algorithm that incorporates the timing constraint of the period obtained by agriculture knowledge. With the constraint, the post-processing algorithm facilitates a significantly higher precision than simple adaptive threshold techniques in previous studies on a simulated dataset. Finally, the overall result of the pipeline with the proposed algorithm is visualized on real-world data captured on the farm in our agriculture department.
更多查看译文
关键词
estrus detection, internet of things, unsupervised learning
AI 理解论文
溯源树
样例
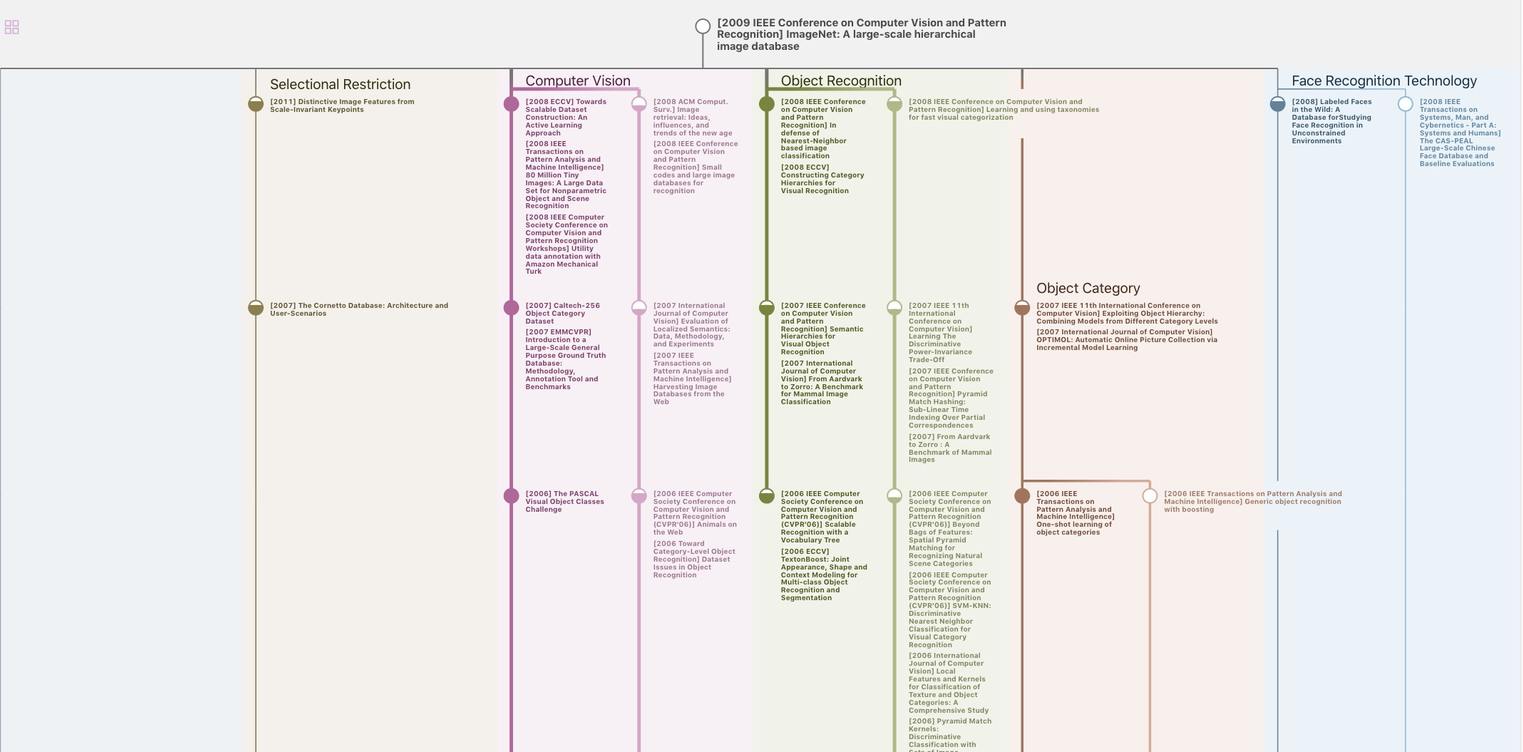
生成溯源树,研究论文发展脉络
Chat Paper
正在生成论文摘要