Comparing different venous thromboembolism risk assessment machine learning models in Chinese patients.
JOURNAL OF EVALUATION IN CLINICAL PRACTICE(2020)
摘要
Objective: Venous thromboembolism (VTE) is a fatal complication and the most common preventable cause of death in hospitals. The risk-to-benefit ratio of thromboprophylaxis depends on the performance of the risk assessment model. A linear model, the Padua model, is recommended for medical inpatients in the United States but is not suitable for Chinese inpatients due to differences in race and disease spectrum. Currently, machine learning (ML) methods show advantages in modeling complex data patterns and have been applied to clinical data analysis. This study aimed to build VTE risk assessment ML models among Chinese inpatients and compare the predictive validity of the ML models with that of the Padua model. Methods: We used 376 patients, including 188 patients with VTE, to build a model and then evaluate the predictive validity of the model in a consecutive clinical dataset from Peking Union Medical College Hospital. Nine widely used ML methods were trained on the model derivation set and then compared with the Padua model. Results: Among the nine ML methods, random forest (RF), boosting-based methods, and logistic regression achieved a higher specificity, Youden index, positive predictive value, and area under the receiver operating characteristic curve than the Padua model on both the test and clinical validation sets. However, their sensitivities were inferior to that of the Padua model. Combined with the receiver operating characteristic curve, RF, as the best performing model, maintained high specificity with relatively better sensitivity and captured VTE patients' patterns more precisely. Conclusions: Advances in ML technology provide powerful tools for medical data analysis, and choosing models conforming to the disease pattern would achieve good performance. Popular ML models do not surpass the Padua model on all indicators of validity, and the drawback of low sensitivity should be improved upon in the future.
更多查看译文
关键词
machine learning,medical informatics,pulmonary diseases,risk assessment,thromboembolism
AI 理解论文
溯源树
样例
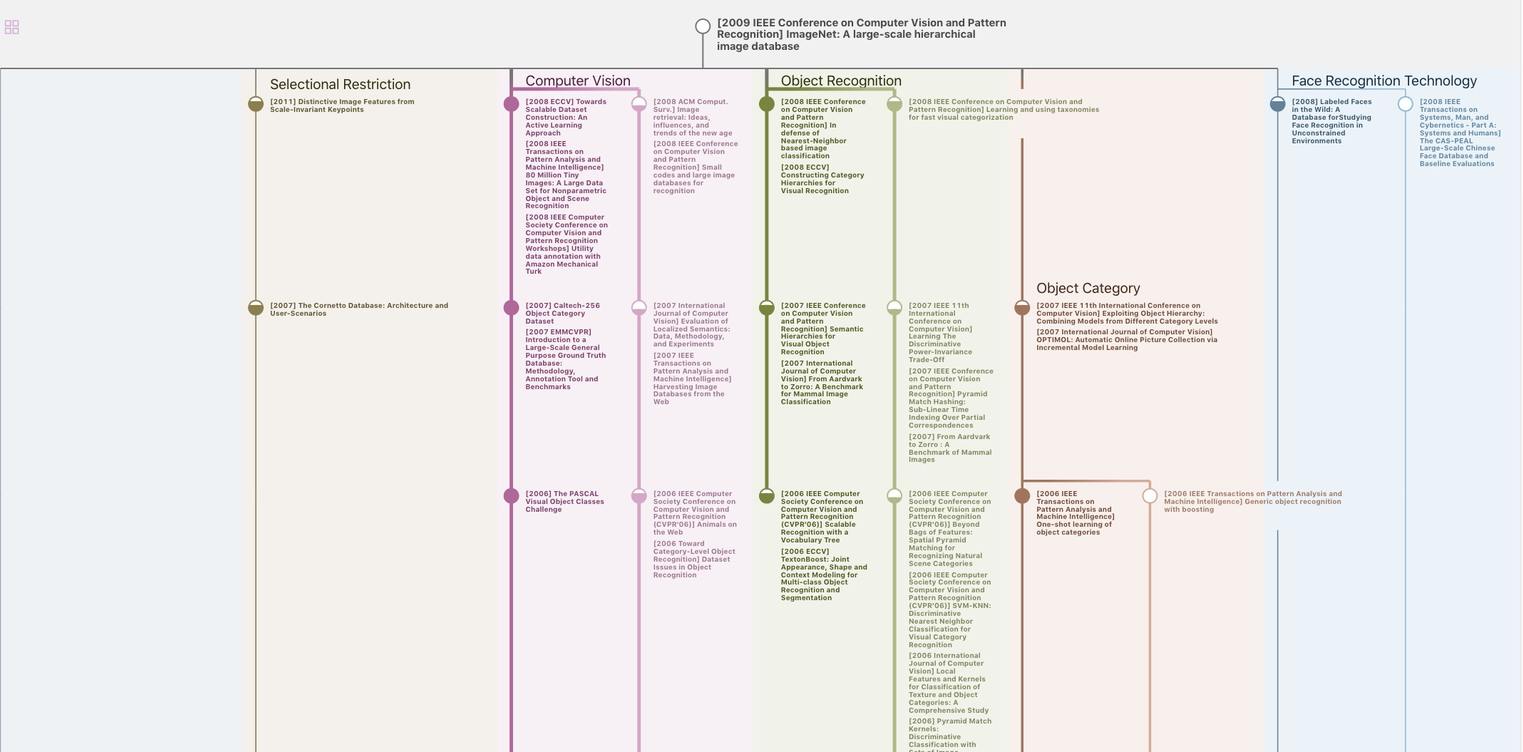
生成溯源树,研究论文发展脉络
Chat Paper
正在生成论文摘要