Event Detection in Micro-PMU Data: A Generative Adversarial Network Scoring Method
power and energy society general meeting(2020)
摘要
A new data-driven method is proposed to detect events in the data streams from \emph{distribution-level phasor measurement units}, a.k.a., micro-PMUs. The proposed method is developed by constructing \emph{unsupervised deep learning} anomaly detection models; thus, providing event detection algorithms that require \emph{no or minimal human knowledge}. First, we develop the core components of our approach based on a Generative Adversarial Network (GAN) model. We refer to this method as the \emph{basic} method. It uses the same features that are often used in the literature to detect events in micro-PMU data. Next, we propose a second method, which we refer to as the \emph{enhanced} method, which is enforced with additional feature analysis. Both methods can detect \emph{point signatures} on single features and also \emph{group signatures} on multiple features. This capability can address the unbalanced nature of power distribution circuits. The proposed methods are evaluated using \emph{real-world} micro-PMU data. We show that both methods highly outperform a state-of-the-art statistical method in terms of the event detection accuracy. The enhanced method also outperforms the basic method.
更多查看译文
关键词
Micro-PMU data, power distribution, event detection, deep learning, generative adversarial network, feature analysis
AI 理解论文
溯源树
样例
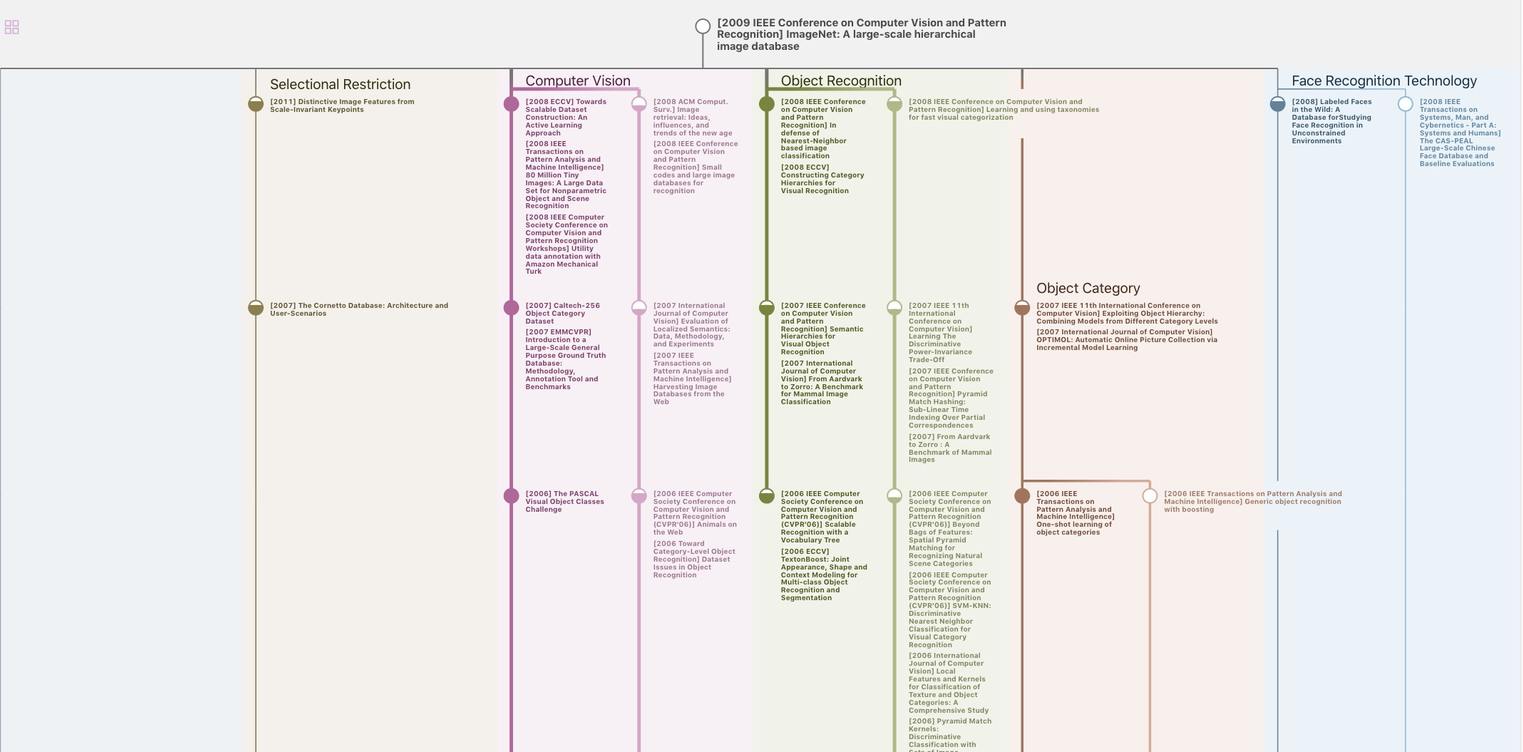
生成溯源树,研究论文发展脉络
Chat Paper
正在生成论文摘要