Learning a Neural 3D Texture Space from 2D Exemplars
CVPR(2020)
摘要
We propose a generative model of 2D and 3D natural textures with diversity, visual fidelity and at high computational efficiency. This is enabled by a family of methods that extend ideas from classic stochastic procedural texturing (Perlin noise) to learned, deep, non-linearities. The key idea is a hard-coded, tunable and differentiable step that feeds multiple transformed random 2D or 3D fields into an MLP that can be sampled over infinite domains. Our model encodes all exemplars from a diverse set of textures without a need to be re-trained for each exemplar. Applications include texture interpolation, and learning 3D textures from 2D exemplars.
更多查看译文
关键词
stochastic procedural texturing,3D natural texture,2D natural texture,texture interpolation,Perlin noise,2D exemplars,neural 3D texture space
AI 理解论文
溯源树
样例
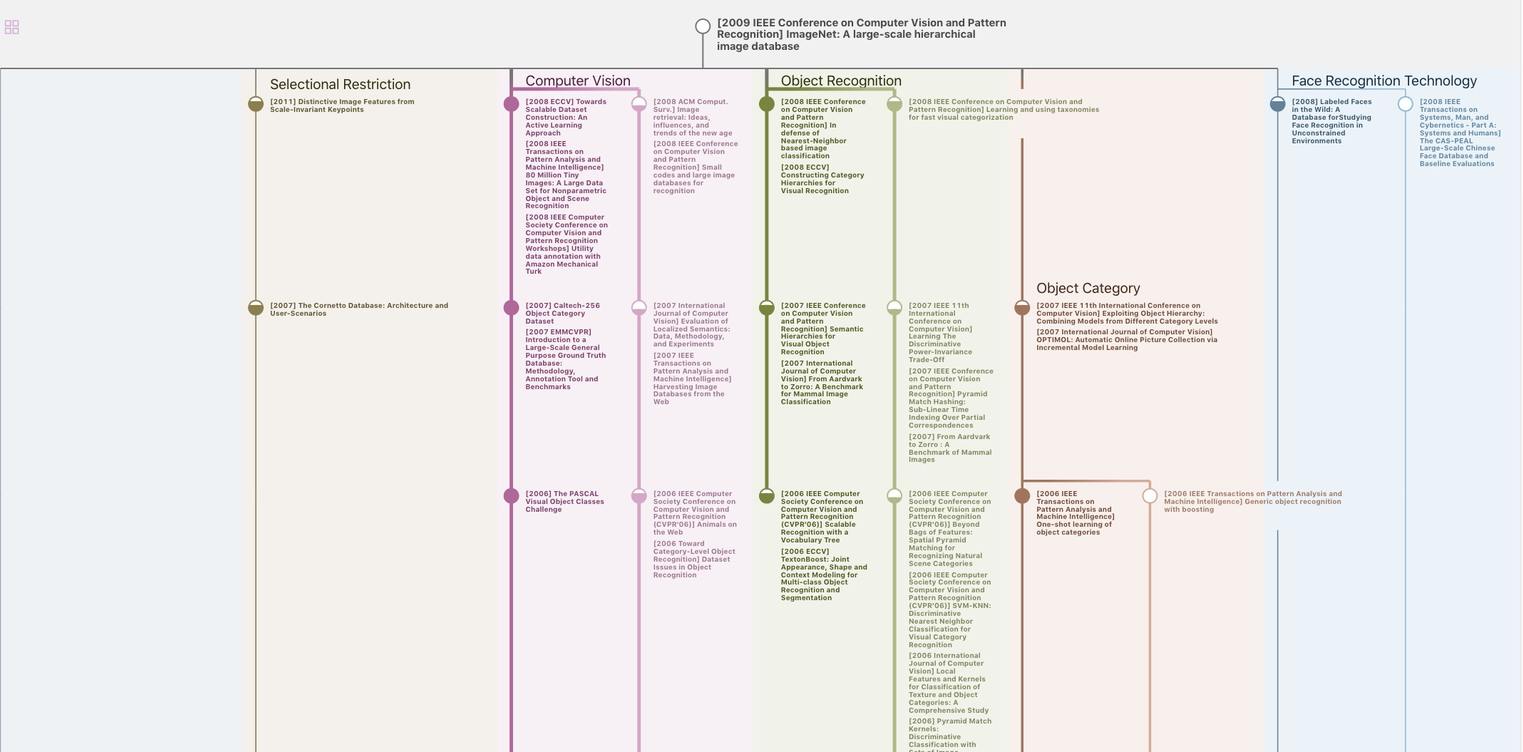
生成溯源树,研究论文发展脉络
Chat Paper
正在生成论文摘要