Multi-step Forecasting via Multi-task Learning
2019 IEEE International Conference on Big Data (Big Data)(2019)
摘要
Multi-task learning is an established approach for improving the generalization of a model. We explore multi-task learning in the context of time series forecasting. Specifically, we look into a multivariate setting where main and auxiliary series are to be forecasted for multi-step ahead. This results in an interesting multi-task learning problem formulation where the learning tasks come from future horizon of main and auxiliary series both. Our proposed method relies firstly on enumerating multiple Convolutional network architectures to balance the number of shared and non-shared layers between different time series tasks. Also, as multi-step strategies minimize forecast errors over the complete horizon, loss functions would be at different scales based on model uncertainty for near versus distant future. For this reason we propose a factorization of the weight vector for the learning tasks with respect to their categorization of belonging to main or auxiliary series and index in future. An optimal number of shared and non-shared layers together with a novel weighted loss, results in superior performance over 2 real-world datasets compared with several baselines.
更多查看译文
关键词
convolutional network architectures,multitask learning,learning tasks,time series forecasting,multistep forecasting
AI 理解论文
溯源树
样例
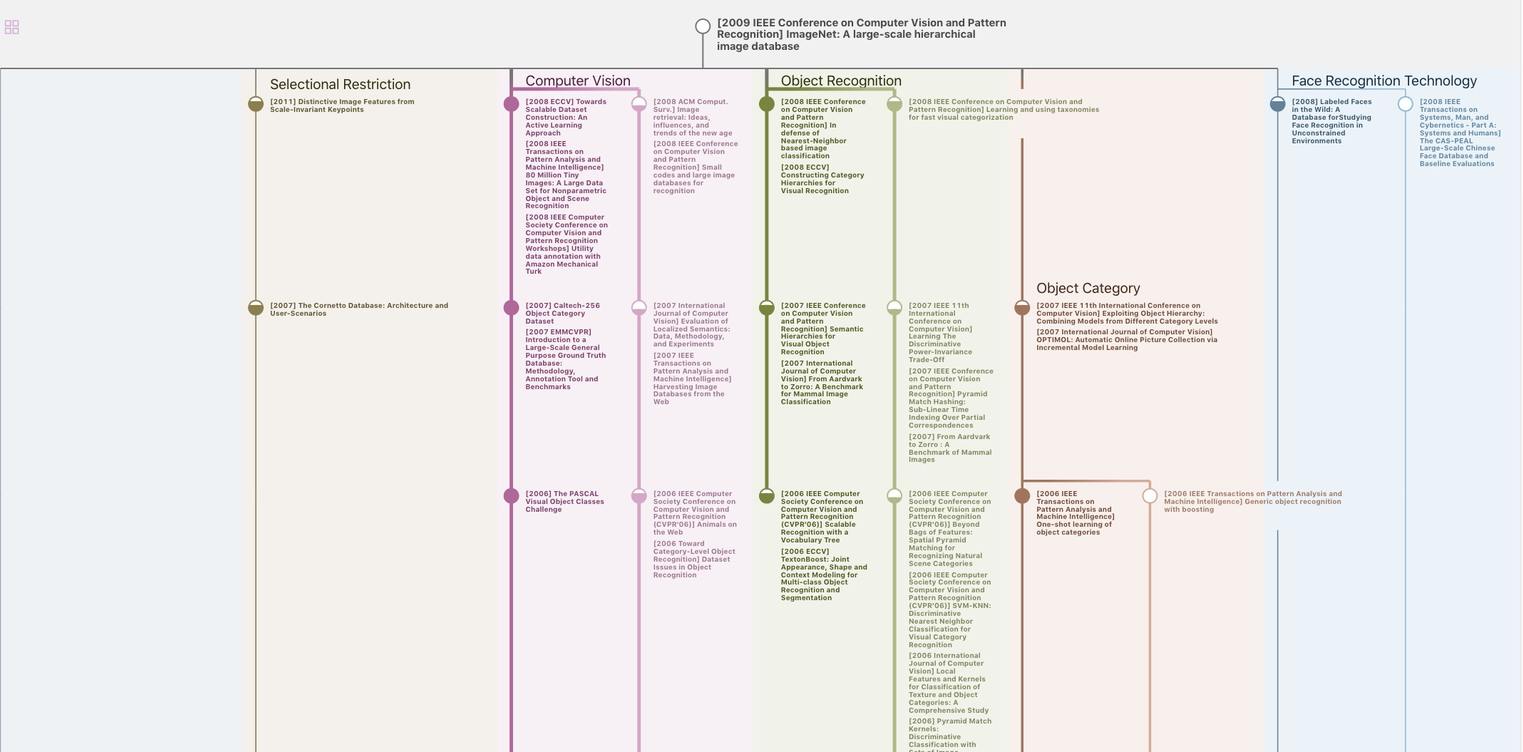
生成溯源树,研究论文发展脉络
Chat Paper
正在生成论文摘要