Hyper-spectral image segmentation using an improved PSO aided with multilevel fuzzy entropy
Multimedia Tools and Applications(2019)
摘要
This paper proposes a novel histogram-based multi-level segmentation scheme of hyper-spectral images. In the proposed scheme an Improved Particle Swarm Optimization (IPSO) algorithm is implemented as a nature-inspired evolutionary algorithm to overcome the drawback of premature convergence and hence getting stuck in local optima problem of PSO. The high-dimension of PSO is decomposed into several one-dimensional problems and premature convergence is removed from each one-dimensional problem. This algorithm is further extended for replacing the worst particles by the fittest particles, determined by their fitness values. Multiple optimal threshold values have been evaluated based on fuzzy-entropy aided with the proposed algorithm. The performance of the IPSO is compared statistically with other global optimization algorithms namely Cuckoo Search (CS), Differential Evolution (DE), FireFly (FF), Genetic Algorithm (GA), and PSO. The produced segmented output of IPSO-fuzzy is then combined with the available ground truth values of image classes to train a Support Vector Machine (SVM) classifier via the composite kernel approach to improving the classification accuracy. This hybrid approach (IPSO-SVM) is then applied to popular hyper-spectral imageries acquired by AVRIS and ROSIS sensors. The final evaluated outcomes of the proposed scheme are also qualitatively compared to show its effectiveness over the other state-of-art global optimizers.
更多查看译文
关键词
Improved particle swarm optimization (IPSO), Multilevel-thresholding, Hyper-spectral imageries, Fuzzy entropy, Support vector machine (SVM), Composite kernel
AI 理解论文
溯源树
样例
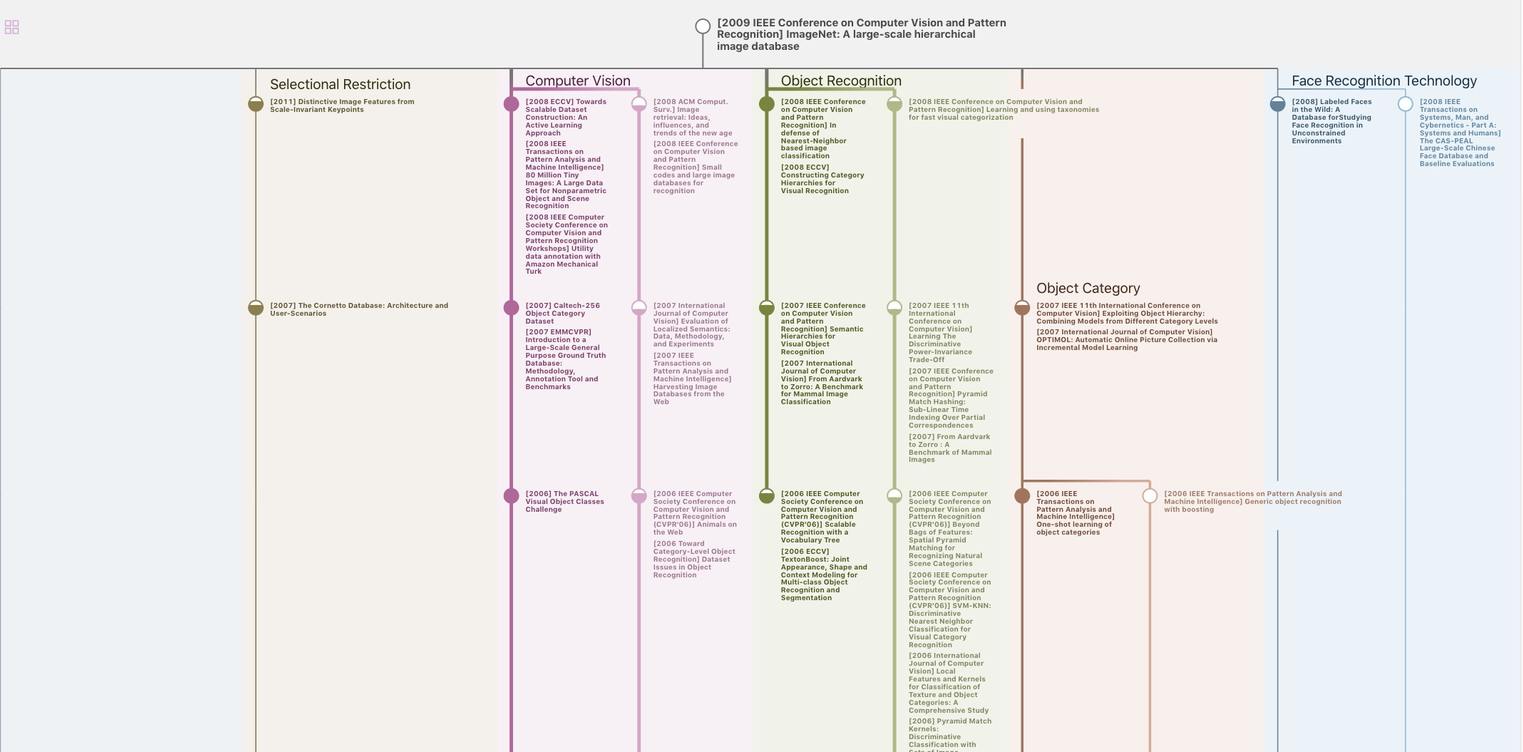
生成溯源树,研究论文发展脉络
Chat Paper
正在生成论文摘要