Gated and attentive neural collaborative filtering for user generated list recommendation.
Knowledge-Based Systems(2020)
摘要
Recommending user generated lists (e.g., playlists) has become an emerging task in many online systems. Many existing list recommendation methods predict user preferences on lists by aggregating their preferences on individual items, which neglects the list-level information (e.g., list attributes) and thus results in suboptimal performance. This paper proposes a neural network-based solution for user generated list recommendation, which can leverage both item-level information and list-level information to improve performance. Firstly, a representation learning network with attention and gate mechanism is proposed to learn the user embeddings, item embeddings and list embeddings simultaneously. Then, an interaction network is proposed to learn user–item interactions and user–list interactions, in which the two kinds of interactions can share the convolution layers to further improve performance. Experimental studies on two real-world datasets demonstrate that (1) the proposed representation learning network can learn more representative user/item/list embedding than existing methods and (2) the proposed solution can outperform state-of-the-art methods in both item recommendation and list recommendation in terms of accuracy.
更多查看译文
关键词
Recommender systems,Collaborative filtering,Neural network,List recommendation
AI 理解论文
溯源树
样例
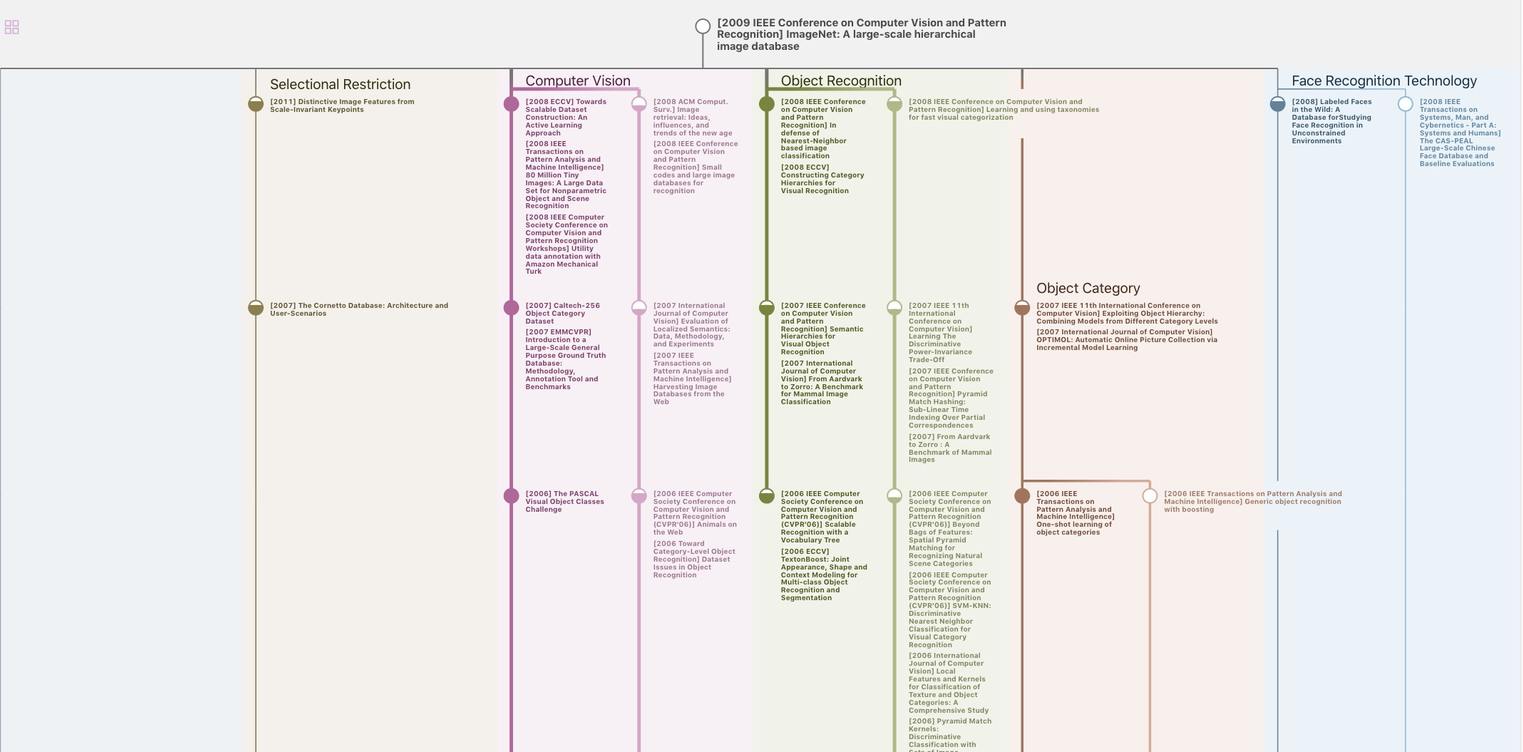
生成溯源树,研究论文发展脉络
Chat Paper
正在生成论文摘要