A CNN-Based Framework for Statistical Assessment of Spinal Shape and Curvature in Whole-Body MRI Images of Large Populations
Lecture Notes in Computer Science(2019)
摘要
The extraction of spines from medical records in a fast yet accurate way is a challenging task, especially for large data sets. Addressing this issue, we present a framework based on convolutional neural networks for the reconstruction of the spinal shape and curvature, making statistical assessments feasible on epidemiological scale. Our method uses a two-step strategy. First, anchor vertebrae and the spinal centerline in between them get extracted. Second, the centerlines are transformed into a common coordinate system to enable comparisons and statistical assessments across subjects. Our networks were trained on 103 subjects, where we achieved accuracies of 3.3mm on average, taking at most 1 s per record, which eases the handling of even very large cohorts. Without any further training, we validated our model on study data of about 3400 subjects with only 10 cases of failure, which demonstrates the robustness of our method with respect to the natural variability in spinal shape and curvature. A thorough statistical analysis of the results underpins the importance of our work. Specifically, we show that the spinal curvature is significantly influenced by the body mass index of a subject. Moreover, we show that the same findings arise when Cobb angles are considered instead of direct curvature measures. To this end, we propose a generalization of classical Cobb angles that can be evaluated algorithmically and can also serve as a useful (visual) tool for physicians in everyday clinical practice.
更多查看译文
AI 理解论文
溯源树
样例
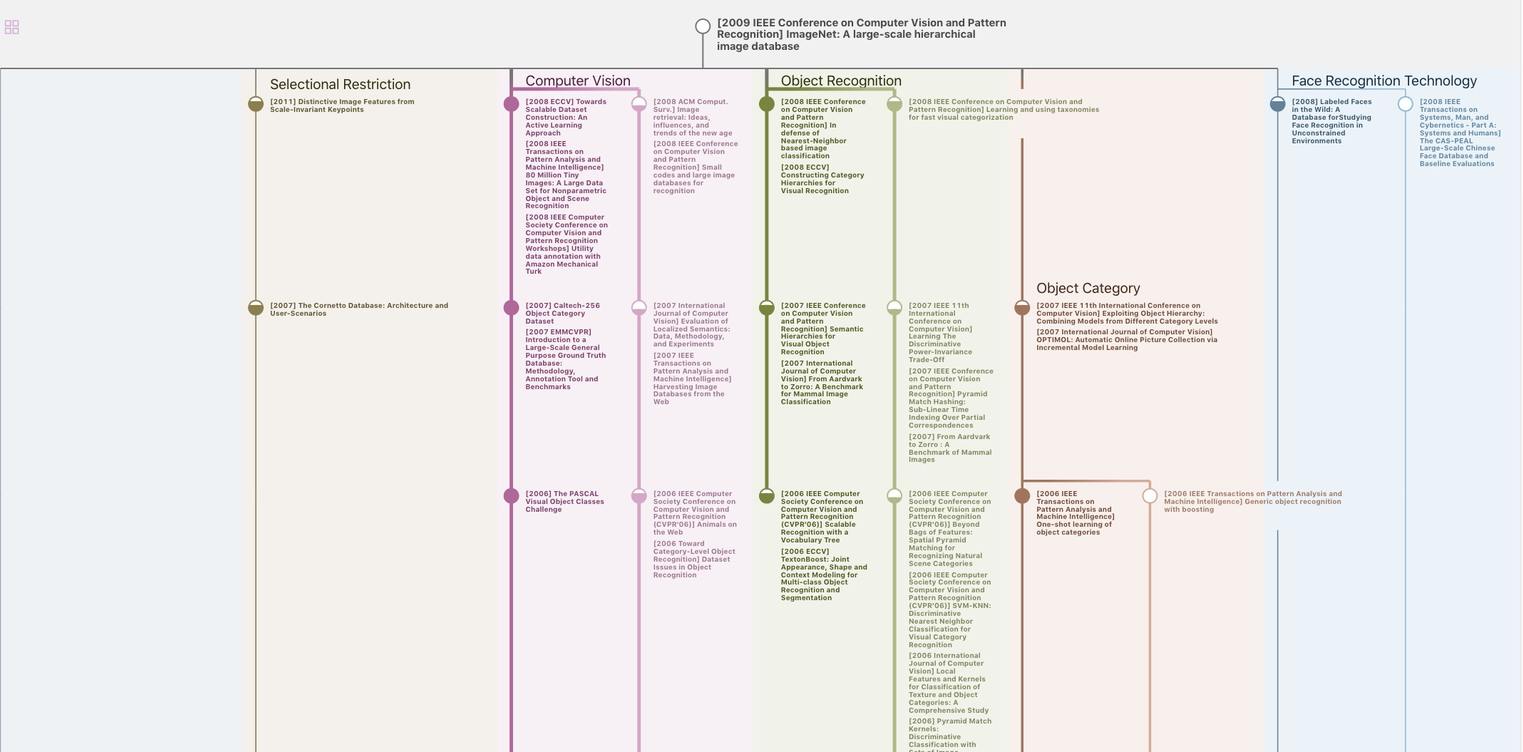
生成溯源树,研究论文发展脉络
Chat Paper
正在生成论文摘要