Fast and Effective Learning for Fuzzy Cognitive Maps: A Method Based on Solving Constrained Convex Optimization Problems
IEEE Transactions on Fuzzy Systems(2020)
摘要
The learning of fuzzy cognitive maps (FCMs) is a timely issue pursued by numerous researchers. Many learning methods, such as population-based algorithms and some hybrid algorithms, have been developed and applied to many fields resulting in better performance. However, those learning algorithms also exhibit obvious limitations: some of them either are difficult to handle the learning problem of large-scale FCM or extremely time-consuming with high computational overload. Furthermore, the learning problem of FCM with noisy data is rarely considered in existing algorithms. In this article, a fast and efficient method for learning FCM is proposed. It first transforms the learning problem of the FCM into a convex optimization problem with constraints, and then, the classic interior-point methods are invoked to solve the optimization problem to obtain the optimized weight matrix of the FCM. A series of experiments involving synthetic data, noisy synthetic data, real data, and publicly available data with noise are considered to demonstrate that the proposed method can rapidly and efficiently learn small-scale and large-scale FCMs and deal with the problem of learning FCM from noisy historical data.
更多查看译文
关键词
Convex optimization,fuzzy cognitive maps (FCMs),learning algorithm
AI 理解论文
溯源树
样例
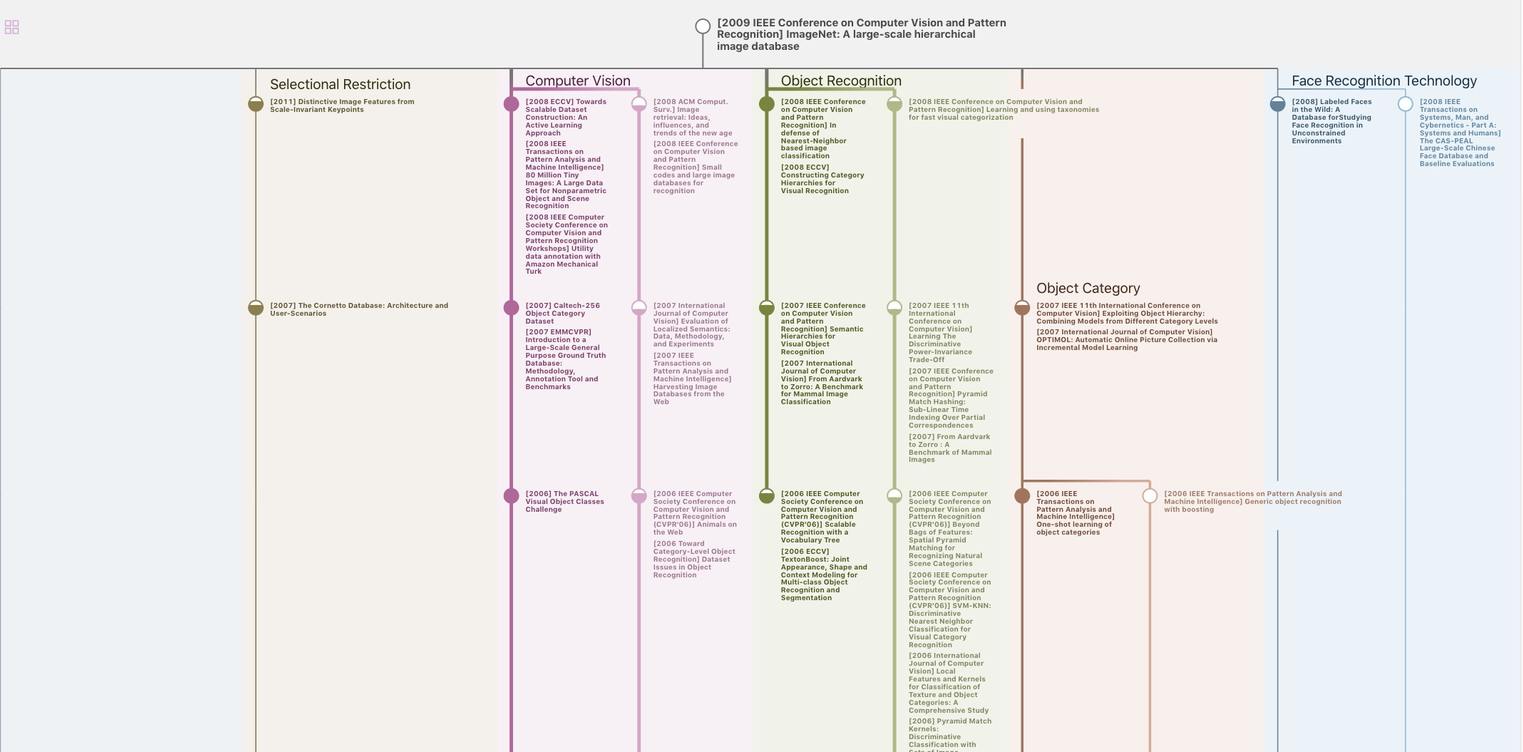
生成溯源树,研究论文发展脉络
Chat Paper
正在生成论文摘要