On Leveraging the Computational Potential of Fog-Enabled Vehicular Networks
Proceedings of the 9th ACM Symposium on Design and Analysis of Intelligent Vehicular Networks and Applications(2019)
摘要
The advent of autonomous vehicles demands powerful processing capabilities of on-board units to handle the dramatic increase of sensor data used to make safe self-driving decisions. Those com- putational resources, being constantly available on the highways, represent valuable assets that can be leveraged to serve as fog computing facility to computational tasks generated from other vehicles or even from different networks. In this paper, we propose a fog-enabled system scheme that can be deployed on a road side unit (RSU) to schedule and offload requested computational tasks over the available vehicles' on-board units (OBUs). The goal is to maximize the weighted sum of the admitted tasks. We model the problem as a Mixed Integer Linear Programming (MILP), and due to NP-hardness, we propose a Dantzig-Wolfe decomposition method to provide a scalable solution. The experiment shows that our ap- proach has a sufficient effectiveness in terms of both computational complexity and tasks acceptance rate.
更多查看译文
关键词
dantzig-wolfe decomposition, fog computing, vehicular networks
AI 理解论文
溯源树
样例
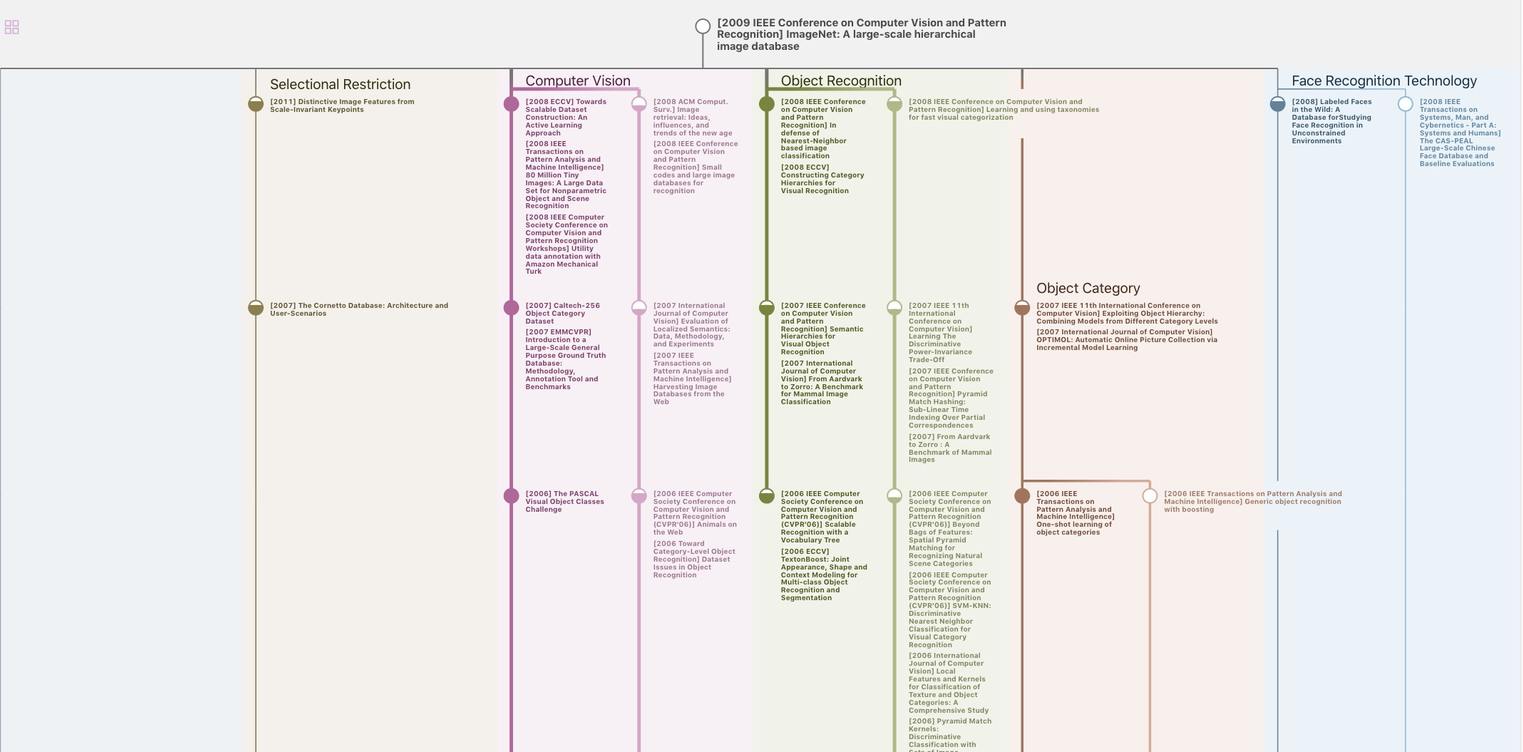
生成溯源树,研究论文发展脉络
Chat Paper
正在生成论文摘要