Quantized Compressed Sensing by Rectified Linear Units
Pamm(2021)
摘要
This work is concerned with the problem of recovering high-dimensional signals $\mathbf{x} \in \mathbb{R}^n$ which belong to a convex set of low-complexity from a small number of quantized measurements. We propose to estimate the signals via a convex program based on rectified linear units (ReLUs) for two different quantization schemes, namely one-bit and uniform multi-bit quantization. Assuming that the linear measurement process can be modelled by a sensing matrix with i.i.d. subgaussian rows, we obtain for both schemes near-optimal uniform reconstruction guarantees by adding well-designed noise to the linear measurements prior to the quantization step. In the one-bit case, we show that the program is robust against adversarial bit corruptions as well as additive noise on the linear measurements. Further, our analysis quantifies precisely how the rate-distortion relationship of the program changes depending on whether we seek reconstruction accuracies above or below the noise floor. The proofs rely on recent results by Dirksen and Mendelson on non-Gaussian hyperplane tessellations. Finally, we complement our theoretical analysis with numerical experiments which compare our method to other state-of-the-art methodologies.
更多查看译文
关键词
Quantization (signal),Noise measurement,Compressed sensing,Q measurement,Sensors,Hamming distance,Additives
AI 理解论文
溯源树
样例
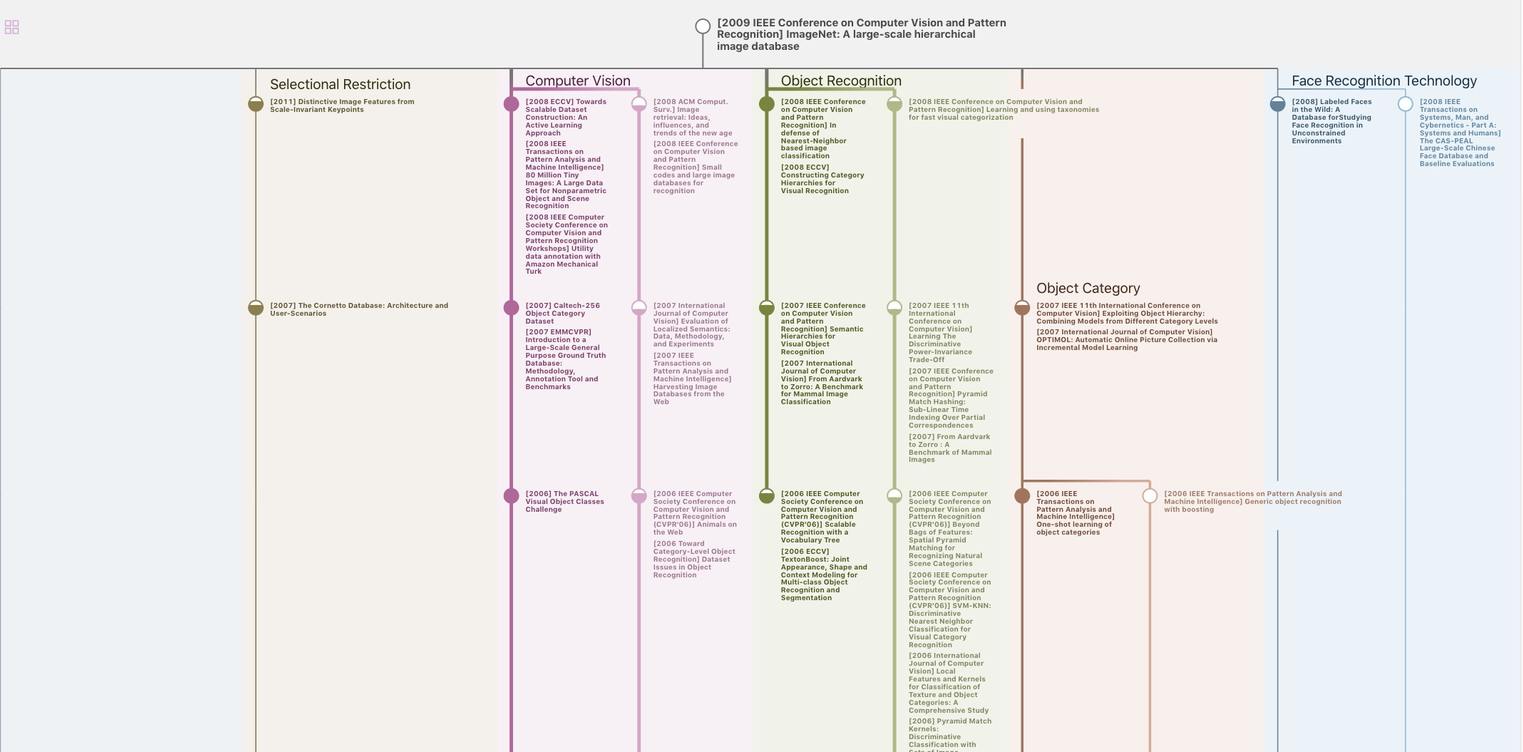
生成溯源树,研究论文发展脉络
Chat Paper
正在生成论文摘要