Pigeon - an Effective Distributed, Hierarchical Datacenter Job Scheduler.
SoCC '19: ACM Symposium on Cloud Computing Santa Cruz CA USA November, 2019(2019)
摘要
In today's datacenters, job heterogeneity makes it difficult for schedulers to simultaneously meet latency requirements and maintain high resource utilization. The state-of-the-art datacenter schedulers, including centralized, distributed, and hybrid schedulers, fail to ensure low latency for short jobs in large-scale and highly loaded systems. The key issues are the scalability in centralized schedulers, ineffective and inefficient probing and resource sharing in both distributed and hybrid schedulers.
In this paper, we propose Pigeon, a distributed, hierarchical job scheduler based on a two-layer design. Pigeon divides workers into groups, each managed by a separate master. In Pigeon, upon a job arrival, a distributed scheduler directly distribute tasks evenly among masters with minimum job processing overhead, hence, preserving highest possible scalability. Meanwhile, each master manages and distributes all the received tasks centrally, oblivious of the job context, allowing for full sharing of the worker pool at the group level to maximize multiplexing gain. To minimize the chance of head-of-line blocking for short jobs and avoid starvation for long jobs, two weighted fair queues are employed in each master to accommodate tasks from short and long jobs, separately, and a small portion of the workers are reserved for short jobs. Evaluation via theoretical analysis, trace-driven simulations, and a prototype implementation shows that Pigeon significantly outperforms Sparrow, a representative distributed scheduler, and Eagle, a hybrid scheduler.
更多查看译文
关键词
Job scheduling, resource management, datacenter
AI 理解论文
溯源树
样例
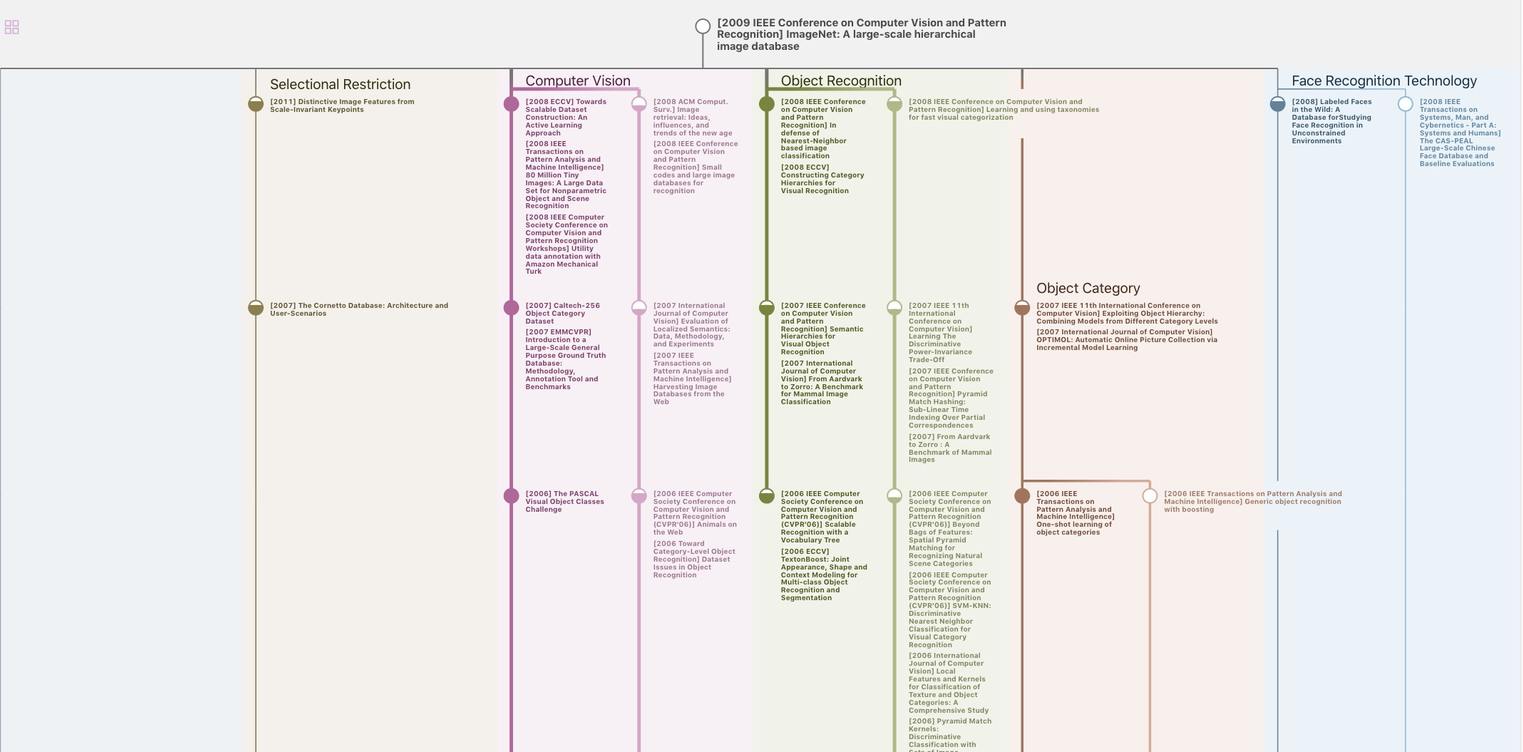
生成溯源树,研究论文发展脉络
Chat Paper
正在生成论文摘要