A parallel approach to calculate lower and upper approximations in dominance based rough set theory.
Applied Soft Computing(2019)
摘要
Feature selection plays an important role in data mining and machine learning tasks. Rough set theory has been a prominent tool for this purpose. It characterizes a dataset by using two important measures called lower and upper approximation. Dominance based rough set approach (DSRA) is an extension to conventional rough set theory. It is based on persistence of preference order while extracting knowledge from datasets. Dominance principal states that objects belonging to a certain decision class should follow the preference order. Preference order states that an object having higher values of conditional attributes should belong to higher decision classes. However, some of the basic concepts like checking preference order consistency of a dataset, dominance based lower approximation and upper approximation are computationally too expensive to be used for large datasets. In this paper, we have proposed a parallel incremental approach called Parallel Incremental Approximation Calculation or PIAC for short, for calculating these measures of lower and upper approximations. The proposed approach incrementally calculates lower and upper approximations using parallel threads. We compare our method with the conventional approach using ten widely used datasets. Whilst achieving the same accuracy levels as the conventional approach, our approach significantly reduces the average computation time, i.e., 71% for the lower approximation and 70% for the upper approximation. Over all datasets, the decrease in memory usage achieved was 99%.
更多查看译文
关键词
Dominance-based rough set approach,Lower approximation,Upper approximation,Incremental approximation calculation
AI 理解论文
溯源树
样例
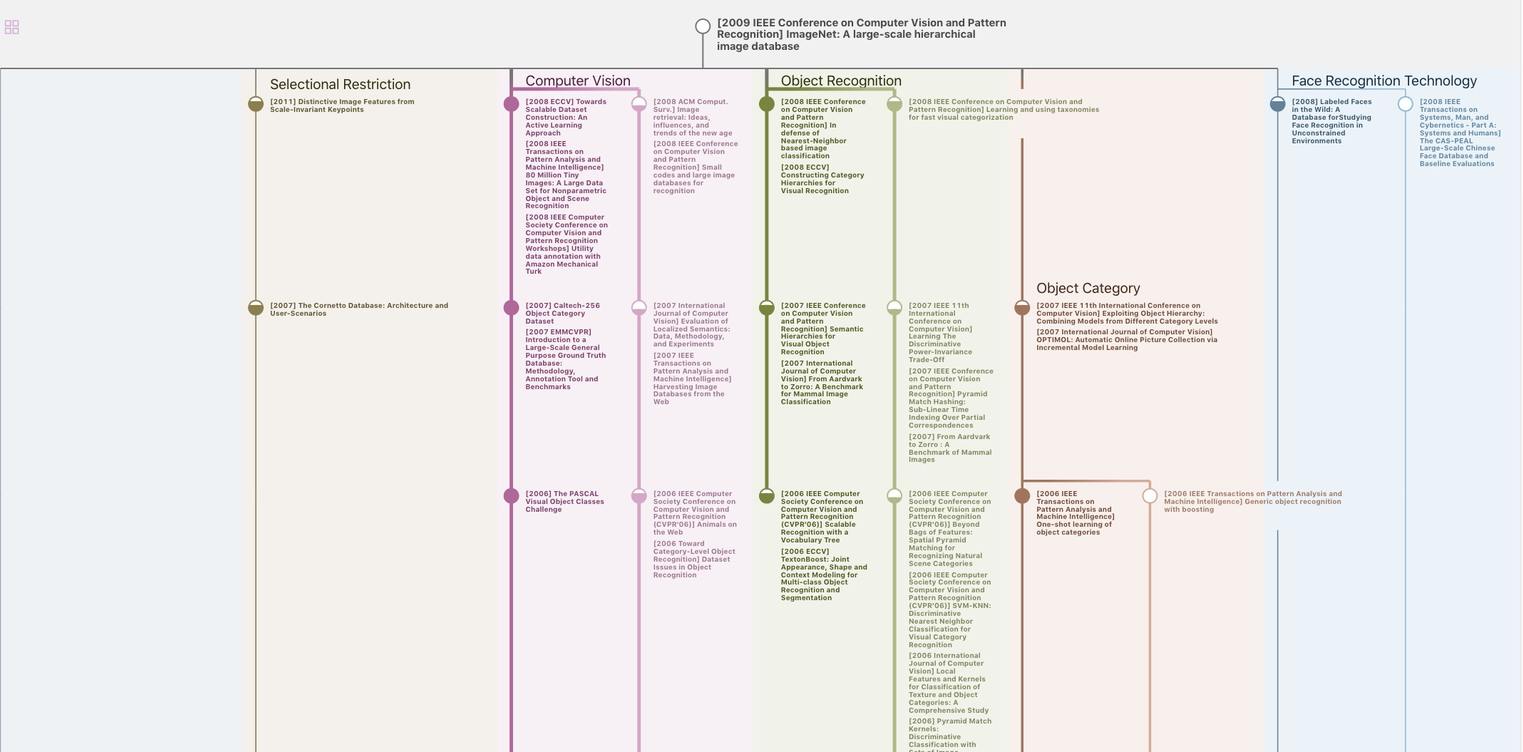
生成溯源树,研究论文发展脉络
Chat Paper
正在生成论文摘要