Automatic Sequential Pattern Mining in Data Streams
Proceedings of the 28th ACM International Conference on Information and Knowledge Management(2019)
摘要
Given a large volume of multi-dimensional data streams, such as that produced by IoT applications, finance and online web-click logs, how can we discover typical patterns and compress them into compact models? In addition, how can we incrementally distinguish multiple patterns while considering the information obtained from a pattern found in a streaming setting? In this paper, we propose a streaming algorithm, namely StreamScope, that is designed to find intuitive patterns efficiently from event streams evolving over time. Our proposed method has the following properties: (a) it is effective: it operates on semi-infinite collections of co-evolving streams and summarizes all the streams into a set of multiple discrete segments grouped by their similarities. (b) it is automatic: it automatically and incrementally recognizes such patterns and generates models for each of them if necessary; (c) it is scalable: the complexity of our method does not depend on the length of the data streams. Our extensive experiments on real data streams demonstrate that StreamScope can find meaningful patterns and achieve great improvements in terms of computational time and memory space over its full batch method competitors.
更多查看译文
关键词
data stream, pattern discovery, time series
AI 理解论文
溯源树
样例
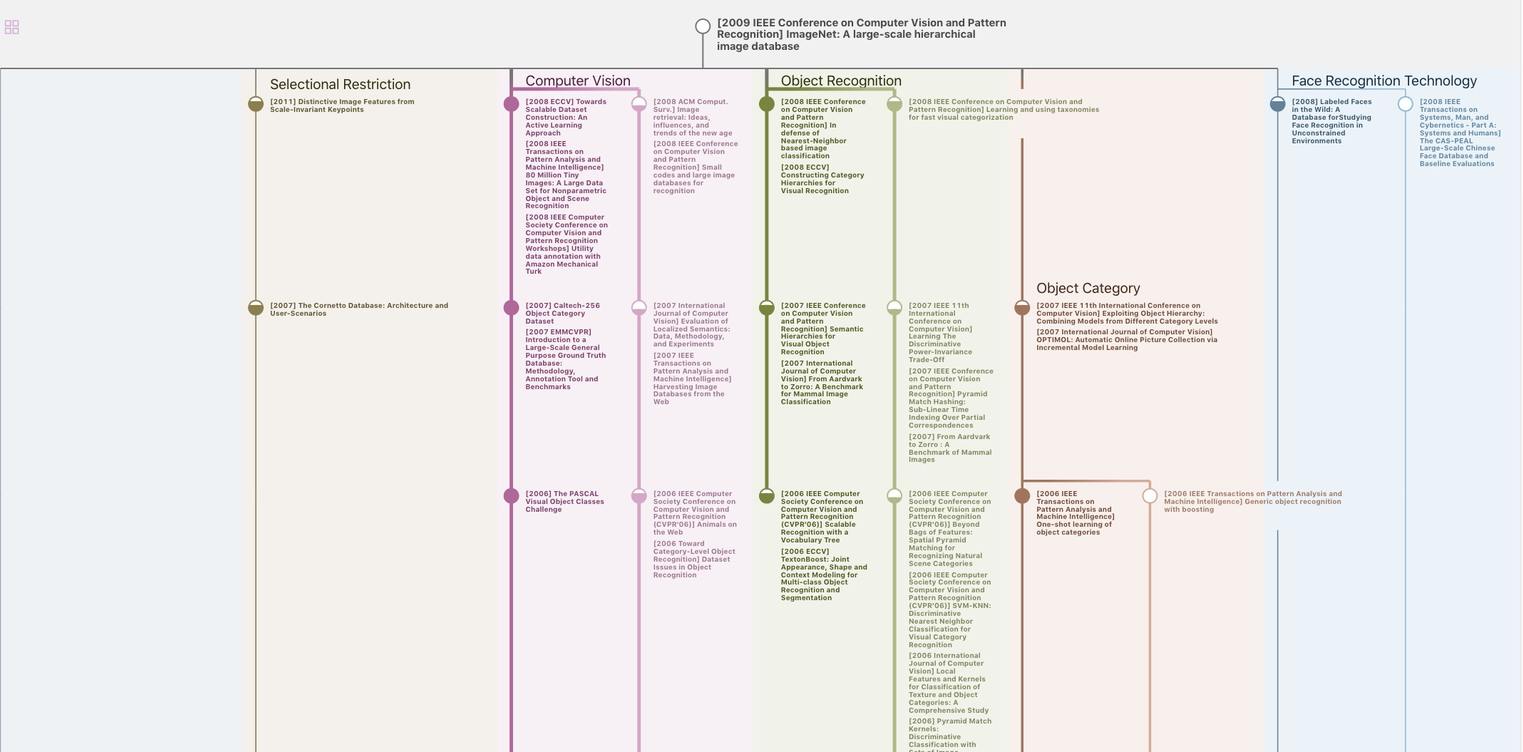
生成溯源树,研究论文发展脉络
Chat Paper
正在生成论文摘要