Local Higher-Order Community Detection Based On Fuzzy Membership Functions
IEEE ACCESS(2019)
摘要
Local community detection, only considering the regional information of the large network, can be used to identify a densely connected community containing the seed node in a network, aiming to address the efficiency problem faced by global community detection. However, most existing studies in local community detection did not account for the higher-order structures crucial to the network, but rather have simply focused single nodes or edges. Moreover, existing higher-order solutions are not purely local methods, as they still use global search to find the best local community, which leads to a global search problem. Furthermore, the quality of the detected community depends on the location of the seed node, which leads to a seed-dependent problem. Thus, in this paper, we proposed a fuzzy agglomerative algorithm (FuzLhocd) for local higher-order community detection based on different fuzzy membership functions. To solve the global search problem, we introduce a novel, purely localized metric called local motif modularity. Based on this local metric, FuzLhocd only needs to visit a limited number of neighborhoods around the seed node. To solve the seed-dependent problem, we systematically studied the formation of the local community, divided the process of local community detection into three stages and employed various fuzzy membership functions at different stages. Our extensive experiments based on both real-world and synthetic networks demonstrated that FuzLhocd not only runs efficiently locally but also effectively solves the seed-dependent problem and achieves a high accuracy as well. We concluded that our local motif modularity metric and FuzLhocd algorithm is highly effective for local higher-order community detection.
更多查看译文
关键词
Local community detection, higher-order structure, motif, fuzzy membership functions
AI 理解论文
溯源树
样例
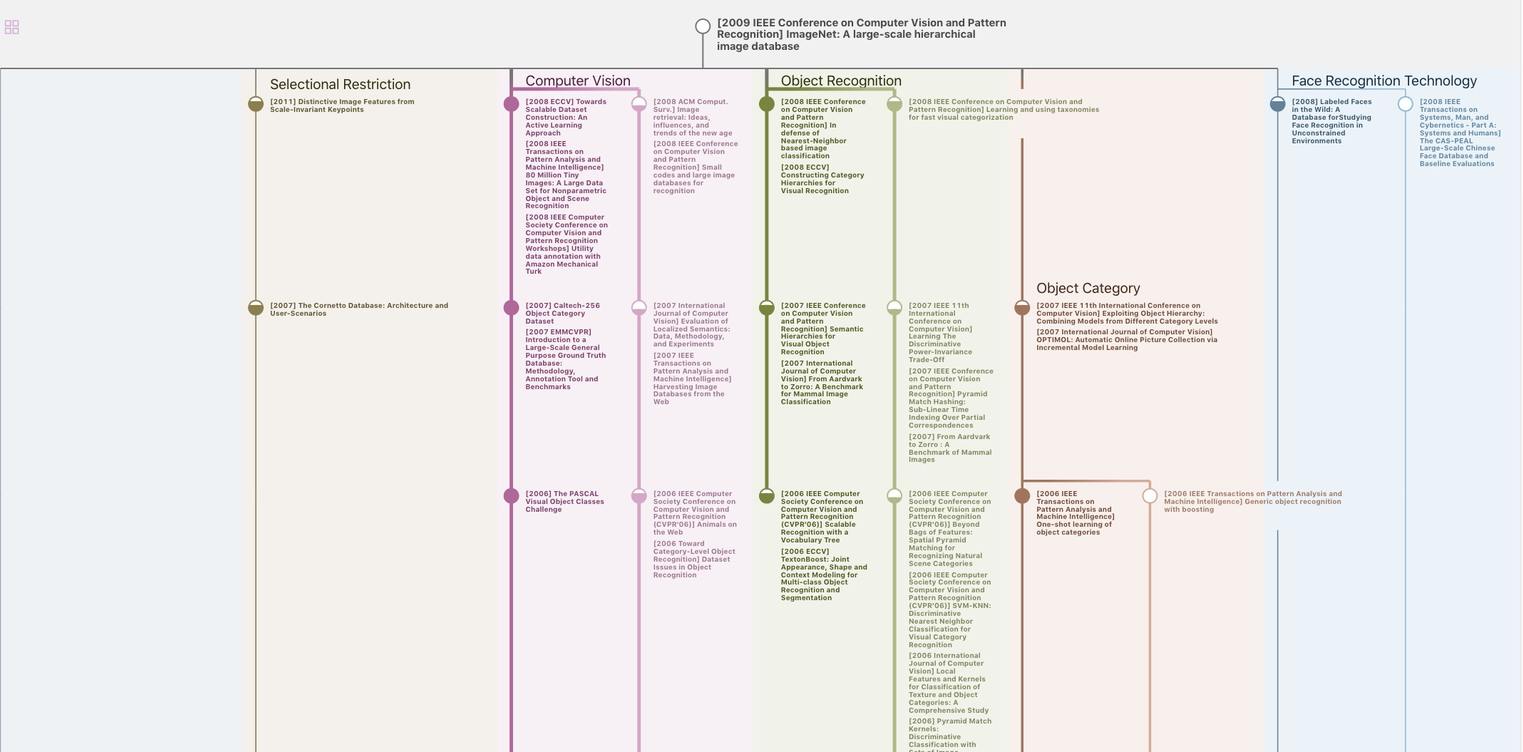
生成溯源树,研究论文发展脉络
Chat Paper
正在生成论文摘要