Perspective on coarse-graining, cognitive load, and materials simulation
Computational Materials Science(2020)
摘要
The predictive capabilities of computational materials science today derive from overlapping advances in simulation tools, modeling techniques, and best practices. We outline this ecosystem of molecular simulations by explaining how important contributions in each of these areas have fed into each other. The combined output of these tools, techniques, and practices is the ability for researchers to advance understanding by efficiently combining simple models with powerful software. As specific examples, we show how the prediction of organic photovoltaic morphologies have improved by orders of magnitude over the last decade, and how the processing of reacting epoxy thermosets can now be investigated with million-particle models. We discuss these two materials systems and the training of materials simulators through the lens of cognitive load theory.
更多查看译文
关键词
Coarse-grained models,Cognitive load,Molecular dynamics,Organic photovoltaics,Epoxy,Thermosets,Teaching,Software,Thermodynamics
AI 理解论文
溯源树
样例
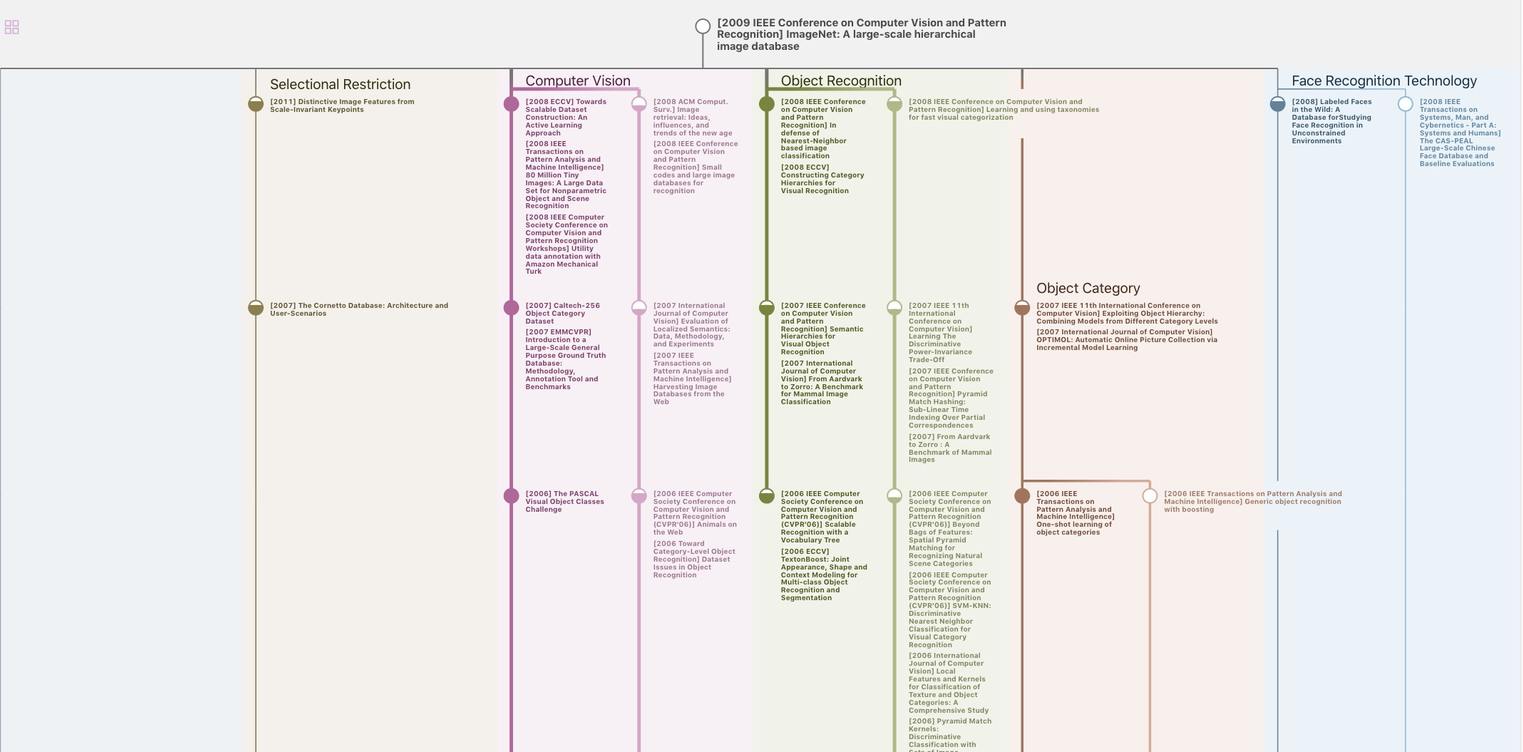
生成溯源树,研究论文发展脉络
Chat Paper
正在生成论文摘要