An Approximate Quadratic Programming For Efficient Bellman Equation Solution
IEEE ACCESS(2019)
摘要
This paper proposes an efficient algorithm which relies on quadratic programming for approximately solving the Bellman equation in reinforcement learning problem and guarantees to return optimal decision parameters. Through further applying universal approximation and fixed cardinality minimization techniques, the proposed algorithm in one hand expands the representation ability of basic linear value functions, on the other hand, it guarantees the convergence of the Bellman error. Experimental results on two canonical reinforcement learning scenarios demonstrate that the proposed algorithm achieves similar or better performance than the state-of-the-art algorithms, while reduces the computation time significantly and improves the robustness of the algorithm against state uncertainty.
更多查看译文
关键词
Markov decision processes, approximate quadratic programming, Bellman equation solutions, universal approximation, fixed cardinality
AI 理解论文
溯源树
样例
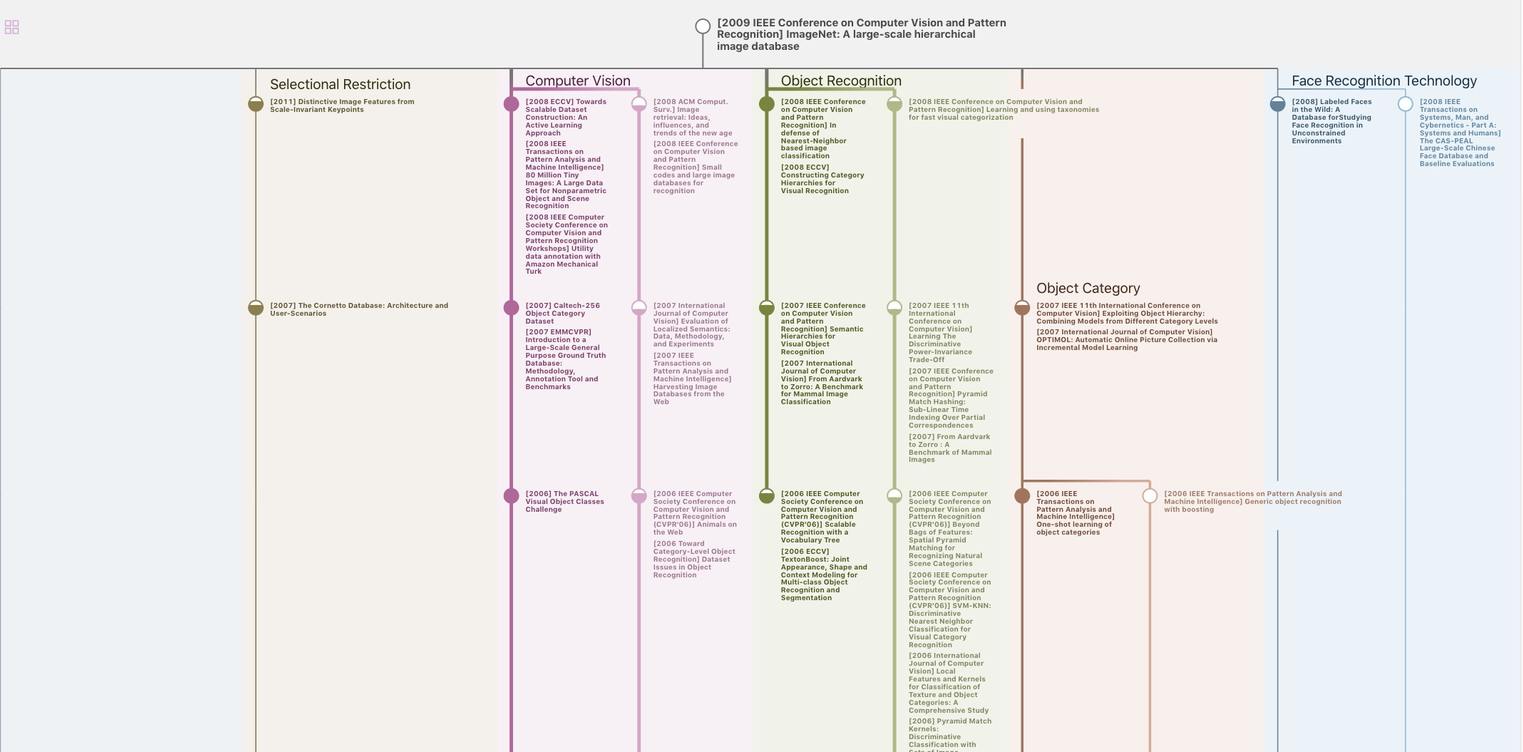
生成溯源树,研究论文发展脉络
Chat Paper
正在生成论文摘要