Detection of sub-surface damage in wind turbine bearings using acoustic emissions and probabilistic modelling
Renewable Energy(2020)
摘要
Bearings are the culprit of a large quantity of Wind Turbine (WT) gearbox failures and account for a high percentage of the total of global WT downtime. Damage within rolling element bearings have been shown to initiate beneath the surface which defies detection by conventional vibration monitoring as the geometry of the rolling surface is unaltered. However, once bearing damage reaches the surface, it generates spalling and quickly drives the deterioration of the entire gearbox through the introduction of debris into the oil system. There is a pressing need for performing damage detection before damage reaches the bearing surface. This paper presents a methodology for detecting sub-surface damage using Acoustic Emission (AE) measurements. AE measurements are well known for their sensitivity to incipient damage. However, the background noise and operational variations within a bearing necessitate the use of a principled statistical procedure for damage detection. This is addressed here through the use of probabilistic modelling, more specifically Gaussian mixture models. The methodology is validated using a full-scale rig of a WT bearing. The bearings are seeded with sub-surface and early-stage surface defects in order to provide a comparison of the detectability at each level of a fault progression.
更多查看译文
关键词
Acoustic Emission,Condition monitoring,Bearings,Damage detection,Probabilistic modelling,Wind turbines
AI 理解论文
溯源树
样例
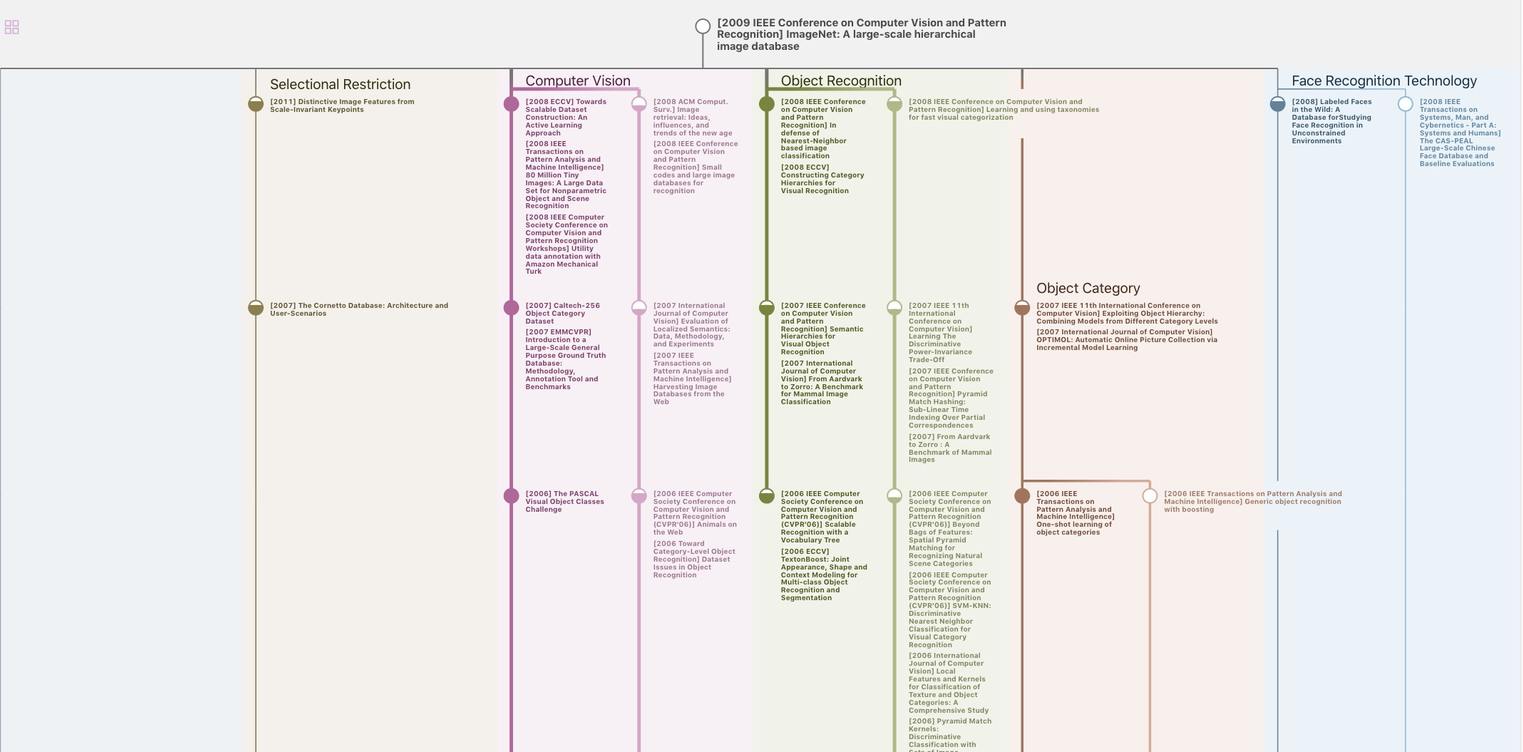
生成溯源树,研究论文发展脉络
Chat Paper
正在生成论文摘要