A dual attention dilated residual network for liver lesion classification and localization on CT images
international conference on image processing(2019)
摘要
Automatic liver lesion classification on computed tomography images is of great importance to early cancer diagnosis and remains a challenging task. State-of-the-art liver lesion classification algorithms are currently based on manually selected regions of interest (ROIs) or automatically detected ROIs. However, liver lesions usually vary in size and shape, which makes the ROI selection process labor-intensive and also poses an obstacle to automatic lesion detection. In this paper, we propose a dual-attention dilated residual network (DADRN) as a potential solution to lesion classification task without manual ROI selection or automatic lesion detection. We incorporated a novel dual-attention module in order to capture the non-local feature dependencies and help the deep neural network focus on the lesion area by enlarging the difference between the lesion area and nonlesion area. To the best of our knowledge, we are the first to employ the self-attention mechanism to address liver lesion classification task. In addition, the well-trained DADRN can be used for weakly-supervised lesion localization without any architectural change or retraining. Experiment results show that DADRN could achieve a lesion classification accuracy comparable to that of the state-of-the-art ROI-based method and outperformed state-of-the-art attention-based approaches in both liver lesion classification and localization tasks.
更多查看译文
关键词
Dual-attention,dilated residual network,lesion classification,weakly-supervised localization
AI 理解论文
溯源树
样例
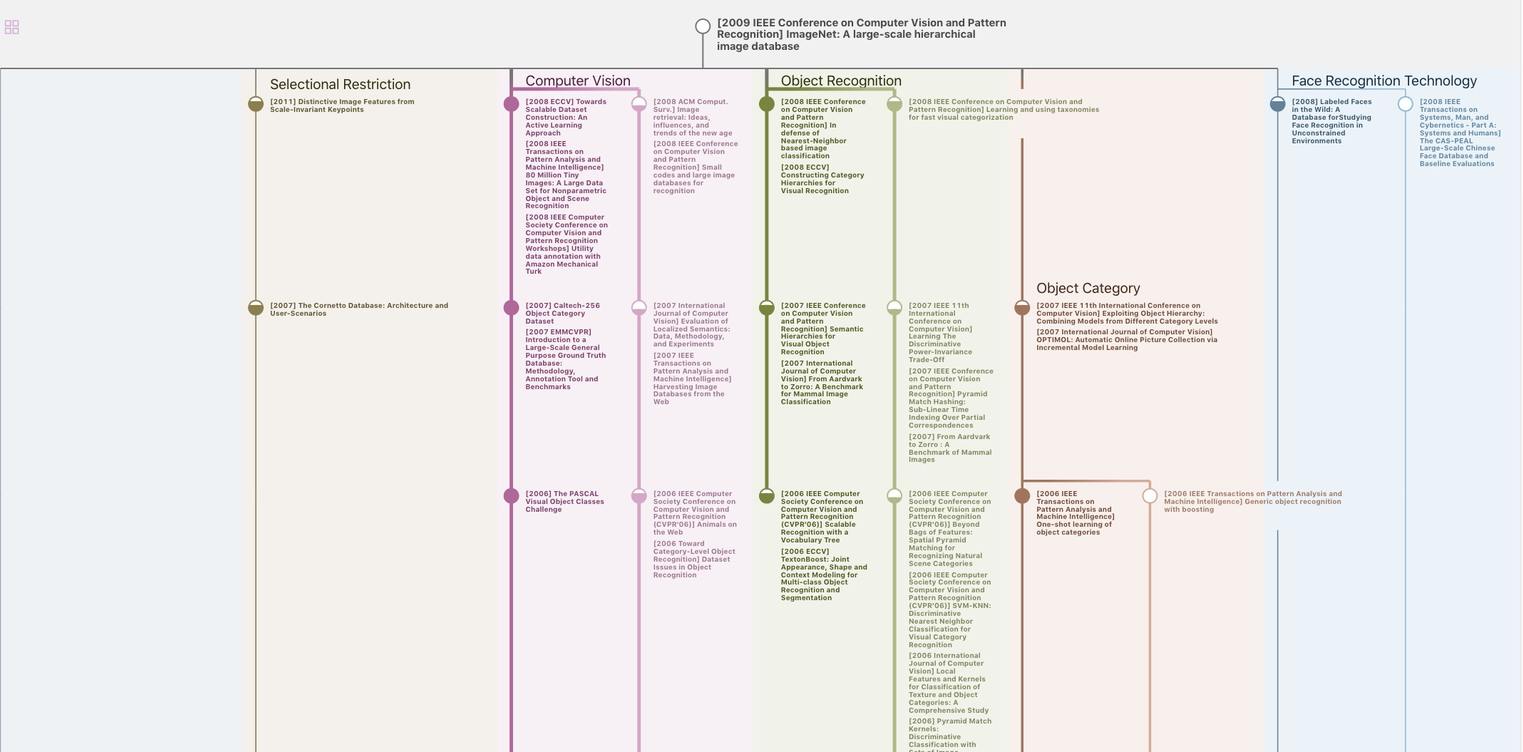
生成溯源树,研究论文发展脉络
Chat Paper
正在生成论文摘要