Efficient Training Techniques For Multi-Agent Reinforcement Learning In Combat Tasks
IEEE ACCESS(2019)
摘要
Multi-agent combat scenarios often appear in many real-time strategy games. Efficient learning for such scenarios is an indispensable step towards general artificial intelligence. Multi-agent reinforcement learning (MARL) algorithms have attracted much interests, but few of them have been shown effective for such scenarios. Most of previous research is focused on revising the learning mechanism of MARL algorithms, for example, trying different types of neural networks. The study of training techniques for improving the performance of MARL algorithms has not been paid much attention. In this paper we propose three efficient training techniques for a multi-agent combat problem which is originated from an unmanned aerial vehicle (UAV) combat scenario. The first one is the scenario-transfer training, which utilizes the experience obtained in simpler combat tasks to assist the training for complex tasks. The next one is the self-play training, which can continuously improve the performance by iteratively training agents and their counterparts. Finally, we consider using combat rules to assist the training, which is named as the rule-coupled training. We combine the three training techniques with two popular multi-agent reinforcement learning methods, multi-agent deep q-learning and multi-agent deep deterministic policy gradient (proposed by Open AI in 2017), respectively. The results show that both the converging speed and the performance of the two methods are significantly improved through the three training techniques.
更多查看译文
关键词
Scenario-transfer training, self-play training, rule-coupled training
AI 理解论文
溯源树
样例
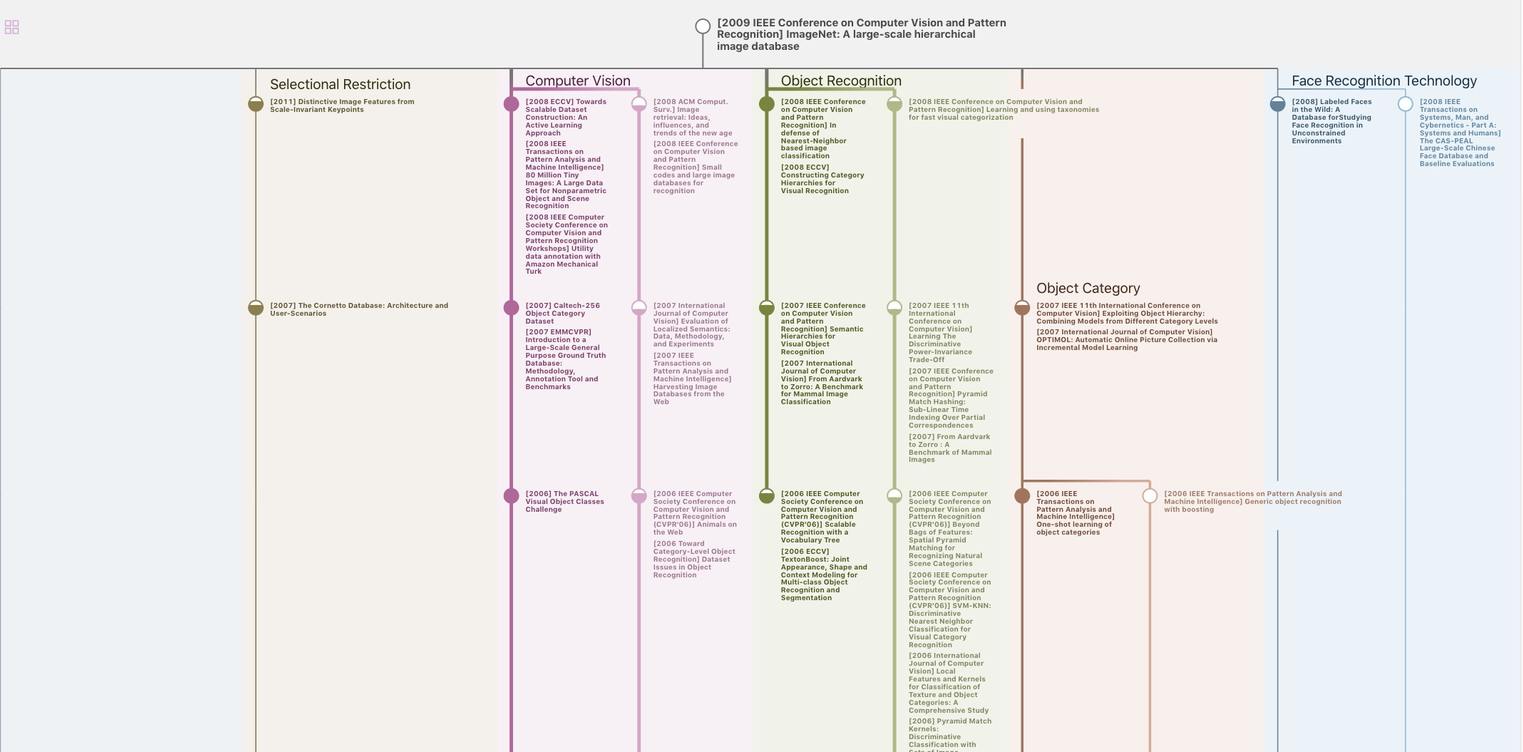
生成溯源树,研究论文发展脉络
Chat Paper
正在生成论文摘要