Leveraging Independent Double-Gate FinFET Devices for Machine Learning Classification
IEEE Transactions on Circuits and Systems I: Regular Papers(2019)
摘要
Mixed-signal machine learning (ML) classification has recently been demonstrated as an efficient alternative for classification with power expensive digital MOSFET circuits. In this paper, a topology based on independent double-gate (IDG) FinFET devices is proposed for classifying high-dimensional input data into multi-class output space with less power and area as compared with state-of-the-art MOSFET classifiers. To facilitate a high-resolution multiplication as a part of the classifier predictions, ML features and feature weights are, respectively, fed to the back and front gate inputs of the individual IDG-FinFET transistors. A classifier that considers the decisions of the individual predictors is designed at 30 nm FinFET technology node and operates at 333 MHZ with nominal supply voltage of one volt. To evaluate the performance of the classifier, a reduced MNIST dataset is generated. The original resolution is reduced from
$28 \times 28$
features to
$9 \times 9$
features and the most important features are selected with sequential backward selection algorithm. The system is simulated in SPICE, exhibiting prediction accuracy of 90%, energy consumption of 25 pJ per classification (over four times lower than similar state-of-the-art classifiers), area of 1,365
${\mu }\text {m}^{2}$
, and a stable response under a wide range of system variations.
更多查看译文
关键词
Logic gates,FinFETs,Integrated circuits,Power demand,SPICE,Sensors
AI 理解论文
溯源树
样例
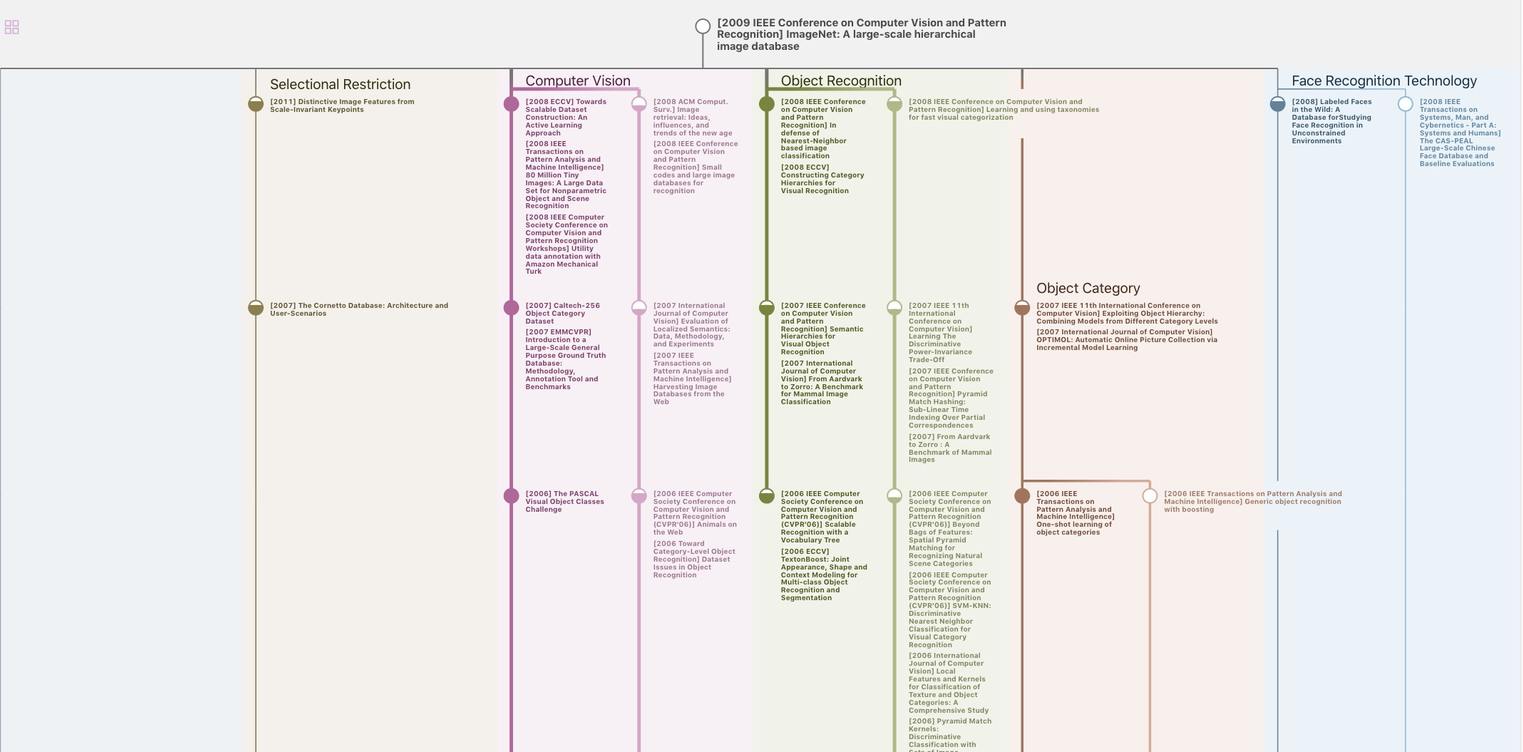
生成溯源树,研究论文发展脉络
Chat Paper
正在生成论文摘要