Multi-task Learning for Neonatal Brain Segmentation Using 3D Dense-Unet with Dense Attention Guided by Geodesic Distance
Domain Adaptation and Representation Transfer and Medical Image Learning with Less Labels and Imperfect Data : first MICCAI Workshop, DART 2019, and first International Workshop, MIL3ID 2019, Shenzhen, held in conjunction with MICCAI 20...(2019)
摘要
The deep convolutional neural network has achieved outstanding performance on neonatal brain MRI tissue segmentation. However, it may fail to produce reasonable results on unseen datasets that have different imaging appearance distributions with the training data. The main reason is that deep learning models tend to have a good fitting to the training dataset, but do not lead to a good generalization on the unseen datasets. To address this problem, we propose a multi-task learning method, which simultaneously learns both tissue segmentation and geodesic distance regression to regularize a shared encoder network. Furthermore, a dense attention gate is explored to force the network to learn rich contextual information. By using three neonatal brain datasets with different imaging protocols from different scanners, our experimental results demonstrate superior performance of our proposed method over the existing deep learning-based methods on the unseen datasets.
更多查看译文
关键词
Neonatal brain segmentation,Multi-task learning,Attention,Geodesic distance
AI 理解论文
溯源树
样例
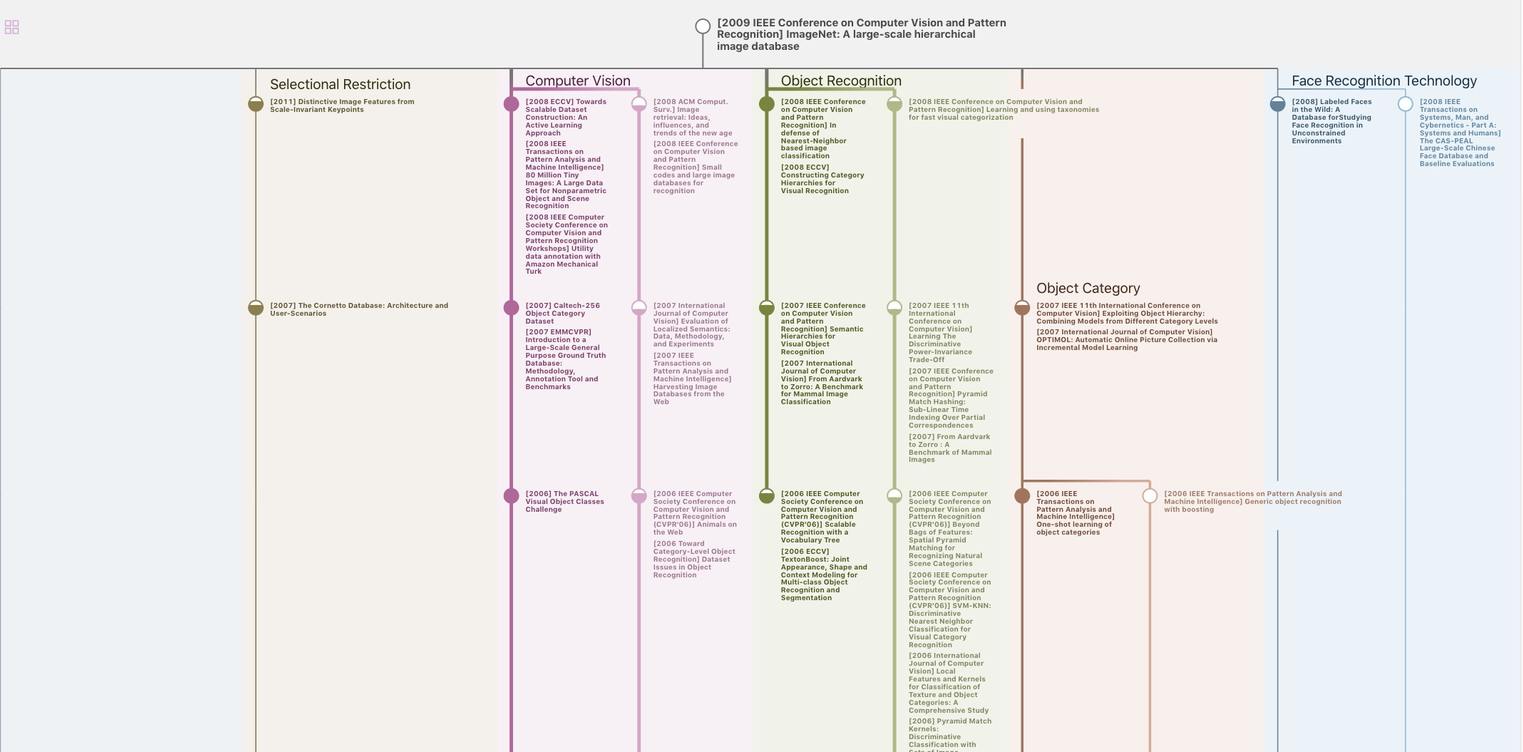
生成溯源树,研究论文发展脉络
Chat Paper
正在生成论文摘要