LSTM-assisted evolutionary self-expressive subspace clustering
International Journal of Machine Learning and Cybernetics(2021)
摘要
Massive volumes of high-dimensional data that evolve over time are continuously collected by contemporary information processing systems, which bring up the problem of organizing these data into clusters, i.e. achieving the purpose of dimensional reduction, and meanwhile learning their temporal evolution patterns. In this paper, a framework for evolutionary subspace clustering, referred to as LSTM–ESCM, is introduced, which aims at clustering a set of evolving high-dimensional data points that lie in a union of low-dimensional evolving subspaces. In order to obtain the parsimonious data representation at each time step, we propose to exploit the so-called self-expressive trait of the data at each time point. At the same time, LSTM networks are implemented to extract the inherited temporal patterns behind data in the overall time frame. An efficient algorithm has been proposed. Numerous experiments are carried out on real-world datasets to demonstrate the effectiveness of our proposed approach. The results show that the suggested algorithm dramatically outperforms other known similar approaches in terms of both run time and accuracy.
更多查看译文
关键词
Subspace clustering,Evolutionary clustering,Self-expressive models,Motion segmentation,LSTM,Deep learning,Temporal data
AI 理解论文
溯源树
样例
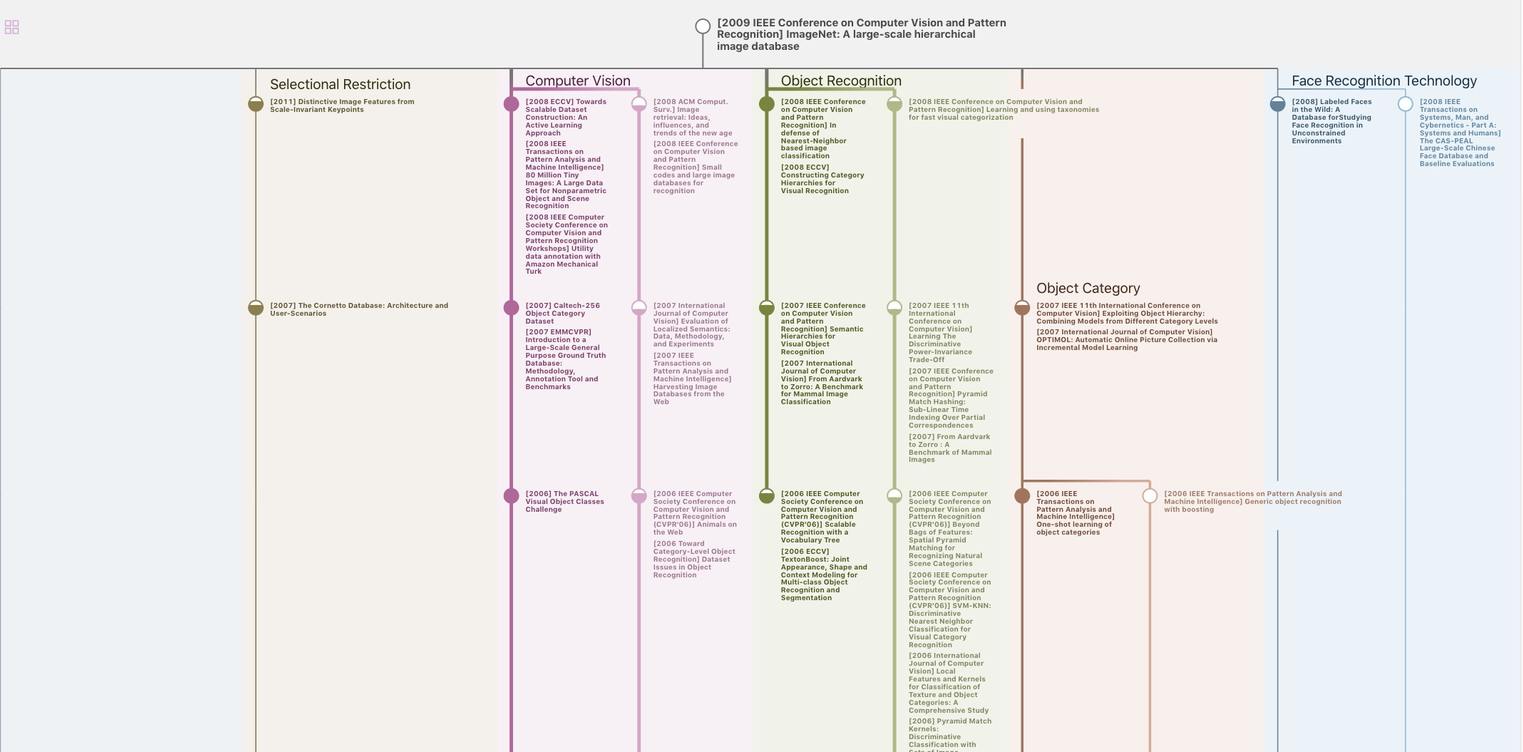
生成溯源树,研究论文发展脉络
Chat Paper
正在生成论文摘要