Progressive Infant Brain Connectivity Evolution Prediction from Neonatal MRI Using Bidirectionally Supervised Sample Selection.
PRIME@MICCAI(2019)
摘要
The early postnatal developmental period is highly dynamic, where brain connections undergo both growth and pruning processes. Understanding typical brain connectivity evolution would enable us to spot abnormal connectional development patterns. However, this generally requires the acquisition of longitudinal neuroimaging datasets that densely cover the first years of postnatal development. This might not be easily investigated since neonatal follow-up scans are rarely acquired in a clinical setting. Furthermore, waiting for the acquisition of later brain scans would hinder early neurodevelopmental disorder diagnosis. To solve this problem, we unprecedentedly propose a bidirectionally supervised sample selection framework, while leveraging the timedependency between consecutive observations, for predicting neonatal brain connectome evolution from a single structural magnetic resonance imaging (MRI) acquired around birth. Specifically, we propose to learn how to select the best training samples by supervisedly training a bidirectional ensemble of regressors from the space of pairwise neonatal connectome disparities to their expected prediction scores resulting from using one training connectome to predict another training connectome. The proposed supervised ensemble learning is time-dependent and has a recall memory anchored at the ground truth baseline observation, allowing to progressively pass over previous predictions through the connectome evolution trajectory. We then rank training samples at current timepoint t(i-1) based on their expected prediction scores by the ensemble and average their connectomes at follow-up timepoint t(i) to predict the testing connectome at t(i). Our framework significantly outperformed comparison methods in leave-one-out cross-validation.
更多查看译文
AI 理解论文
溯源树
样例
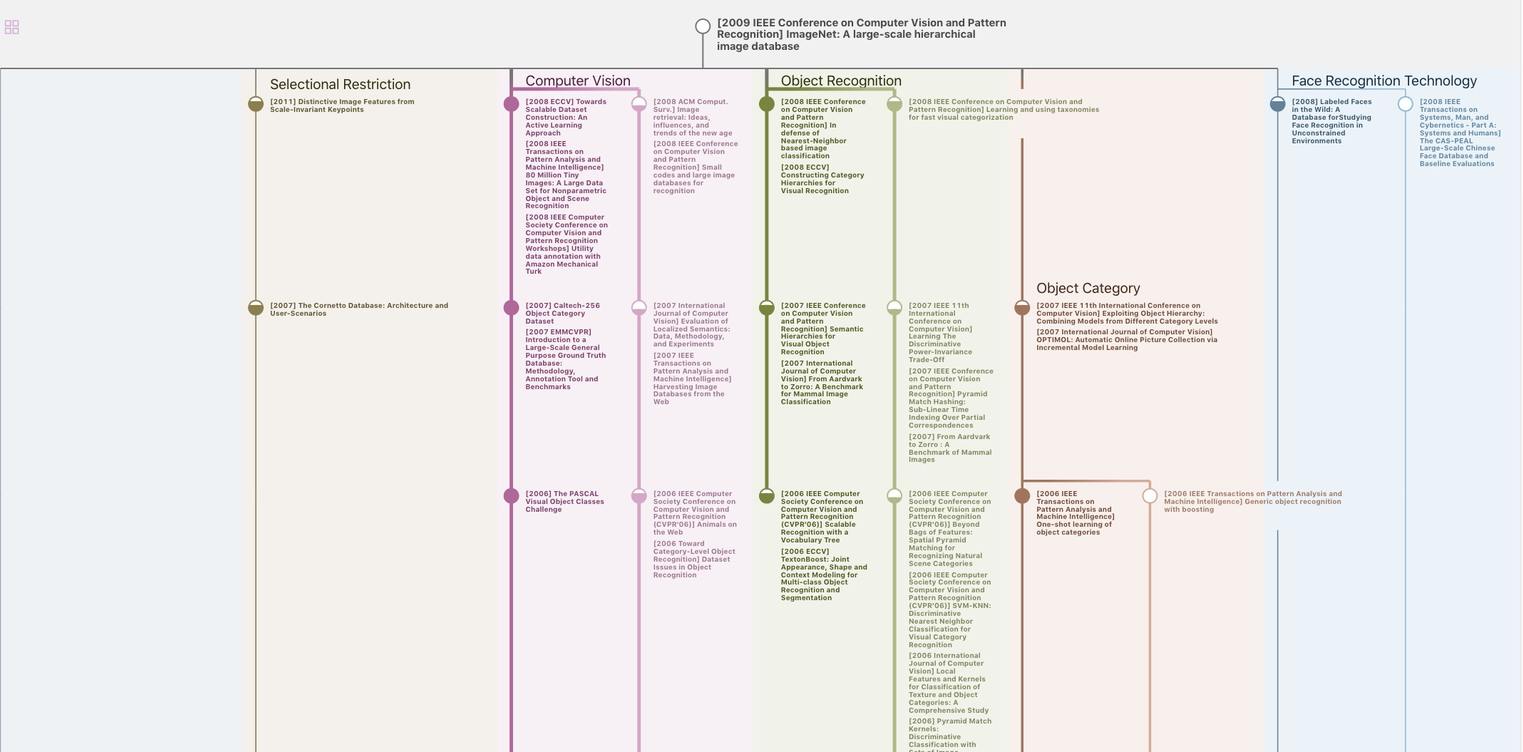
生成溯源树,研究论文发展脉络
Chat Paper
正在生成论文摘要