Tree-LSTM - Using LSTM to Encode Memory in Anatomical Tree Prediction from 3D Images.
Lecture Notes in Computer Science(2019)
摘要
Extraction and analysis of anatomical trees, such as vasculatures and airways, is important for many clinical applications. However, most tracking methods so far intrinsically embedded a first-order Markovian property, where no memory beyond one tracking step was utilized in the tree extraction process. Motivated by the inherent sequential construction of anatomical trees, vis-a-vis the flow of nutrients through branches and bifurcations, we propose Tree-LSTM, the first LSTM neural network to learn to encode such sequential priors into a deep learning based tree extraction method. We also show that mathematically, by using LSTM, the variational lower bound of a higher order Markovian stochastic process could be approximated, which enables the encoding of a long term memory. Our experiments on a CT airway dataset show that, by adding the LSTM component, the results are improved by at least 11% in mean direction prediction accuracy relative to state-of-the-art, and the correlation between bifurcation classification accuracy and evidence is improved by at least 15%, which demonstrate the advantage of a unified deep model for sequential tree structure tracking and bifurcation detection.
更多查看译文
AI 理解论文
溯源树
样例
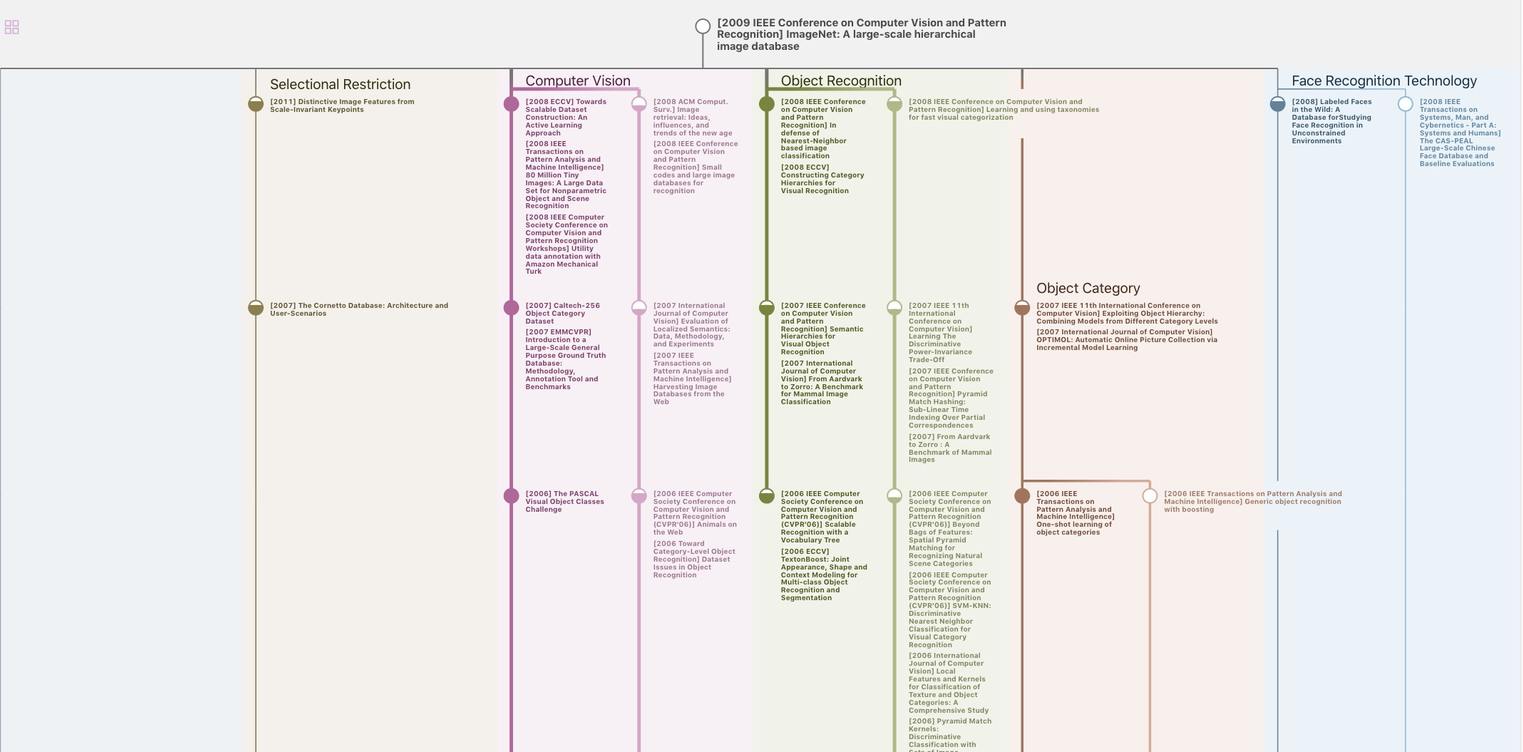
生成溯源树,研究论文发展脉络
Chat Paper
正在生成论文摘要