Individualized 3D Dose Distribution Prediction Using Deep Learning.
AIRT@MICCAI(2019)
摘要
In cancer radiotherapy, inverse treatment planning is a multi-objective optimization problem. There exists a set of plans with various trade-offs on Pareto surface which are referred as Pareto optimal plans. Currently exploring such trade-offs, i.e., physician preference is a trial and error process and often time-consuming. Therefore, it is desirable to predict desired Pareto optimal plans in an efficient way before treatment planning. The predicted plans can be used as references for dosimetrists to rapidly achieve a clinically acceptable plan. Clinically the dose volume histogram (DVH) is a useful tool that can visually indicate the specific dose received by each certain volume percentage which is supposed to describe different trade-offs. Consequently, we have proposed a deep learning method based on patient’s anatomy and DVH information to predict the individualized 3D dose distribution. Qualitative measurements have showed analogous dose distributions and DVH curves compared to the true dose distribution. Quantitative measurements have demonstrated that our model can precisely predict the dose distribution with various trade-offs for different patients, with the largest mean and max dose differences between true dose and predicted dose for all critical structures no more than 1.7% of the prescription dose.
更多查看译文
关键词
Deep learning, Treatment planning, Trade-offs, Dose prediction
AI 理解论文
溯源树
样例
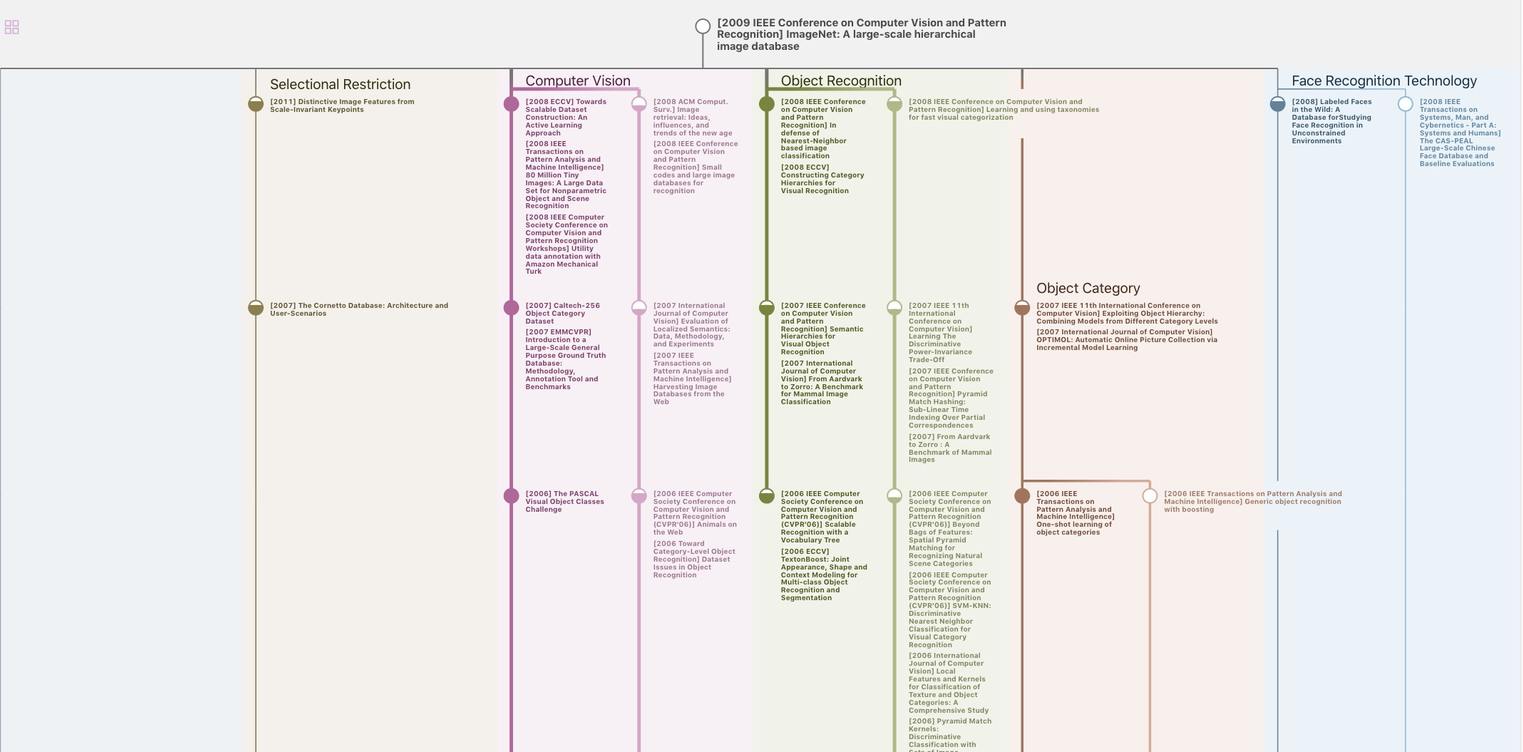
生成溯源树,研究论文发展脉络
Chat Paper
正在生成论文摘要