Unsupervised Domain Adaptation to Improve Image Segmentation Quality Both in the Source and Target Domain
IEEE Computer Society Conference on Computer Vision and Pattern Recognition Workshops(2019)
摘要
Domain adaptation is becoming more and more important with the advancing development of machine learning and the ever-increasing diversity of available data. The advancement of autonomous driving depends very much on progress in machine learning, which relies heavily on vast amounts of training data. It is well known that the performance of such models drops, as soon as the data used during inference stems from a different domain as the training data. To avoid the need to label a separate dataset for each new domain, e.g., each new camera sensor, methods for domain adaptation are necessary. Most interesting are unsupervised domain adaptation approaches since they do not require costly labels for the target domain. In this paper we adapt a known domain adaptation approach to work in an unsupervised fashion for semantic segmentation on high resolution data and provide some analysis of the learned representations. With our domain-adapted semantic segmentation we were able to achieve a significant 15 % absolute increase in mean intersection over union (mIoU), securing a surprisingly good 5th rank on the target domain Kill I test set without having used any Kill I labels during training. In addition to that, we even improved quality on the source domain data.
更多查看译文
关键词
machine learning,training data,unsupervised domain adaptation approaches,target domain,known domain adaptation approach,high resolution data,domain-adapted semantic segmentation,source domain data,image segmentation quality
AI 理解论文
溯源树
样例
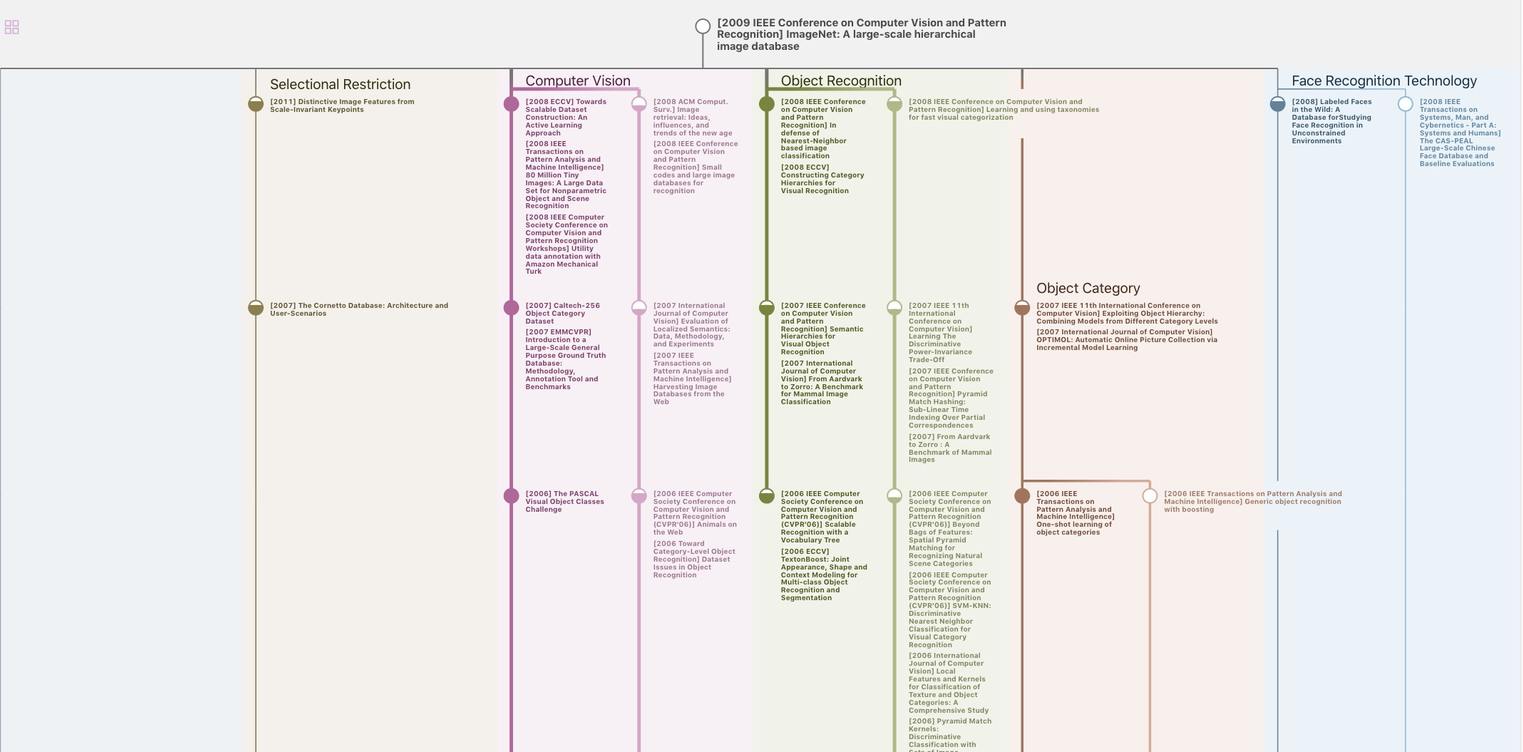
生成溯源树,研究论文发展脉络
Chat Paper
正在生成论文摘要