A gradient boosting decision tree approach for insider trading identification: An empirical model evaluation of China stock market.
Applied Soft Computing(2019)
摘要
Insider trading is a kind of criminal behavior in stock market by using nonpublic information. In recent years, it has become the major illegal activity in China’s stock market. In this study, a combination approach of GBDT (Gradient Boosting Decision Tree) and DE (Differential Evolution) is proposed to identify insider trading activities by using data of relevant indicators. First, insider trading samples occurred from year 2007 to 2017 and corresponding non-insider trading samples are collected. Next, the proposed method is trained by the GBDT, and initial parameters of the GBDT are optimized by the DE. Finally, out-of-samples are classified by the trained GBDT–DE model and its performances are evaluated. The experiment results show that our proposed method performed the best for insider trading identification under time window length of ninety days, indicating the relevant indicators under 90-days time window length are relatively more useful. Additionally, under all three time window lengths, relative importance result shows that several indicators are consistently crucial for insider trading identification. Furthermore, the proposed approach significantly outperforms other benchmark methods, demonstrating that it could be applied as an intelligent system to improve identification accuracy and efficiency for insider trading regulation in China stock market.
更多查看译文
关键词
Stock market,Insider trading,Gradient boosting decision tree,Differential evolution,Identification system
AI 理解论文
溯源树
样例
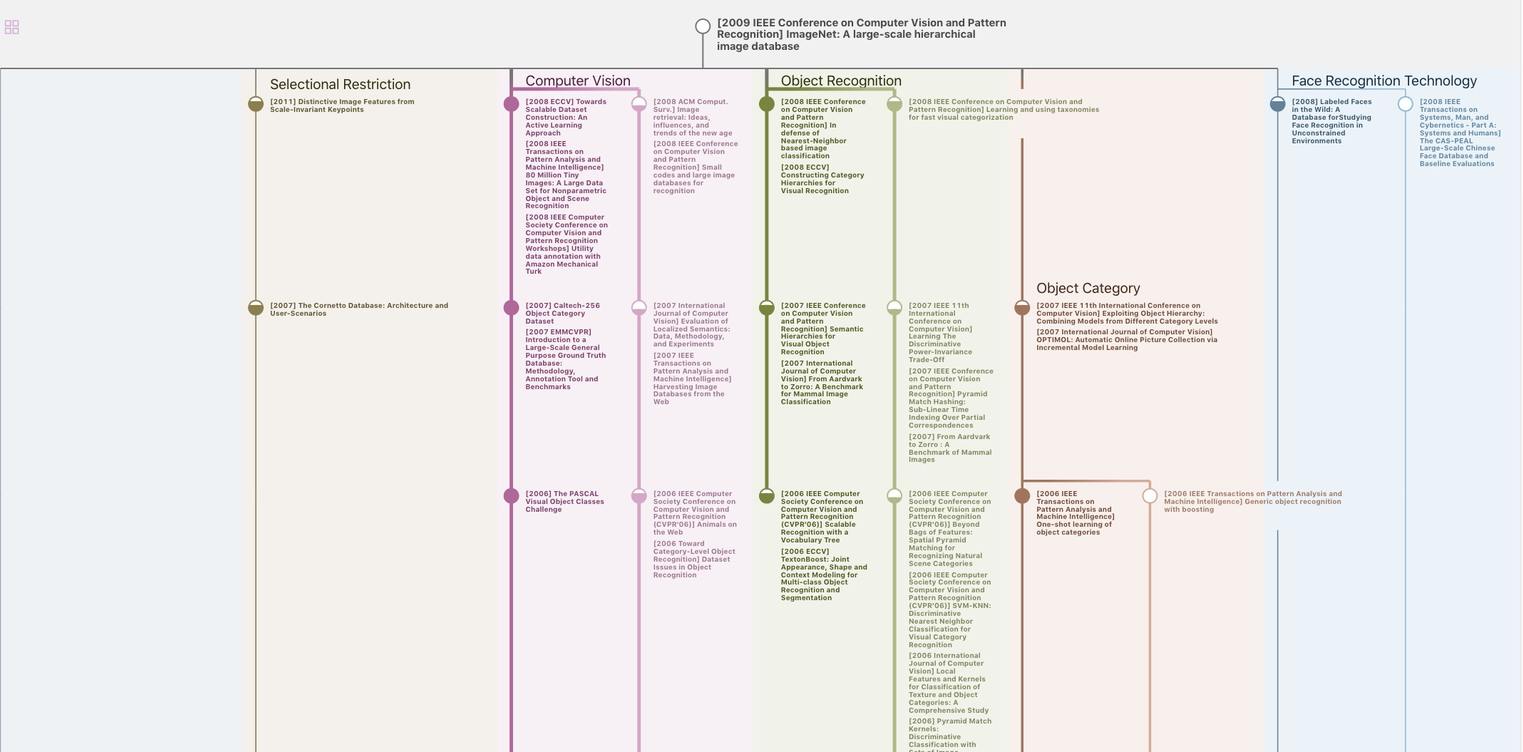
生成溯源树,研究论文发展脉络
Chat Paper
正在生成论文摘要