1.2 Watt Classification of 3D Voxel Based Point-clouds Using a CNN on a Neural Compute Stick
Neurocomputing(2020)
摘要
With the recent surge in popularity of Convolutional Neural Networks (CNNs), motivated by their significant performance in many classification and related tasks, a new challenge now needs to be addressed: how to accommodate CNNs in mobile devices, such as drones, smartphones, and similar low-power devices? In order to tackle this challenge we exploit the Vision Processing Unit (VPU) that combines dedicated CNN hardware blocks and very low power requirement. The lack of readily available training data and memory requirements are two of the factors hindering the training and accuracy performance of 3D CNNs. In this paper, we propose a method for generating synthetic 3D point-clouds from realistic CAD scene models to enrich the training process for volumetric CNNs. Furthermore, an efficient 3D volumetric object representation Volumetric Accelerator format (VOLA) is employed. VOLA is a sexaquaternary (power-of-four subdivision) tree-based representation which allows for significant memory saving for volumetric data. Multiple CNN models were trained and pruning techniques for the weights were applied to the trained 3D Volumetric Network in order to remove almost 70% of the parameters and outperform the existing state-of-the-art networks. The top performing and efficient model was ported to the Movidius™Neural Compute Stick (NCS). After deployment on the NCS, it takes 11 ms ( ∼ 90 frames per second) to perform inference on each input volume, with a reported power requirement of 1.2 W, which leads to 75.75 inferences per second per Watt.
更多查看译文
关键词
3D Convolutional Neural Network,3D object classification,Embedded systems,Low-power
AI 理解论文
溯源树
样例
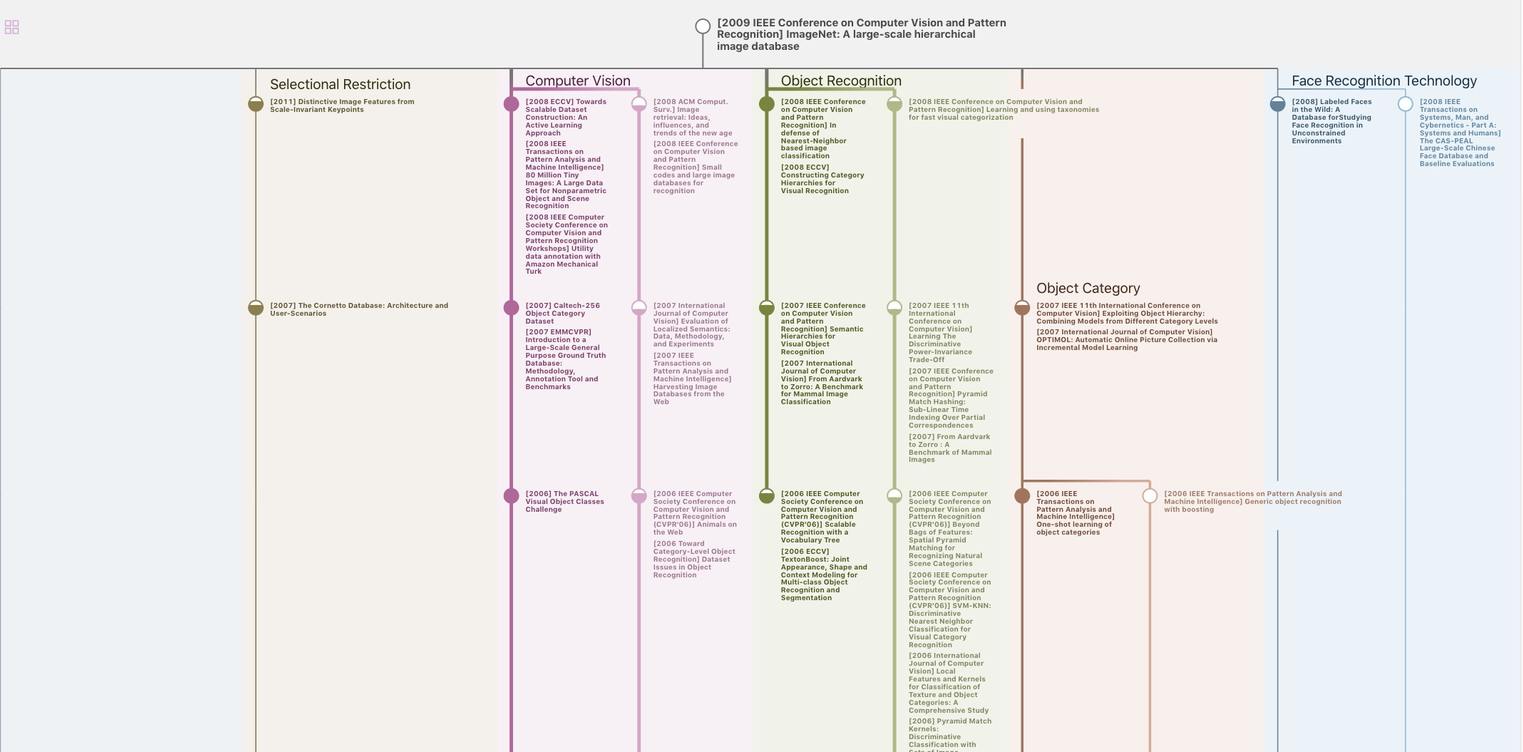
生成溯源树,研究论文发展脉络
Chat Paper
正在生成论文摘要