Decomposition of Overlapping Signals with Multivariate Sparse Learning
Circuits, Systems, and Signal Processing(2019)
摘要
Multivariate kernel sparse learning models have attracted wide attention due to their efficient decomposition of nonlinear feature structures in the time–frequency space. Nevertheless, problems involving multi-modal signal sources have become more common such that the kernel weights selected by these methods are not strictly the sparsest possible solution. This paper presents a novel multivariate sparse learning model that focuses on the decomposition of multicomponent multivariate signals that partially and arbitrarily overlap. The multivariate signal components can be discriminatively reconstructed based on their representation in the kernel space. The model is based on the multivariate extension of the chirplet transform: the time–frequency distribution that can achieve good accuracy when estimating the instantaneous frequency of the overlapping signal components. To improve the decomposition accuracy, discriminative multilayer sparse learning is introduced to this scheme in both the training and testing frameworks in algorithm design. The discriminative structures of the dictionary maximize the weighted difference between the feature variance and the noise variance. The effectiveness of the proposed algorithm is confirmed by the results from decomposing several multicomponent signals that overlap in the time–frequency domain.
更多查看译文
关键词
Multivariate sparse learning (MSL), Multivariate chirplet transform (MCT), Overlapping signals, Multivariate signals decomposition, Time–frequency distribution
AI 理解论文
溯源树
样例
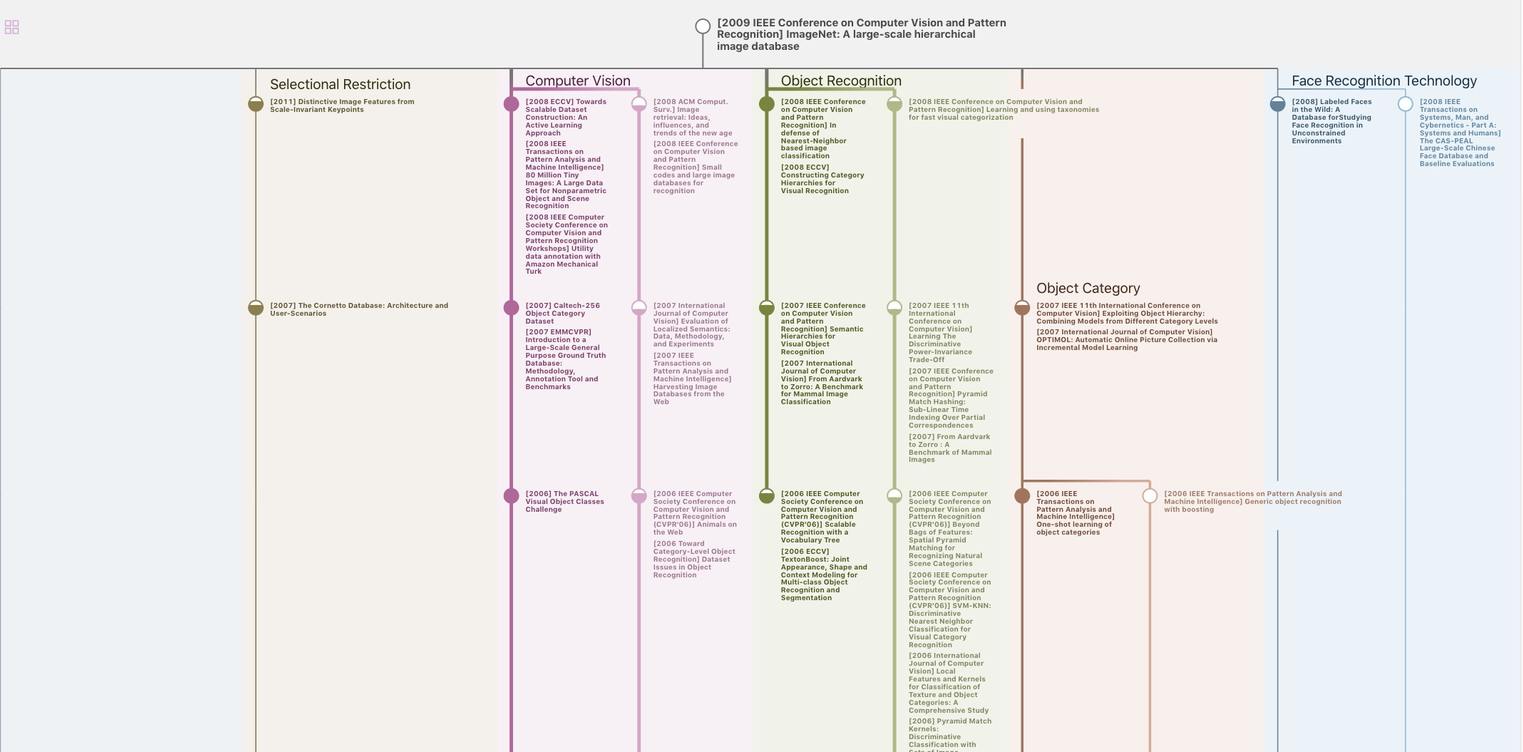
生成溯源树,研究论文发展脉络
Chat Paper
正在生成论文摘要