Reinforcement Learning for Distantly Supervised Relation Extraction
IEEE Access(2019)
摘要
Relation extraction is a task of identifying semantic relations between entity pairs from plain text, which can benefit a lot of AI applications such as knowledge base construction and answer questioning. The distant supervision strategy is introduced to automatically create large-scale training data, which inevitably suffers from noisy label problem. Recent works handle the sentence-level denoising by reinforcement learning, which regards the labels from distant supervision as the ground-truth. However, few works focus on the label-level denoising that corrects noisy labels directly. In this paper, we propose a reinforcement learning-based label denoising method for distantly supervised relation extraction. The model consists of two modules: extraction network (ENet) and policy network (PNet). The core of our label denoising is designing a policy in the PNet to obtain latent labels, where we can select the actions of using the distantly supervised labels or the predicted labels from the ENet. More concretely, the task can be modeled as an iterative process. First, the ENet predicts the relation probability, through which the model generates state representation. Second, the PNet learns the latent labels with taken actions and uses them to update the ENet. Then the optimized ENet gives the rewards to the PNet. The joint learning of two modules can obtain a reliable latent label and effectively improve the classification performance. The experimental results show that reinforcement learning is effective for noisy label correction and the proposed method can outperform the state-of-the-art relation extraction systems.
更多查看译文
关键词
Relation extraction,distant supervision,reinforcement learning,noisy label
AI 理解论文
溯源树
样例
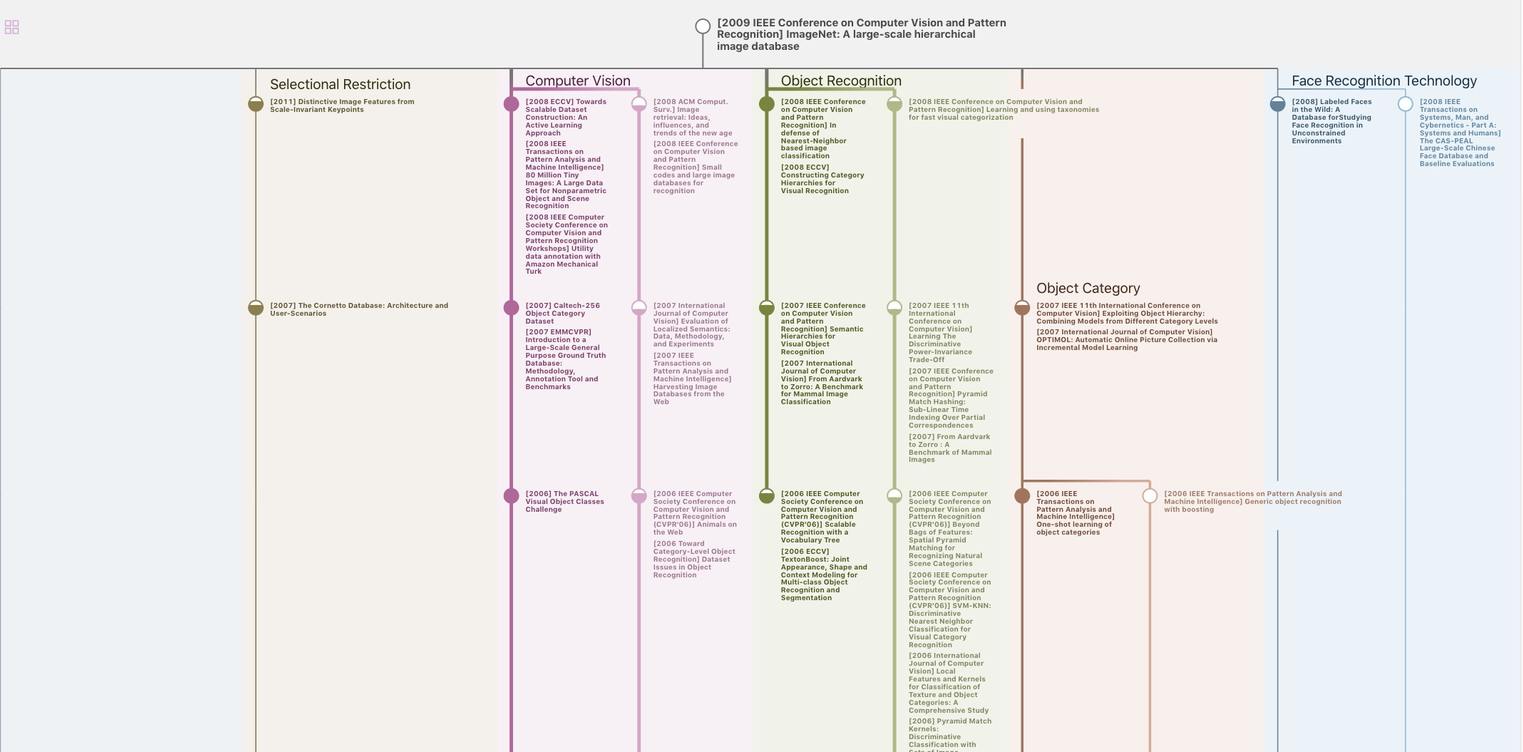
生成溯源树,研究论文发展脉络
Chat Paper
正在生成论文摘要