Automatic Welding Defect Detection of X-ray Images by Using Cascade AdaBoost with Penalty Term
IEEE Access(2019)
摘要
Welding defect constitute a great danger to the safe usage of petroleum pipelines. In addition, manual welding defect detection has numerous deficiencies, such as subjectivity and inaccurate estimation of geometric parameters. Thus, we proposed an automatic welding defect detection system for X-ray images. In the system, five typical defects (cracks, lack of penetration, lack of fusion, round defects, and stripy defects) and non-defects were chosen for recognition. There are three stages in the system: defect extraction, defect detection, and defect recognition. In the first stage, background subtraction with an adaptive thresholding method was adopted to identify the potential defects. In the second stage, to extract real defects from the massive number of potential defects, the adaptive cascade boosting (AdaBoost) algorithm was employed for binary classification. Grayscale features and geometric properties of the defects were extracted for the classification. In the third stage, the AdaBoost algorithm was extended for multi-classification. In the process of distinguishing defects from non-defects, a high detection rate is necessary. To ensure the high true positive rate (TPR) and the low false positive rate (FPR), we proposed the cascade AdaBoost algorithm with penalty term. The accuracy of the defect detection was 85.5%, and the TPR was 91.66%. Moreover, three comparison tests of support vector machine (SVM), k-nearest neighbor (KNN), and random forest (RF) were employed to validate the superiority of AdaBoost. The experimental results indicate that the proposed detection system can be effective for defect detection.
更多查看译文
关键词
AdaBoost,image processing,X-ray image,nondestructive testing,welding defects
AI 理解论文
溯源树
样例
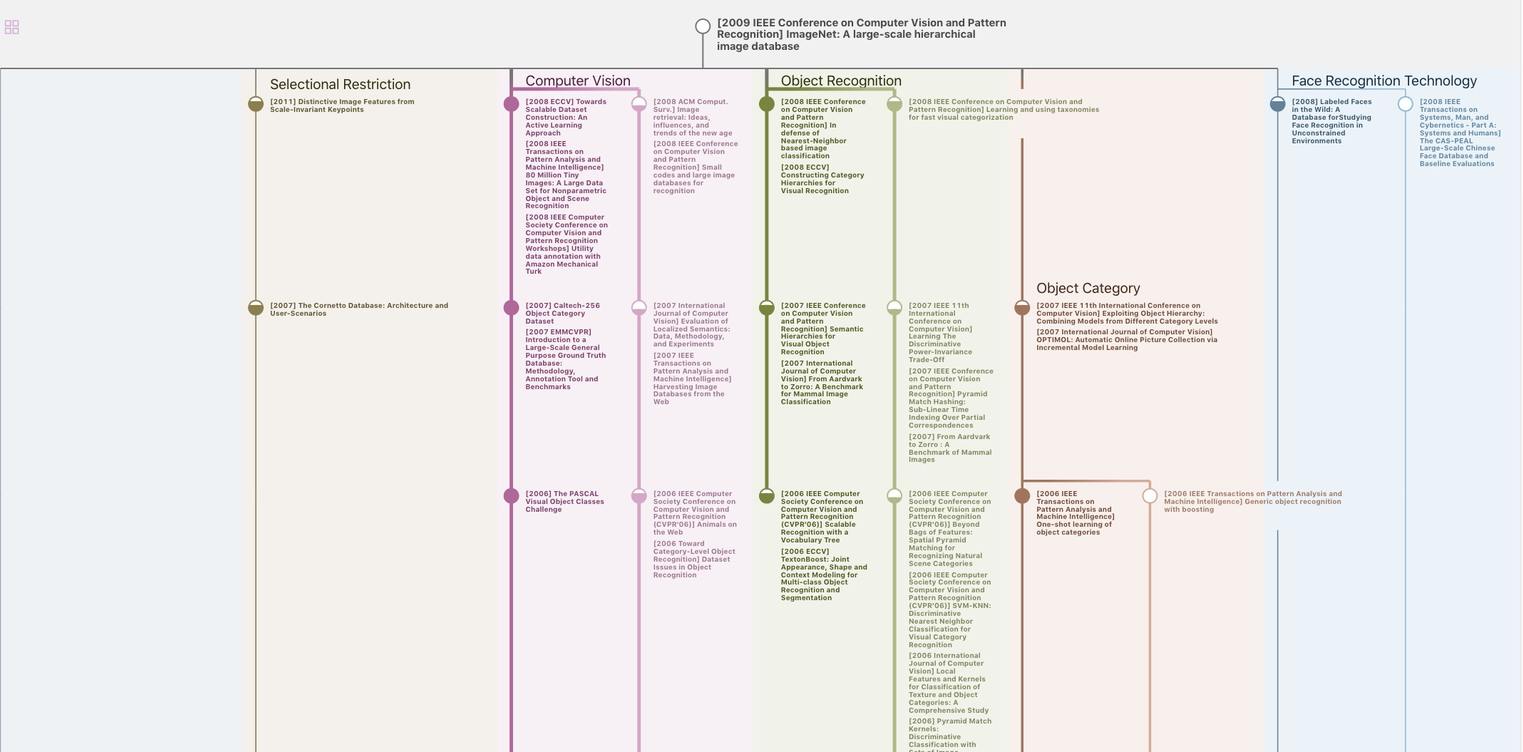
生成溯源树,研究论文发展脉络
Chat Paper
正在生成论文摘要