Sequence- and structure-based prediction of amyloidogenic regions in proteins
Soft Computing(2019)
摘要
Machine learning methods are increasingly used in proteomics research, especially in analyzing and predicting protein structures, functions, subcellular localizations and interactions. However, much research in recent years has focused on protein misfolding problem and the impact of unfolded and defective proteins on cell dysfunction, due to its considerable importance for molecular medicine. These abnormal proteins degradation and deposition often result in the formation of certain plaque cores among them the so-called amyloid fibrils which are responsible for an increasing number of highly debilitating disorders in humans. Yet, a significant challenge remains, especially in understanding the underlying causes and major risk factors of these harmful deposits in vital organs and tissues. This paper explores the potential of string kernel-based support vector machines in the prediction of amyloidogenic regions in proteins by incorporating the most informative features of the protein sequence such as predicted secondary structure and solvent accessibility, with a special focus on α -helical conformations which seem to be primarily concerned with amyloidogenesis. The performances compared with the most popular methods on Pep424 and Reg33 benchmark datasets indicate the robustness of the predictive model. Furthermore, the results showed accurate prediction of regions promoting fibrillogenesis for experimentally determined amyloid proteins and revealed that the five amino acids Leucine, Glycine, Alanine, Valine and Serine are predominantly present in amyloid-prone regions and confirm that the core regions of an amyloid aggregate are not necessarily fully buried.
更多查看译文
关键词
Protein misfolding, Amyloid aggregation, Secondary structure, Solvent accessibility, Support vector machine, String kernels
AI 理解论文
溯源树
样例
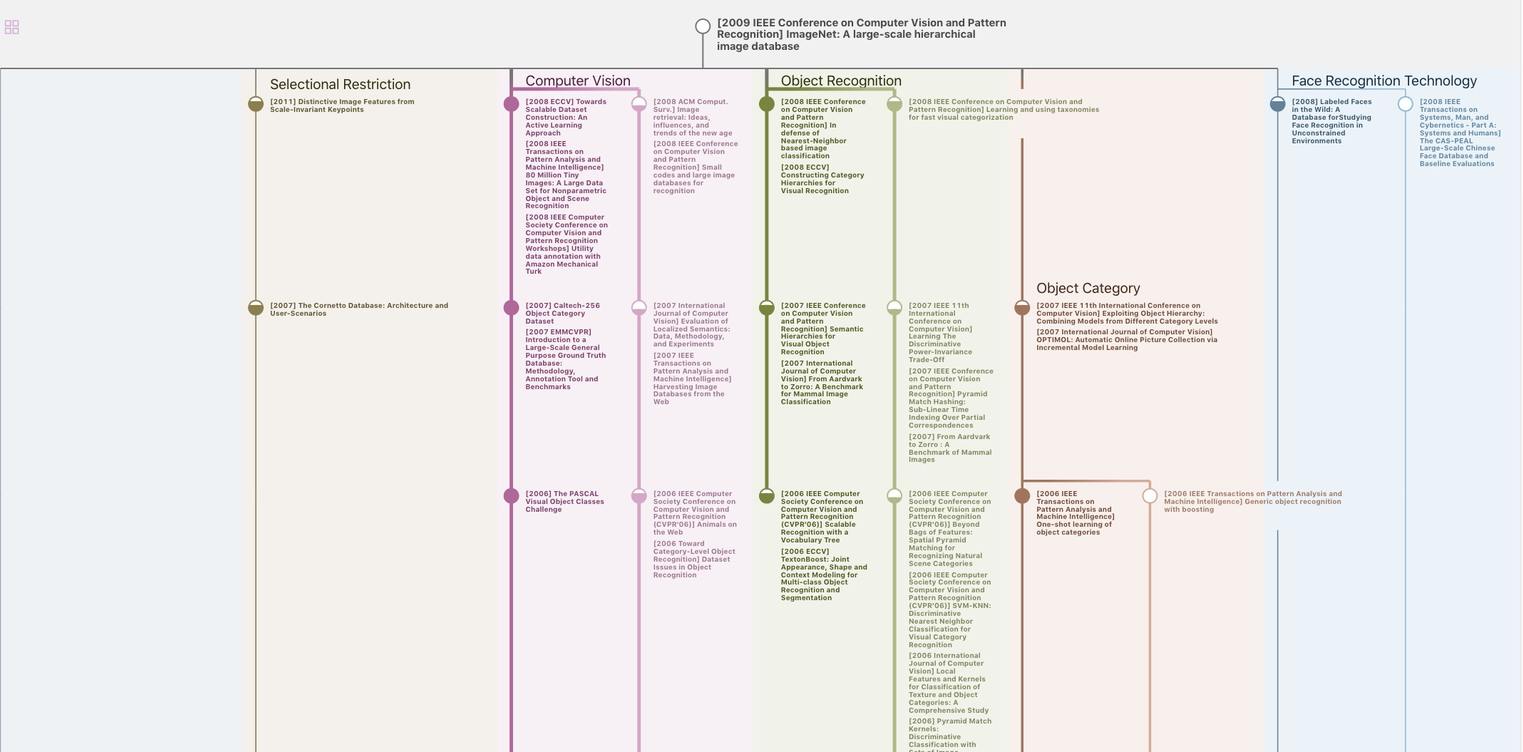
生成溯源树,研究论文发展脉络
Chat Paper
正在生成论文摘要