Channel Attention Networks for Image Translation
IEEE Access(2019)
摘要
Existing image-to-image translation methods usually adopt an encoder-decoder structure to generate images. The encoder extracts the features of input images using a sequence of convolution layers until a bottleneck, and then, the intermediate features are decoded to the target image. However, the existence of bottleneck layer in such structure may lead to blurry and bad quality of the translated images, since different domain translations may be related to the global or local region in the input image or even in an abstract level. To prevent these problems, we propose the channel attention networks for image translation in this paper. It is a novel model that supports the multi-domain image-to-image translation using one single model. Conditioning on the target domain label, an auto-encoder-like network with multiple attention connections is trained to translate the input image into the target domain. The attention connections better shuttle the low-level information in the encoder to the decoder, which helps to preserve the structure. A multi-level attention mechanism is also designed in the proposed model to further improve the performance of our model. More specially, the feature maps in the encoder are first squeezed by average pooling and used to output a channel-wise attention mask. The attention mask softly determines which channels of the feature maps are translated and which channels are kept. By enforcing the model to learn a cyclic domain transformation during training, our model does not require paired training data, which greatly improves the versatility to different kinds of data. We experimentally demonstrated the effectiveness of our proposed model on the facial and clothing image translation tasks. The extensive ablations are also conducted to further validate the contribution of the proposed attention module used in our model.
更多查看译文
关键词
Deep learning,computer vision,image generation,generative adversarial networks,image translation
AI 理解论文
溯源树
样例
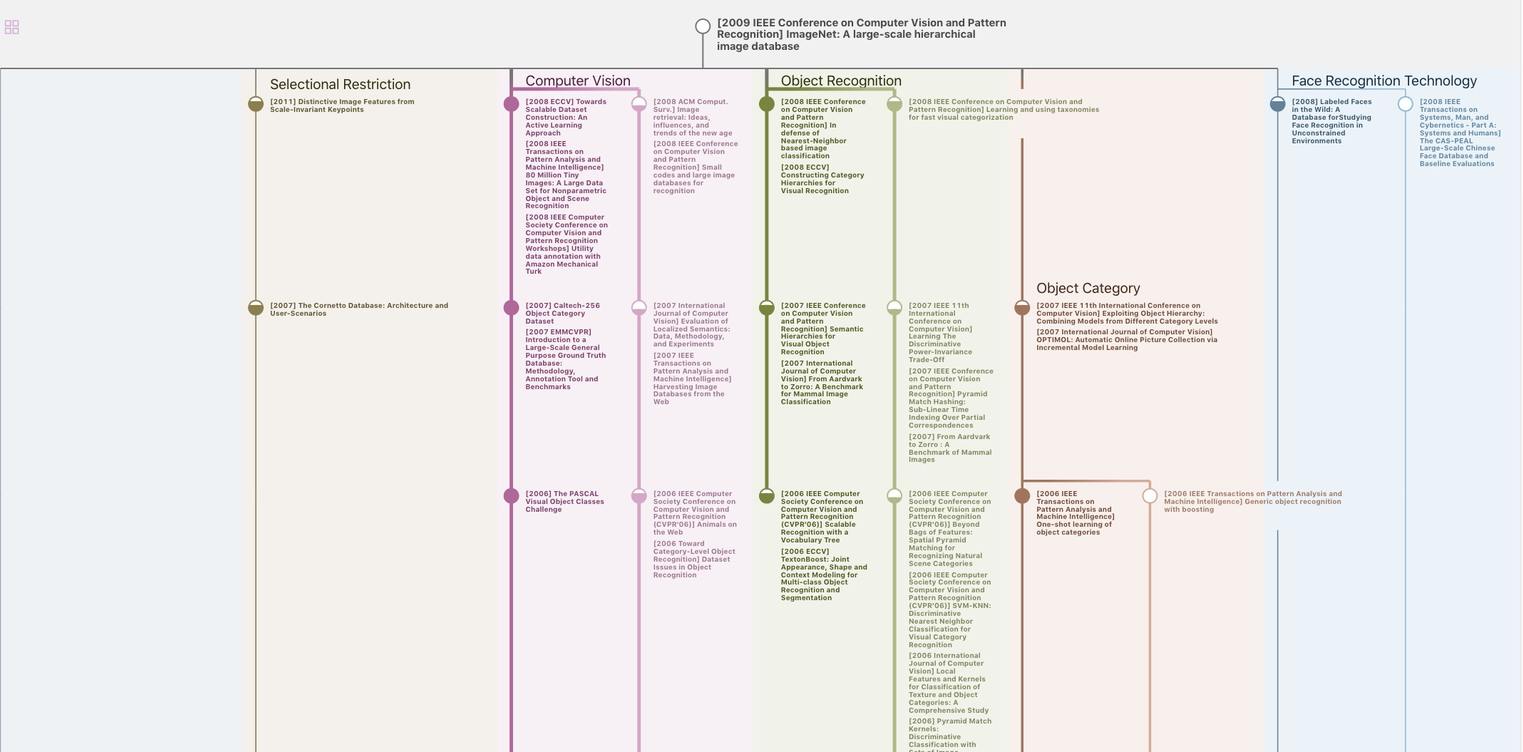
生成溯源树,研究论文发展脉络
Chat Paper
正在生成论文摘要